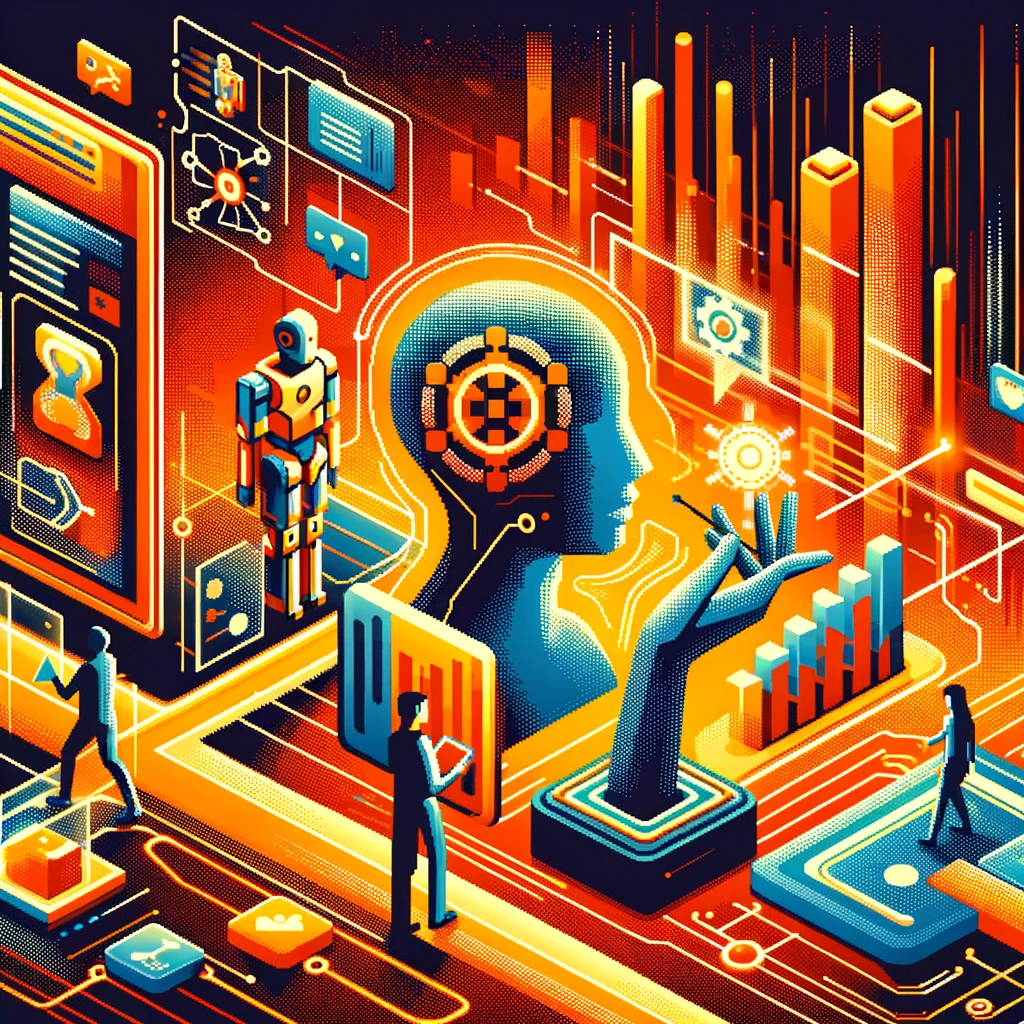
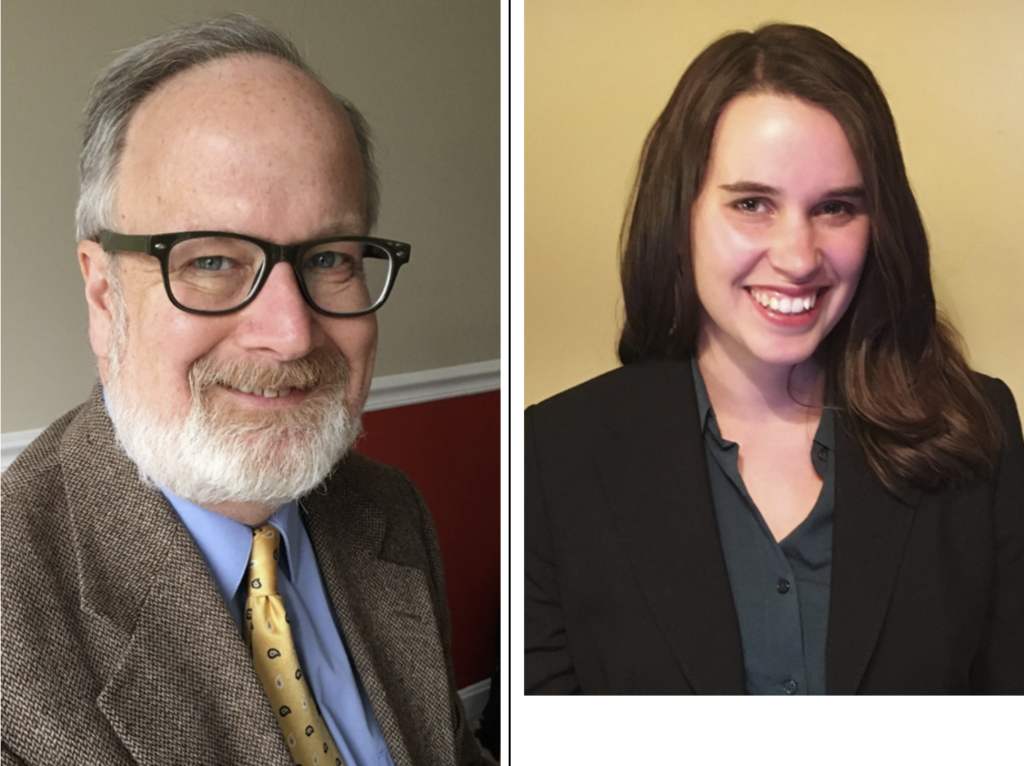
🔬 Research Summary by Dr. James E. McCarthy and Dr. Lillian K.E. Asiala.
Dr. McCarthy is Sonalysts’ Vice President of Instructional Systems and has 30+ years of experience developing adaptive training and performance support systems.
Dr. Asiala is a cognitive scientist and human factors engineer at Sonalysts Inc., with experience in instructional design, human performance measurement, and cognitive research.
[Original papers (see the papers below) by Dr. James E. McCarthy & Lillian K.E. Asiala, LeeAnn Maryeski, & Nyla Warren]
- McCarthy, J.E., Asiala, L.K.E., Maryeski, L., & Warren, N. (2023). Improving the State of the Art for Training Human-AI Teams: Technical Report #1 — Results of Subject-Matter Expert Knowledge Elicitation Survey. arXiv:2309.03211 [cs.HC]
- McCarthy, J.E., Asiala, L.K.E., Maryeski, L., & Warren, N. (2023). Improving the State of the Art for Training Human-AI Teams: Technical Report #2 — Results of Researcher Knowledge Elicitation Survey. arXiv:2309.03212 [cs.HC]
- Asiala, L.K.E., McCarthy, J.E., & Huang, L. (2023). Improving the State of the Art for Training Human-AI Teams: Technical Report #3 — Analysis of Testbed Alternatives. arXiv:2309.03213 [cs.HC]
Overview: There is growing interest in using AI to increase the speed with which individuals and teams can make decisions and take action to deliver desirable outcomes. Much of this work focuses on using AI systems as tools. However, we are exploring whether autonomous agents could eventually work with humans as peers or teammates, not merely tools. We need a robust synthetic task environment that could serve as a testbed to conduct our research. The studies summarized here provide a foundation for developing such a testbed.
Introduction
Have you ever found yourself arguing with your GPS? Have you ever thanked Siri or Alexa? These examples of personification suggest that it is not far-fetched to believe that intelligent agents may soon be seen as teammates. We needed to select or build an appropriate testbed to examine fundamental issues associated with “teaming” with AI-based agents. We established a foundation for this effort by conducting stakeholder surveys and a structured analysis of candidate systems.
Key Insights
1 Step One: Feature Surveys
Given the focus of our work, we decided to develop separate but overlapping surveys for the researchers and the military subject-matter experts. The goal of the surveys was to determine what capabilities stakeholders felt should be included in a testbed.
1.1 Methods
We identified various topics for which stakeholders could provide valuable input and developed a range of open-ended and Likert-style questions from these knowledge elicitation objectives. Respondents completed the survey electronically.
The analysis of the survey results occurred in two threads. First, members of the research team conducted independent thematic analyses of the responses for each open-ended question to identify ideas that recurred across answers, even if the respondents used different wording. After completing the independent analyses, we met and established a consensus list of themes for each question and mapped the individual responses to that consensus list. Second, in parallel with this qualitative analysis of the open-ended questions, we also conducted a quantitative analysis of the various Likert-like items.
