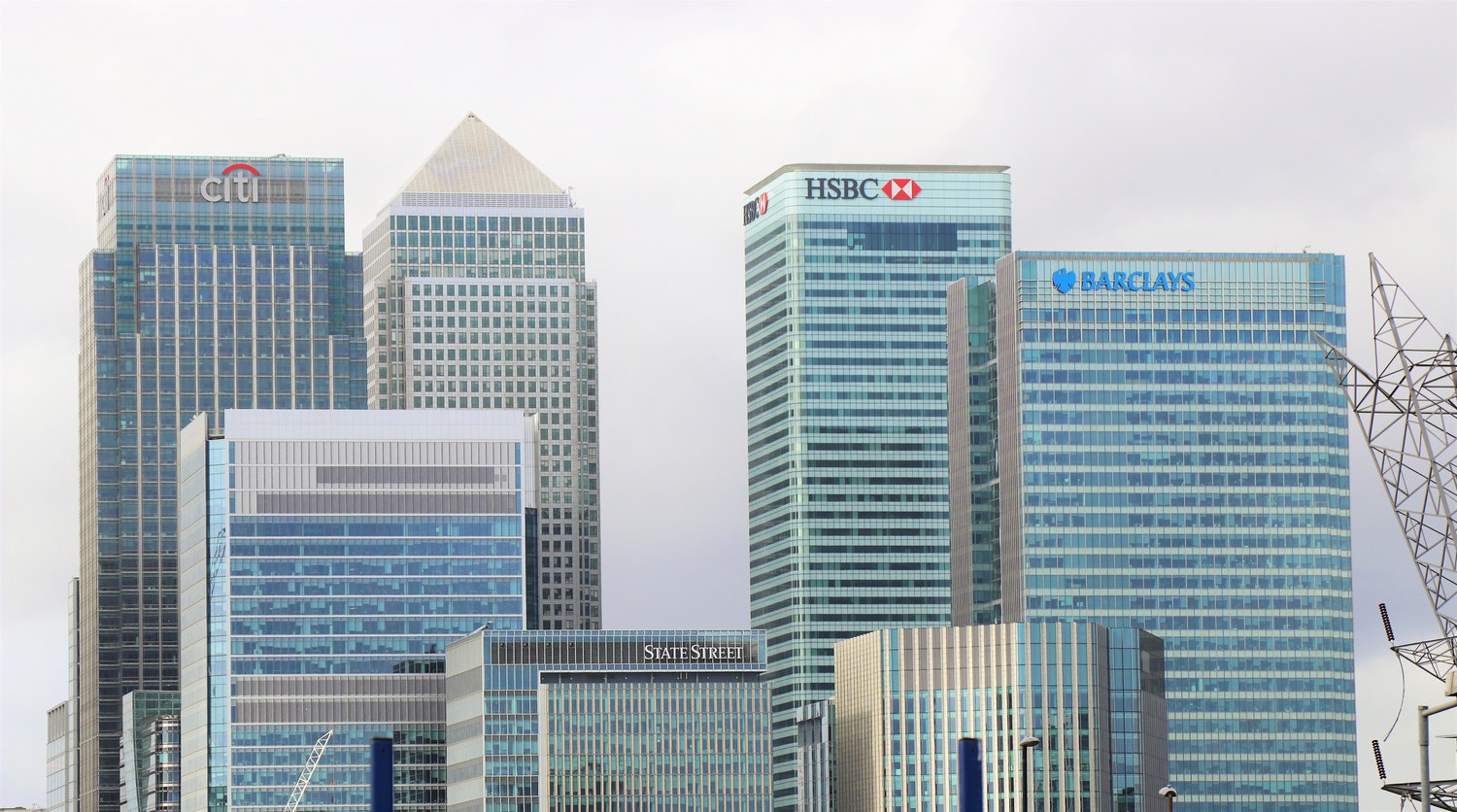
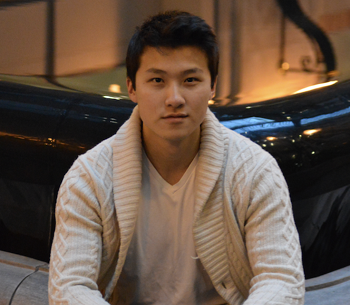
In this guest post, Jimmy Huang (Subject Matter Expert for Data Pooling at TickSmith) explains the origin story of data pooling in the banking sector, and its ethical implications in an increasingly AI-driven world. TickSmith powers your mountains of data, and is the Capital Market industry’s first end-to-end data management platform. There, Jimmy leads the design and implementation of data pooling software of OTC asset classes for CanDeal and the 6 largest Canadian banks to support risk and data monetization initiatives.
Preface
As with most innovative and far-reaching concepts, we must pay detailed attention to the topic at hand without oversimplifying, diverging, or getting lost in jargon, especially when dealing with financial language. I will provide a succinct and approachable account of financial data pooling. However, the groundwork will take some time to build — the context of why and how data pooling has come about in the banking sector is crucial in determining its implications in an increasingly AI-driven world.
Groundwork
Data pooling within the financial context refers to taking in sensitive data from multiple organizations to derive higher level insights otherwise impossible to achieve. The concept is innovative in the business world with applications in monetizing data products and in the technological world for the big data capabilities as well as the entitlement functions needed to support the platform.
