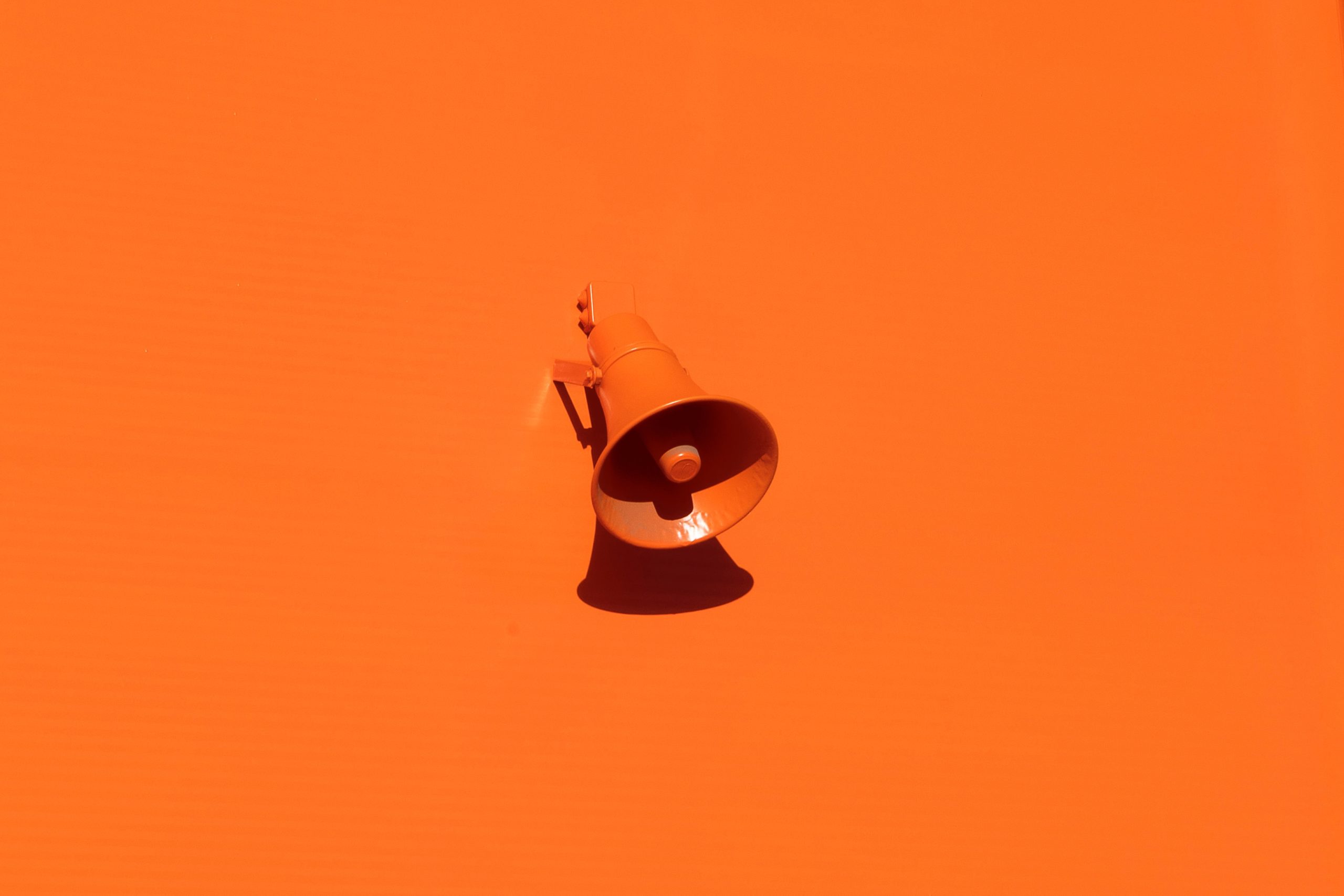
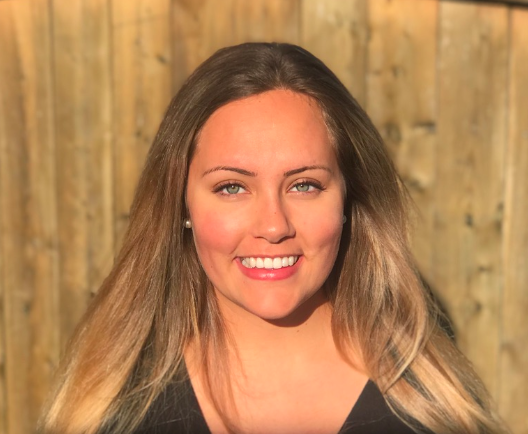
🔬 Research Summary by Grace Wright, Business Development Manager at a technology start-up and has worked in research roles focused on responsible and ethical development and use of AI and other emerging technologies.
[Original paper by Christian Meske, Enrico Bunde]
Overview: With the rise of “hate speech” on social media platforms, the demand for content moderation has been growing and as a result, companies have been dedicating further resources to developing AI systems that can support hate speech detection and moderation at scale. This paper explores principles that can be used to inform the design of these AI systems, with a specific focus on the experiences of the human content moderators that use them to support their work.Â
Introduction
Hate speech on social media has become a controversial topic in today’s world, sparking debates about free expression, deplatforming, and the policies that guide content moderation in an ever changing world.
