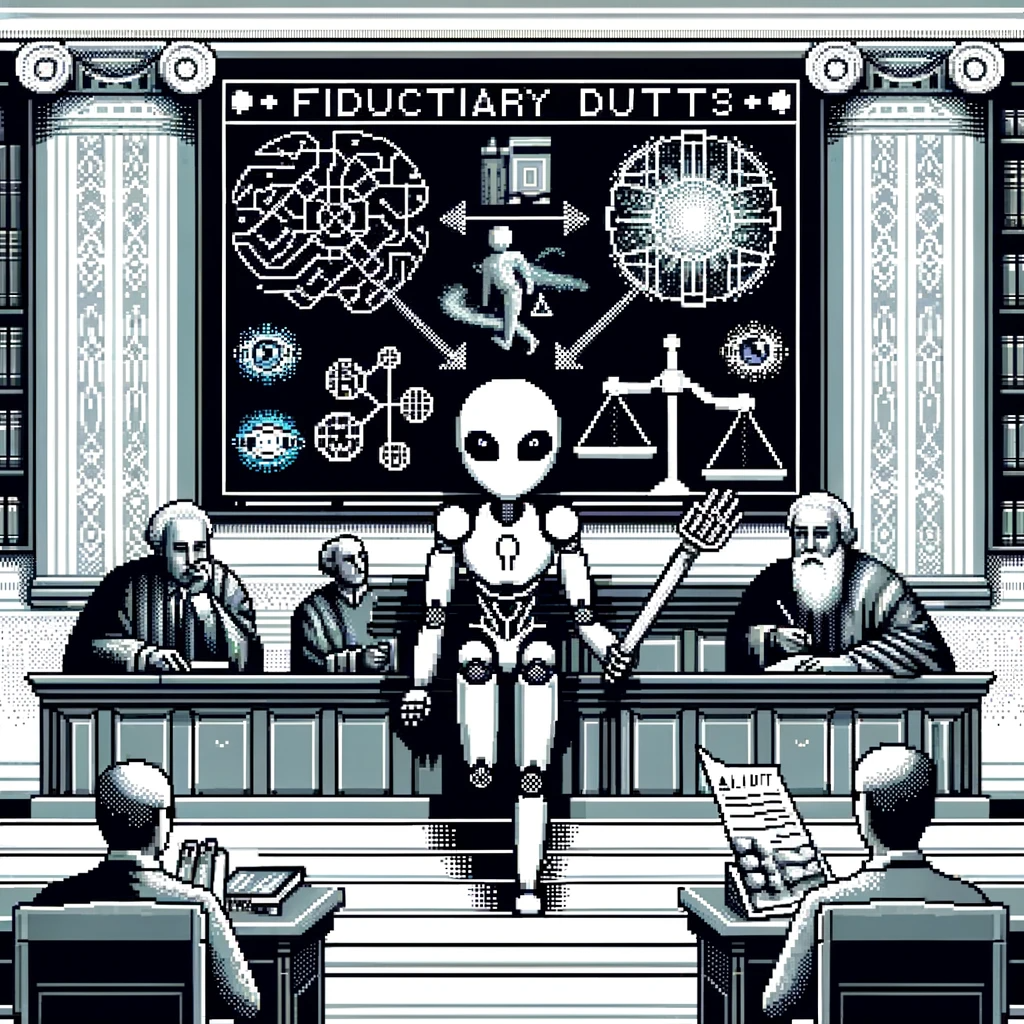
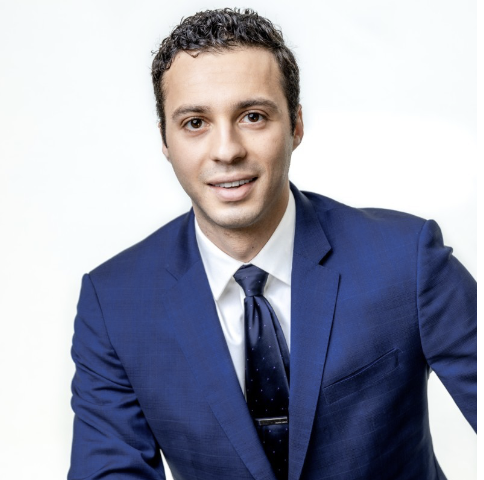
🔬 Research Summary by David Shekman , a third-year law student at Northwestern University Pritzker School of Law, will be practicing in San Francisco upon graduation, and is an avid data scientist.
[Original paper by Sebastian Benthall and David Shekman]
Overview: A fiduciary is a trusted agent with the legal duty to act with loyalty and care toward a principal who employs them. This paper discusses fiduciary duties as a path forward for AI alignment and presents a method for designing AI systems to comply with fiduciary duties. A Fiduciary AI designer should understand the system’s context, identify its principals, assess the best interests of those principals, and then be loyal to those interests.
