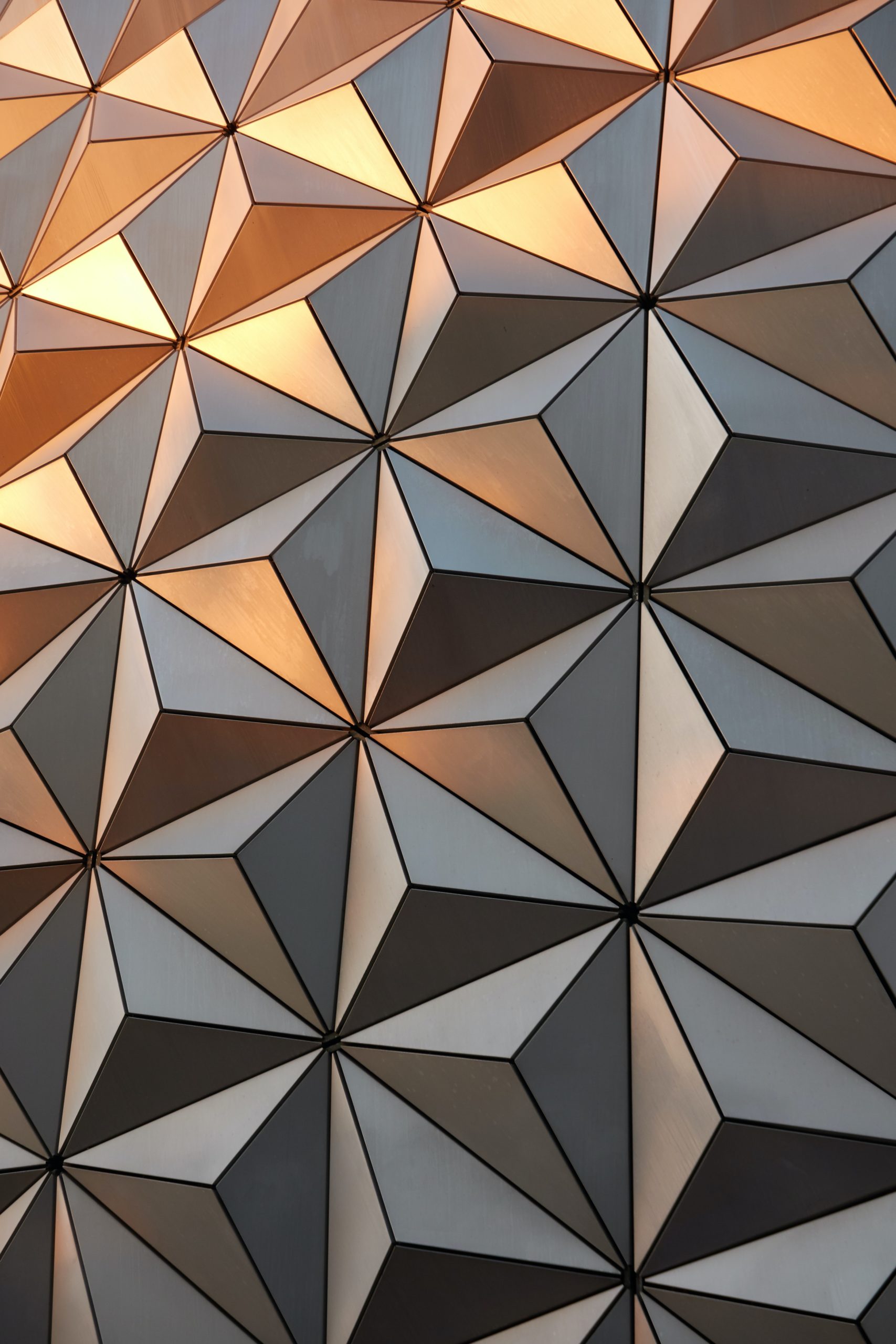
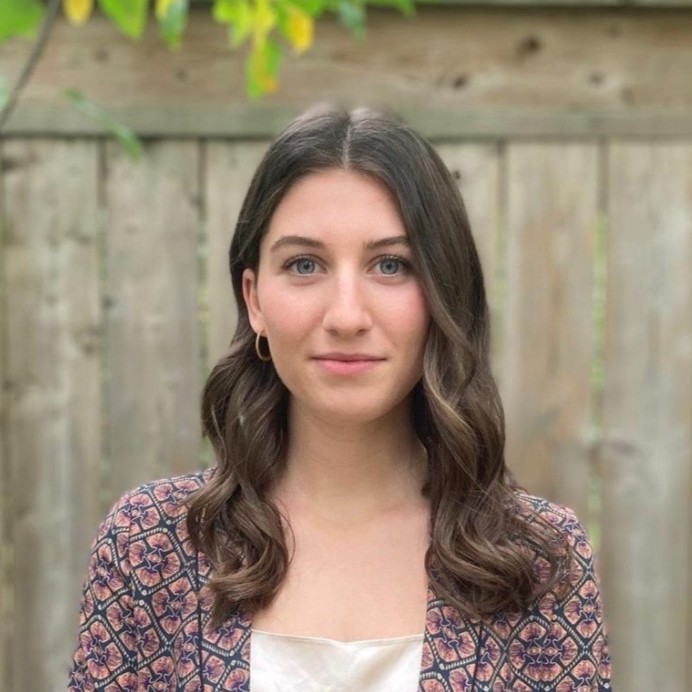
🔬 Research Summary by Sarah Villeneuve, a Program Lead working on Fairness, Transparency, and Accountability at the Partnership on AI.
[Original paper by McKane Andrus and Sarah Villeneuve]
Overview: Most current algorithmic fairness techniques require access to demographic data (such as race, gender, or sexuality) in order to make performance comparisons and standardizations across groups. These demographic-based algorithmic fairness techniques look to overcome discrimination and social inequality with novel metrics operationalizing notions of fairness and by collecting the requisite data, often removing broader questions of governance and politics from the equation. In this paper, we argue that collecting more data in support of fairness is not always the answer and can actually exacerbate or introduce harm for marginalized individuals and groups, and discusses two paths forward that can mitigate the risks discussed.
Introduction
Algorithmic decision making systems carry the risk of systematic, albeit usually unintentional, discrimination or unfairness. A number of high profile examples of discrimination in algorithmic systems, such as racial bias in mortgage approval algorithms or sexist hiring tools, have sparked calls for the adoption of algorithmic fairness techniques to combat the harmful social biases that emerge from, or get reinforced, through algorithmic systems.
Many algorithmic fairness techniques require access to data on a sensitive attribute or protected category of an individual in order to make comparisons and standardizations across groups. However, in many domains, demographic data remains either inaccessible or unreliably collectable, due to a number of legal and organizational barriers to collecting sensitive, demographic data. Without a clear understanding of how practitioners can responsibly collect this data, they remain unable to adequately identify and assess discrimination in their systems.
Before answering the question of “how” demographic data should be collected responsibly to support fairness interventions, we needed to first consider the more normative question of if or when demographic data should be collected.
In our paper, “Demographic Reliant Algorithmic Fairness: Characterizing the Risks of Demographic Data Collection and Use in the Pursuit of Fairness,” we challenge the notion that algorithmic discrimination can be overcome with smart enough technical methods and sufficient data alone. Specifically, we explore under what conditions demographic data, such as gender, race, sexuality, and age, should be collected and used to enable algorithmic fairness methods by investigating a range of social risks to individuals and communities.
Key Insights
Demographic Data and Concerns Around Measurement
We use this term to refer to categorizations about individuals based on observable or self-identifiable characteristics such as skin tone, hair length, and vocal range. These characteristics are used to inform demographic categories, which attempt to collapse complex socio-political concepts into categorical variables.
