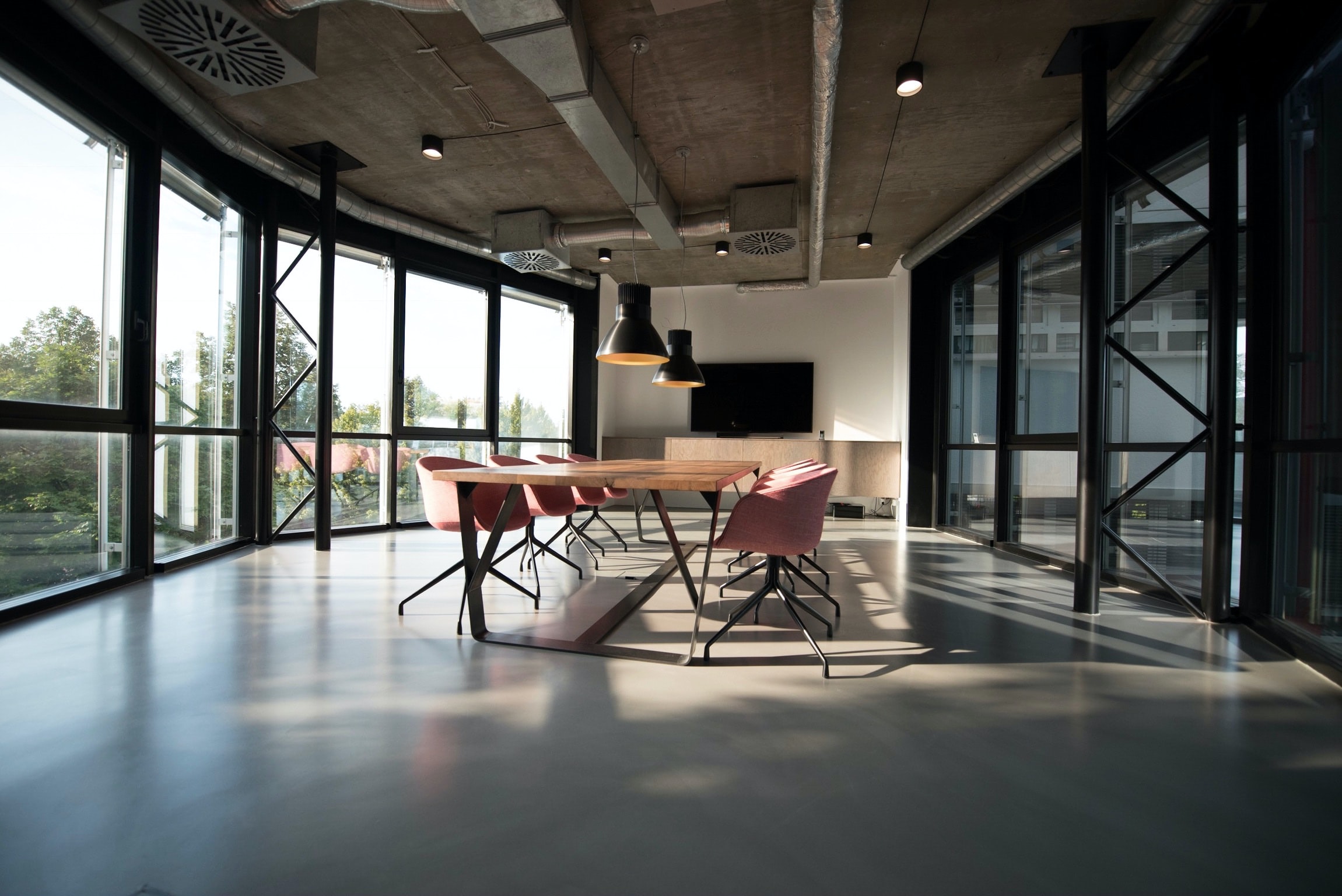
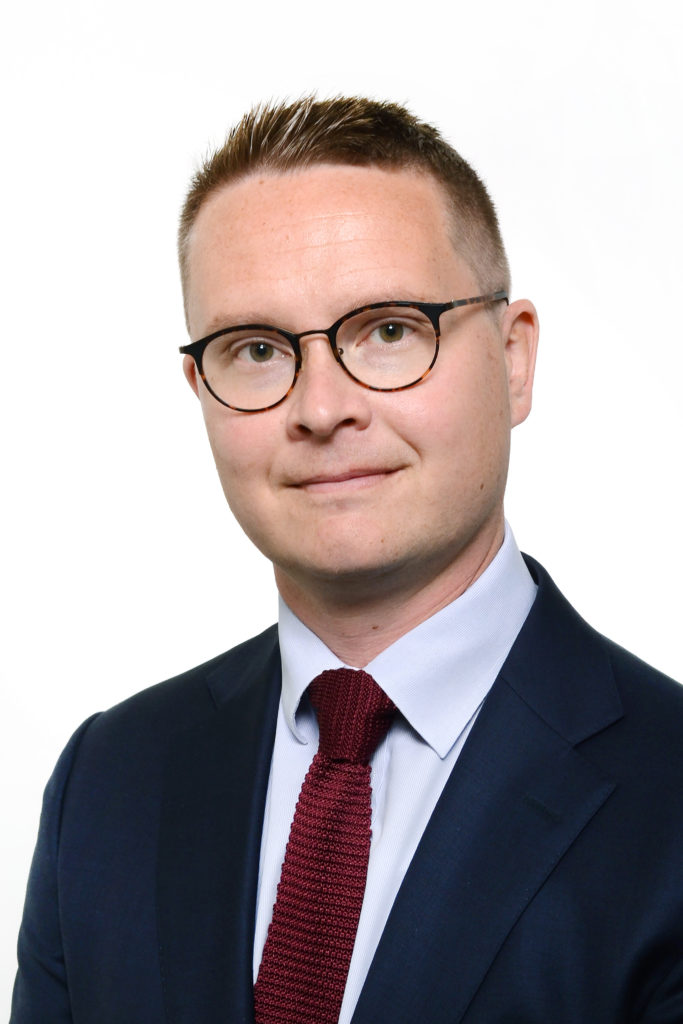
đŹ Research Summary by Matti Mäntymäki, an Associate Professor (Information Systems Science) and PI of the Digital Economy and Society Research Group at the University of Turku.
[Original paper by Matti Mäntymäki, Matti Minkkinen, Teemu Birkstedt, Mika Viljanen]
Overview:Â Organizations can translate ethical AI principles into practice through AI governance. In this paper, we wish to facilitate the adoption of AI governance tools by defining and positioning organizational AI governance.Â
Introduction
Corporate governance, IT governance, data governanceâŚis AI governance just another tick-box exercise for organizations? We donât think so. Even for organizations with well-defined governance structures, AI systems introduce a list of unique considerations. We believe AI governance is a useful concept for all organizations looking to reap the benefits of AI while mitigating the risks. However, it is not always clear how the overall governance fabric unfolds: what does organizational AI governance mean and what differentiates it from the other elements such as IT governance and data governance? Drawing from the previous research, this paper lays the terminological and conceptual foundations that the future work on AI governance can build upon.
Key Insights
AI governance offers a systematic, traceable approach for developing, deploying and monitoring trustworthy AI systems. With Europe set to become the global leader in responsible AI, the need for governing AI systems is evident. From organizationsâ point of view, the challenge of implementing AI governance has been the ambiguous nature of an emerging field. As Butcher and Beridze (2019) put it: âAI governance is an unorganized area.â By providing a clear definition for organizational AI governance, we wanted to make the overall concept more practical and approachable. Our definition is as follows:
âAI governance is a system of rules, practices, processes, and technological tools that are employed to ensure an organizationâs use of AI technologies aligns with the organizationâs
strategies, objectives, and values; fulfills legal requirements; and meets principles of ethical AI followed by the organization.â