Introduction
Not long after each wave of AI innovation arrives, so do renewed concerns about whether these tools align with the ethics and goals of society and how to ensure that they do. AI alignment research in legal and technical communities has been developing in parallel but siloed. We propose a deeper understanding of a Fiduciary AI system by evaluating existing fiduciary duties and proposals for expansion and leaning on reward modeling paradigms. We hope this work can help bridge AI alignment discussions in the technical and legal communities.
To this end, we have outlined the legal rationale for fiduciary duties, particularly their application to computational systems and AI. We have then provided a guide for how a Fiduciary AI system can be designed or audited for compliance with these rules.
Key Insights
Understanding Fiduciary Duties
Fiduciary duties are some of humanity’s oldest rules, dating back to Hammurabi’s Code, and are legally recognized throughout the world today. Named for fiducia, the Latin word for trust, they concern the duties of an agent hired to perform a task for a principal. Fiduciary duties arose from a particular combination of factors — a recognition that specialization is an important factor in society, that specialization inherently requires reliance by beneficiaries on the expertise of those specialists, and that certain types of specialist-client relationships create a greater level of reliance and greater potential for exploitation or abuse and thus deserved an extra level of protection. Fiduciary duties were put in place to ensure that clients of these specialists were protected from exploitation (duty of loyalty) and were ensured the quality of specialist service was up to a minimum standard (duty of care). Over time, additional rules and structures around fiduciaries were developed to resolve conflicts and clarify vague circumstances.
Implementing Fiduciary Duties for AI
We see many similarities in the core issues facing AI alignment and the initial power dynamics that led to the modern structure of fiduciary duties. Our approach borrows from this legal construct the underlying concepts and their mechanisms that have developed over time while adapting them into a technical model that can be implemented in the design of AI. We developed a six-step structure for AI designers to follow that addresses the primary rules and structure of fiduciaries — (1) context, (2) identification, (3) assessment, (4) aggregation, (5) loyalty, and (6) care.
Context
In the law, no fiduciaries are absolute, required under all circumstances to serve the interests of a principal. Rather, individuals are fiduciaries to others due to their respective roles in a legally recognized context. A first design principle for Fiduciary AI is that the context within which the AI is acting as a fiduciary must be established and understood.
Identification
Designing Fiduciary AI requires an explicit understanding of a system’s principals. In most cases today, AI is designed mainly to benefit those who deploy the system, using mechanism design techniques to steer the behavior of users toward those goals. Fiduciary AI can be designed with other categories of principals in mind, and identifying these principals is essential for compliance with fiduciary duties. This choice is not purely technical but inferred from the previously identified legal or social context.
Assessment
Once the principals of a fiduciary AI system are identified, the designer should identify the principal’s best interests in the scope of the fiduciary context. One approach to assessing these best interests is to learn an objective function from data provided by the population of principals, like behavior observations, statements of preferences, or direct measurements of user well-being. Yet even these approaches are not straightforward, as temporal discounting (diminished value of delayed reward) and the time inconsistency of preferences present complications in understanding the best interests of a principal. Legal rules can also provide context-specific understandings of “best interest” that may be used to guide the system design.
Aggregation
One conceptual challenge that computational fiduciaries raise is how a system can be loyal to the interests of multiple, potentially conflicting principals. The field of social choice and voting theory has shown that it is impossible to design a mechanism that aggregates preferences while meeting several theoretically desirable criteria. However, many of these technical problems are alleviated when the preferences can be expressed and aggregated into partially ordered functions. The problem of conflicting principal interests has also arisen for non-computational fiduciaries and, in some cases, settled by further legal rules. These tend to be context-specific subsidiary duties.
Loyalty
Loyalty is one of the two pillars of fiduciary duty. Given the incompleteness of instructions to an AI, loyalty is optimized around the principal’s best interests. The system must put the principal’s best interests (limited by the above scope) ahead of anyone else’s. This loyalty has a general duty of alignment and contextually specific subsidiary rules with clearer requirements. The optimization of a proxy objective function can characterize the general duty of loyalty. Subsidiary duties of loyalty can provide firmer constraints on system behavior and address problems that are difficult to solve using machine learning.
Care
The duty of care sets a minimum standard for the quality of the agent’s representation, holding the agent liable for foreseeable harm, even without intent. Care requires the agent to be informed before making decisions and not to make them recklessly, and the standard of prudence is defined contextually. Some aspects of AI design are especially prone to negligence. Whereas the choice of training data and benchmarking of a model are often deliberate choices, the inductive bias of a machine learning system can be an afterthought, determined by default. This makes inductive bias a good candidate for being addressed by the duty of care.
Between the Lines
The problems that arise in AI alignment are more similar to prior non-technical problems society has faced than they may initially seem. Much like the development of fiduciary law itself, this technical approach will continue to grow and adjust as it is implemented. Future work applying this model and identifying its boundaries, conflicts, and vague areas will help refine and clarify the model and develop the additional rules and mechanisms that bring it closer to the end goal of AI alignment. Also remaining as open questions are the usefulness of human-centered and participatory design methods and how this conception of care compares with other notions of care in AI and digital infrastructure.