1.2 Results
Six themes emerged from the analysis:
- System Architecture
- Teaming
- Task Domain
- Data Collection and Analysis
- Autonomy
- Ease of Use
One theme that strongly emerged when discussing the desired system architecture was the need for operational flexibility within the STE. Respondents wanted to be able to modify the STE over time, and this was expressed in language calling for modularity, open-source development, flexibility, and so forth. Respondents also suggested that we investigate existing STEs that could be used “as-is” or extended to meet particular needs. The flexibility theme continued when respondents mentioned teaming. Team sizes fell between 6 and 12, and they wanted to be able to assign humans and agents to do a variety of roles flexibly. When respondents mentioned the task domain, they emphasized the need for sufficient levels of complexity, fidelity, and interdependence to ensure that the lab results would transfer to the field. Data collection and analysis was another topic that several respondents addressed. They wanted to ensure that the STE was instrumented to collect a wide range of data points they could use to create specific metrics. The last two themes focused on having some form of autonomy within the STE and creating a game-play environment that is easy to learn, including intuitive displays.
2 Step Two: Testbed Analysis
Several respondents recommended that our team look into existing human-AI teaming testbeds rather than creating something new. This was surprising because our initial literature review indicated no “consensus” testbed existed, and each lab developed its own. Nonetheless, we took the recommendation seriously and systematically investigated the associated landscape.
2.1 Methods
The research team began its analysis of potential testbeds by defining a three-dimensional taxonomy. We noted that testbeds could be assessed for the level of interdependency they support, their relevance to the likely application environment, and the sophistication of the agents they could house.
We then used the results of the surveys to identify, define, and weight eight evaluation factors:
- Data Collection & Performance Measurement Factors
- Implementation Factors
- Teaming Factors
- Task Features
- Scenario Authoring Factors
- Data Processing Factors
- System Architecture Factors
- Agent Factors
In parallel with this process, the team conducted a literature review and identified 19 potential testbeds across three categories:
- Testbeds that were specifically developed to support research on Human-AI teaming.
- Testbeds that were built on a foundation provided by commercial games.
- Testbeds built on a foundation provided by open-source games.
Using the factor definitions, the research team developed a scoring standard, and then two researchers rated each of the testbeds selected for evaluation. After completing their evaluations, the researchers met to discuss any ratings that exceeded a difference of two points. These discussions aimed to identify cases where the raters may have applied the evaluation criteria differently.
2.2 Results
A casual review of the 19 testbeds allowed the research team to eliminate nine candidates without a detailed review. The researchers scored and ranked the remaining ten testbeds. The testbeds with the highest ranks were:
ASIST Saturn+. This testbed was developed within the Artificial Social Intelligence for Successful Teams (ASIST) program. ASIST aims to study Artificial Social Intelligence (ASI) as an advisor in all-human teaming. The Saturn+ testbed presented users with urban search and rescue scenarios within Microsoft’s Minecraft gaming environment.
ASIST Dragon. ASIST researchers also developed this testbed. It presents bomb disposal scenarios.
Black Horizon. Sonalysts, Inc. developed Black Horizon to allow students to master orbital mechanics fundamentals. In Black Horizon, each learner plays as a member of a fictional peacekeeping organization. Players learn to control an individual satellite and coordinate satellite formations with different sensor, communication, and weapon capabilities.
BW4T. Researchers from Delft University in the Netherlands and the Florida Institute for Human and Machine Cognition developed Blocks World for Teams (BW4T). In it, teams of two or more humans and agents cooperate to move a particular sequence of blocks from rooms in a maze to a drop zone.
SABRE. The Situational Authorable Behavior Research Environment (SABRE) was created to explore the viability of using commercial game technology to study team behavior. SABRE was developed using Neverwinter Nights™, produced by Bioware. Neverwinter Nights is a role-playing game based on Dungeons and Dragons. The team’s task was to search a virtual city (an urban overlay developed for the Neverwinter Nights game) and locate hidden weapons caches while earning (or losing) goodwill with the non-player characters.
Between the lines
Considering the results of the survey and analysis, none of the highly rated testbeds was an adequate fit for our needs. The research team opted to deprioritize Black Horizon because it did not provide a proper teamwork environment. SABRE was eliminated because it was not an open-source solution. The two ASIST testbeds were unsuitable because their architectures did not support synthetic teammates. BW4T was removed because it presented security challenges.
Instead, we used the lessons gathered during the review to develop a Concept of Operations for a novel testbed. The envisioned testbed would present team members with a time-sensitive search-and-recovery task in outer space. We are assessing whether we can affordably develop the testbed and release it as an open-source environment.