Our definition is suited for organizations of all sizes, and it covers the technological, ethical and regulatory aspects of AI governance. The definition also incorporates both the internal (organizationâs values and strategy) and external (requirements from the operating environment) drivers for AI governance.
Our definition is based on the four main arguments:
1. AI governance is a system with interlinked processes.
2. The AI governance system is normative, its key elements being rules, practices, processes, and technological tools.
3. Organizational AI governance needs to address the entire AI system life cycle.
4. The use of AI systems is affected by multiple internal and external factors with possibly conflicting requirements.
Whatâs more, we explored the literature to distinguish corporate governance, IT governance, data governance and AI governance, and align their relative scopes. Firstly, we wish to highlight that corporate governance provides the background for all other activities. Within corporate governance, data governance and AI governance are seen as overlapping, yet not convergent subfields of IT governance. As an example of top-down strategic choices, environmental and social sustainability initiatives from the corporate governance level could be linked to the use of AI systems, that is, AI governance. While several good practices are transferable from IT governance to AI governance, the scalability and increased autonomy of AI systems also calls for special safeguards.
With these insights, we wish to support organizations adopting AI governance as part of their overall governance structure. The definitions will make it easier to align AI governance with organizationâs own strategies but also the existing legal and ethical frameworks. Our work serves as a stepping stone towards responsible AI and promotes further developments on critical tools such as AI governance frameworks.
Between the lines
This paper is about the governance of AI, not governance by AI. The latter, however, would be an interesting research topic for the future. Furthermore, the developments in the intersection of AI governance, data governance, and IT governance will be an area we would like to keep an eye on: to what extent will data governance shape AI governance or the other way around, and will these two eventually become one? Most importantly, organizations need practical AI governance frameworks to work their way towards demonstrably legal and ethically sound use of AI systems.