Increasingly, artificial intelligence (AI) is being used to support human content moderators with detecting and reviewing potential hate speech content shared on social media platforms. The research discussed in this paper focuses on design principles that can be used to build these AI systems (referred to as decision support systems), and more specifically, principles that incorporate the perspectives and user experiences of the human content moderators that use them.
To explore this challenge in greater depth, the researchers sought to answer to major questions: 1) what key design principles can be used when creating explainable AI (XAI)-based user interfaces (UIs) to support content moderators, and 2) how are those UIs perceived by key stakeholders and how influential are they in helping moderators understand the reasons behind a particular outcome the AI systems produce? Through conducting multiple design cycles, the researchers explored these questions in greater depth and developed four key replicable design principles to help guide future development of these AI systems.
Key Insights
The Problem
Social media is a tool that can be used to connect people across the world, exchange ideas and perspectives, and generate positive social change. However, like any other tool, it comes with its risks and concerns over its potentially harmful impact. Increasingly, the rise of hate speech on social media platforms have been at the centre of these concerns, raising challenges for social media companies trying to moderate this content at a global scale.
In response to these concerns, social media companies have made efforts to introduce policies to address hate speech and limit its potential psychological harm on users. To enforce these policies, these companies often use human moderators to review content that may violate content policies and community codes of conduct. However, moderation at scale is incredibly challenging given the sheer volume of content shared on a daily basis, not to mention the social, cultural, and linguistic nuances that impact moderation as well.
The Role of AI
To address this challenge, companies are deploying artificial intelligence-based systems to help moderators with hate speech detection and decision making. While these decision support systems (DSSs) can be helpful, they often present the classic black box challenge – which simply refers to the lack of explainability/transparency in how an AI system generates an outcome. As the researchers note, to mediate this issue, significant amounts of attention, research, and investment has been channelled to create more explainable AI (XAI).
However, as the authors of this paper argue, despite this research, many metrics for assessing DSSs and their explainability do not focus enough on the experience of the human moderators that use them, but instead are evaluated primarily from a technical perspective. To address this deficit, the authors conducted research to develop a design framework that involves the end users in evaluating the user interfaces of DSSs.
The Design PrinciplesÂ
To accomplish this goal, the researchers conducted three design cycles to develop a reproducible framework for evaluating key design principles that can be used when designing XAI user interfaces (UIs) for content moderators. The four design principles that were evaluated through the research included:
- Creating systems with transfer learning techniques to improve decision support (i.e., a computer learning to apply knowledge from one problem to another to help shape its decision)
- Developing systems with features that provide explanations of decisions to help users understand the rationale behind certain outcomes
- Designing systems with the capacity to present relevant context to various use cases so that users have a better understanding of the case they are examining
- Providing systems with the ability to let users take case-based actions that incorporate their own social and cultural knowledge in order to make fairer decisions
The Research Process
To evaluate the efficacy and utility of the design principles in focus, the researchers conducted three design cycles. The first cycle involved a literature review to assess how existing research integrated the role of human content moderators in the detection of AI-based hate speech, followed by the development of general requirements for AI-based decision support systems. They also implemented a transfer learning model to generate predictions and explanations of specific outcomes for the user interface. The design was then assessed by research participants with experience in social media content moderation and the feedback was integrated into the second design cycle.
The second design cycle was focused on refinement, including UI evaluation by nearly 200 participants who assessed its perceived usefulness, ease of use, intention to use, and overall utility. The feedback from this cycle was then integrated into the third and final design cycle in the research, which involved examining different strategies for evaluating design principles. After refining the model further, the researchers implemented the UI and had it assessed by a larger pool of participants to help inform how users perceived the overall quality of the UI and the usefulness of it to practitioners designing these systems.
Research ImpactÂ
The research found that the majority of practitioners found the UI useful, which indicates the promising replicability of these principles in other DSSs. The results of this research also demonstrated that providing an explanation of specific DSS outcomes can help achieve goals related to developing more explainable AI models, including as it relates to trustworthiness and informativeness. Finally, the research also showed that human content moderators are important to involve in the evaluation of DSSs, since they offer unique backgrounds, expertise, informational needs, and expectations that impact content moderation decisions.
Between the lines
The research described in this paper has important implications for the development of future DSSs used for content moderation. Firstly, it offers practical principles for developing these systems that can guide future practice and development. Moreover, it emphasises the importance of incorporating the perspectives and experiences of human content moderators in these systems since they are the most familiar with content moderation processes.
While the research highlights the importance of human content moderator perspectives, this takeaway does prompt additional questions for research. For example, given the variability of human perspectives, including psychological, political and sociocultural influence, does the incorporation of human content moderator perspectives ultimately increase the fairness of these decisions? How might those decisions change based on current political event or changing leadership at either a national and corporate level? These concerns and others like them will undoubtedly breed new challenges for social media companies and governments alike as they determine how to effectively manage this complex, nascent issue.