I occupy a niche specialty in this world of financial data and technology. As the subject matter expert for the technology used to pool private pricing data at TickSmith, a big data company based in Montreal, my skill set is simultaneously particular and cross-functional.
To give a brief overview, for the past 3 years I led the collection, anonymization, and pooling of sensitive content from the 6 largest banks in Canada to generate complex reports using big data technology on the cloud, in particular Amazon Web Services (AWS), for all the bank participants. CanDeal, a fixed income exchange in Canada, formed a data services group called DataVault Innovations that uses our technology to pool data from the following 6 banks in Canada: BMO Nesbitt Burns Inc., CIBC World Markets, National Bank Financial Inc., RBC Capital Markets, Scotia Capital, and TD Securities. To my knowledge, this is the first product in history that facilitates the mass-sharing of sensitive content on the cloud between all major competitive banks within a country. This comes with many implications both positive and negative depending on how such a platform is put into production and how it is to be used.
In line with the Montreal AI Ethics Institute code of ethics, I believe that if there is a use case driving innovation it should be explored with careful analysis and implemented in a responsible way.
Use Cases
Since the 2007-08 financial crisis, financial regulators internationally have strengthened market risk controls. Essentially requiring banks to price assets and assess risk more accurately as well as to keep more capital on-hand to weather the default of, for example, debt-related assets to ensure another financial crisis does not occur. For the Canadian banks, this was the primary reason to implement a data pool.
There are 4 major use cases that a data pool can address for the banks:
- 1. To prove a wider set of prices for instruments that aren’t traded as often (to price securities more accurately)
- 2. To generate higher-level derived data sets and analytics impossible using just one individual bank’s data
- 3. To catalogue previously disorganized data and to help feed validated data into other downstream systems (help internal IT teams)
- 4. To package and monetize joint data products
Reactions
On February 27, 2020, I was invited to speak at the Financial Information Services Association (FISD) Technology Forum in the Great Hall of the J.P. Morgan building in London, UK.
My panel was the last event in the forum with the topic being “Data pooling and leveraging big data technology for regulatory compliance”. The format was to be a fireside chat between me and Richard Caven, the Financial Services Business Leader for Amazon Web Services (AWS). Conference listeners were most interested in how feasible it was to implement the data pool as well as the pros/cons of the product.
In terms of implementation feasibility, each country or region must evaluate for themselves the following 3 factors:
- 1. Homogeneity of traded instruments (to determine efficacy of the data pool)
- 2. Fragmentation of the banking sector
- 3. Competition laws
One main concern is whether data pooling is intrinsically anti-competitive. In my opinion, it is not, if implemented in the right way. If the pool was made public and the time-to-market on the work required to ingest new data sets from new banks is short enough, then the barriers to entry looks to be sufficiently low such that anyone can join relatively easily.
As for the pros and cons of the product, this leads us to…
The Data Pool’s Implications
The benefits of the data pool is self-evident. Market risk teams in banks can more accurately price assets they hold and can trade at lower fees as a result – this may translate to billions of dollars for Canadian banks alone. Under the new FRTB regime, banks may not have to slash the trading desks they currently have that would otherwise be unprofitable.
The risks to data pooling are more subtle and with many implications we will not even know until it occurs. One conference member from Google asked me during the Q&A section of my talk: what if someone lets loose a machine learning algorithm on the anonymized pooled data to attempt to de-anonymize it and associate a trade with a counterparty? Will it succeed?
Perhaps it will. Pooled price data is only useful insofar as it has content used to identify the instrument and other complementary attributes to the data such as the price, quantity, coupon, etc. The more fields we redact, the data will be made more secure and anonymized, but it becomes decreasingly useful until we can’t even identify what kind of data we are looking at.
Perhaps with a certain measure of confidence, an advanced algorithm can determine the specific banks that an anonymized trade comes from. This can invariably happen given that we put any useful morsel of content in data. However, this does not mean it is too much of a risk to take.
This is already happening and it has always been happening in the financial world albeit on a much smaller scale. A corn futures trader, for example, standing in the pits of the Chicago Board of Trade for years understands the “corn futures” contract so intimately that by seeing a trade occur along with the timing of it, the price it trades at, and a host of other nuances in the pits, this trader can reasonably deduce who the client is giving the order to the traders.
The power of AI in this case is not that it can derive insights humans are unable to – but that it can derive them at a scale and retain consistency.
Our job then is to continually innovate but at the same time make sure that as a society we can adapt to the implications of what we build. The data pool will allow for more participating banks to try to game a competitive advantage with algorithms analyzing the pooled datasets.
Over the course of history, we have seen societal problems eradicated not because the issue at hand has been “solved” in a traditional sense but our reaction to the problem has changed. We may realize that the perceived problem we had in the first place is no issue at all with the right perspective. This brings to mind the idea of the “holy fool”. In many societies over history, schizophrenic qualities have been interpreted as a divine blessing as opposed to a disease to be cured. In Russian history, for example, the yurodivy or holy fool is a form of Eastern Orthodox asceticism where what we call an individual with mental illness does not pose a harm to society nor the subject as it is an acceptable way to live that is ingrained within the society’s tolerances.
Therefore our response to AI on data pooling is conjointly to see what data content we may further redact to limit what can be derived and to adapt how we react to data pools being a reality to stay.