This project of categorization can oftentimes be misaligned with the needs, desires, and experiences of the people being categorized, meaning that it is entirely possible for “fairness” efforts to be misaligned from the start. Our paper delves into what these misalignments might look like and the types of risk they entail.
Risks of Demographic Data Use and Collection
The risks we discuss in our paper fall into two categories: Risks to Individuals and Risks to Communities. The risks we identified through our research are listed below:
Individual Risks
Privacy: Many categorizations that might be salient axes of algorithmic discrimination are also categorizations that governments and policing institutions are likely to target discriminatory or oppressive practices towards. Attributes such as documentation status, political affiliation, and sexuality cannot be shared without entailing some type of risk to the individual. Similarly, collecting demographic information also carries the risk of exposing data subjects to bigotry and direct discrimination in the case of unvetted data sharing and data leaks.
Individual Miscategorization and Identity Misrepresentation: This risk can arise when these systems fail to accurately represent an individual’s identity. Individual miscategorization can occur when an individual is misclassified despite there being a representative category that they could have been classified under. Identity misrepresentation on the other hand, can occur when categories used do not adequately represent the individual as they self-identify. Miscategorization and identity misrepresentation may not only lead to social and political discrimination, but also psychological and emotional harms via feelings of invalidation and rejection.
Data Misuse: The final individual risk we consider is Data Misuse. Corporations collecting and using individuals’ demographic data to train and deploy algorithmic decision making systems are facing increased pressure (from both the public and regulatory bodies) for transparency on how such data is collected and used. Data Misuse can refer to either the use of data for a purpose other than that for which it was collected or consent was obtained or instances where data is shared with third parties or packaged and sold to other organizations.
Risks to Communities
Expanded Surveillance: The assumption that more data will result in fairer algorithmic systems neglects to consider the risk of expanded surveillance and questions around who benefits from increased data collection. Scholars of surveillance and privacy have shown time and time again that the most disenfranchised and “at-risk” communities are routinely made “hypervisible” by being subjected to invasive, cumbersome, and experimental data collection methods, often under the rationale of improving services and resource allocation. Increased visibility and awareness of being under surveillance is likely to have a chilling effect on already disenfranchised groups and society at large.
Misrepresentation and Reinforcing Oppressive Categories: At a high level, these risks center around essentializing or naturalizing schemas of categorization, categorizing without flexibility over space and time, and misrepresenting reality by treating demographic categories as isolated variables instead of structural, institutional, and relational phenomenon. Misrepresentation occurs when entire groups are forced into boxes that do not align with or represent their identity and lived experience. Even in cases where groups feel adequately represented by a categorization schema, there is a risk of reinforcing and naturalizing the distinctions between groups, especially in cases where demographic variables are uncritically adopted as an axis for differential analysis.Private Control of What Constitutes as Fairness: When those collecting data have blindspots about what impacts decision-making and individuals’ life experiences, various forms of discrimination and inequality run the risk of being misread as inherent qualities of groups or cultural differences between them. When an organization is asking already marginalized groups to share information for the purposes of assessing unfairness, it is important that unfairness is defined in concert with the groups in question.
Between the lines
There are several possible paths forward that would enable organizations to overcome the various legal and organizational challenges associated with the collection and use of demographic data. One set of possible paths forward include a range of approaches that aim to prevent organizations from ever directly learning users’ sensitive attributes. Most of these approaches prioritize anonymizing datasets that include demographics by enforcing standards such as k-anonymity, p-sensitivity, and/or differential privacy.
A second set of paths forward include participatory data governance models. These approaches involve data subjects more directly in determining what data is collected and towards what ends, such as data cooperatives and data trusts, can help mitigate most of the previously discussed risks to individuals and communities.
More research is needed to understand the feasibility of these approaches in practice. The Partnership on AI is currently working on piloting select approaches with Partners. If you’re interested in working with us to assess system fairness, please reach out to Sarah Villeneuve ([email protected]).