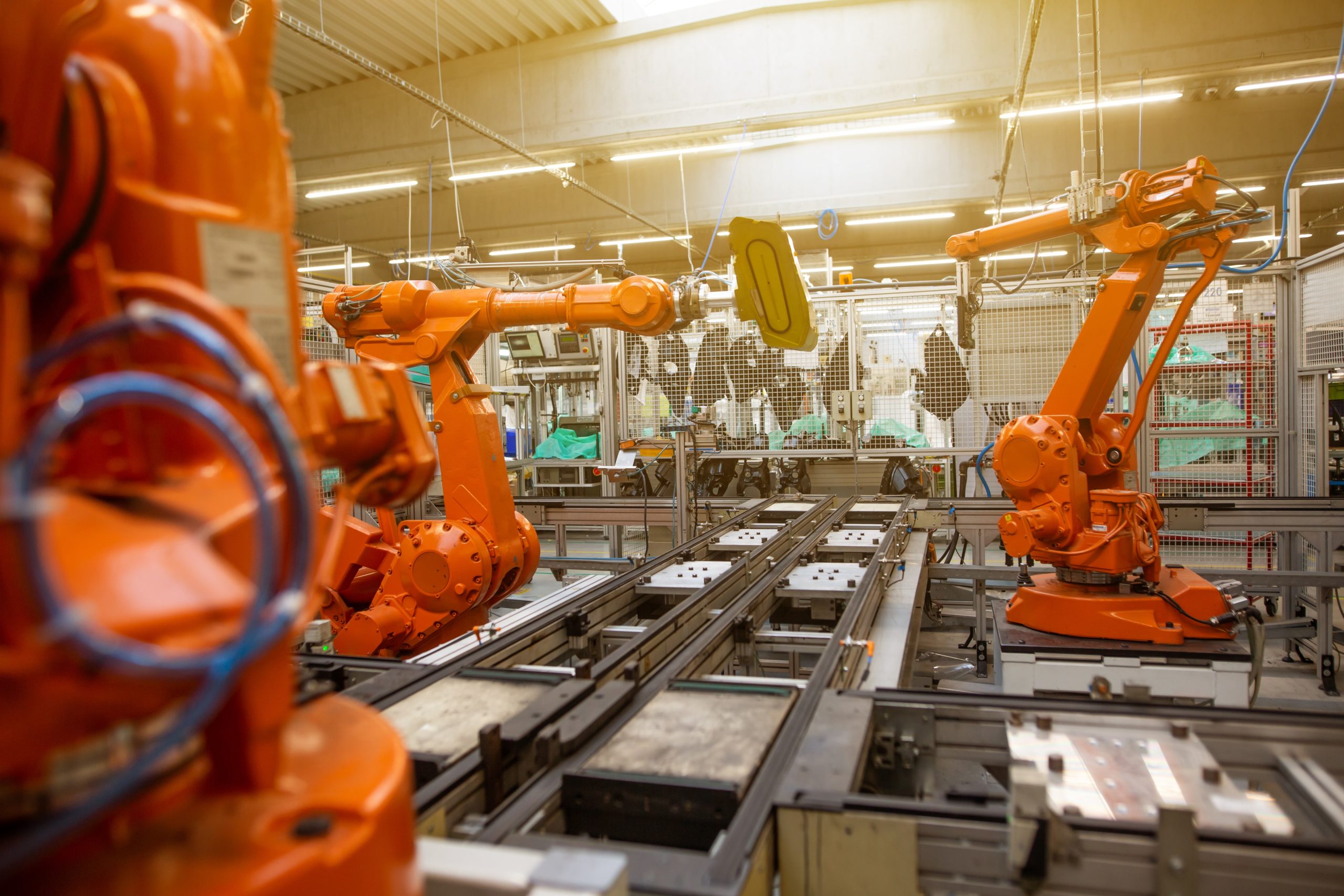
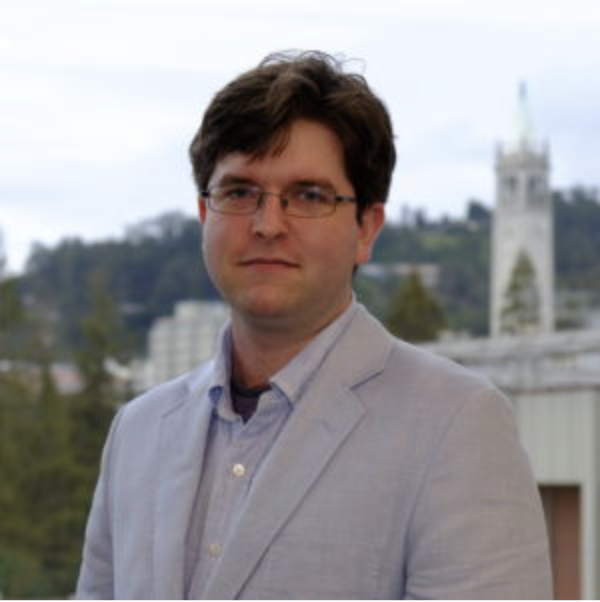
🔬 Research Summary by Thomas Krendl Gilbert, a Postdoctoral Fellow at Cornell Tech’s Digital Life Initiative, and has a Ph.D. in Machine Ethics and Epistemology from the University of California, Berkeley.
[Original paper by Thomas Krendl Gilbert, Sarah Dean, Tom Zick, Nathan Lambert]
Overview:  This white paper introduces “Reward Reports,” a new form of documentation that could help improve the ability to analyze and monitor AI-based systems over time. Reward Reports could be particularly useful for trade and commerce regulators; standards-setting agencies and departments; and civil society organizations that seek to evaluate unanticipated effects of AI systems.
Introduction
Many of the most interesting and complex questions for ethical AI are about the longer-term effects and behaviors of real systems. For example, beyond avoiding road collisions, how will self-driving car fleets subtly change the flow of traffic over time? How can electricity be distributed reliably and equitably even as demand fluctuates month by month? How can social media platforms encourage meaningful engagement without prompting the production of increasingly divisive content? To address these challenges, we need to document the types of feedback at stake in AI systems, not just the data they observe or the models they learn.Â
The white paper introduces a framework for documenting deployed learning systems, called Reward Reports. The authors outline Reward Reports as living documents that track updates to design choices and assumptions behind what any particular automated system is learning to optimize for. Reward Reports will make it possible to apply more powerful legal standards to AI, making their designers liable for different types of harms. They will also help supply designers with stakeholder feedback that informs them about the system’s performance over time. Policymakers and civil society organizations could then use them to make AI systems accountable to the public interest.
Key Insights
What is reinforcement learning?
At present, there is a lot of technical work on how to make artificial intelligence (AI) applications that are fair. This means that the data they use and the models they learn portray people accurately, and without causing harm. But the most difficult ethical challenges in AI transcend one-off decisions, and are instead about problems that are fundamentally dynamic. Fortunately, an emerging kind of AI called reinforcement learning (RL) promises to solve dynamic problems by learning from different types of real-time feedback rather than historical data alone. You can think of RL as automating what engineers already do with machine learning: feed data to a classifier, which learns a model, which is then used to make decisions, monitored to make sure it performs well, and retrained on new data as needed. RL is a framework for describing how to do all this automatically, without needing manual inputs from humans. This is why many experts consider RL to be the single most likely path to artificial general intelligence — machines that can do pretty much everything humans can do, including teaching themselves how to do things.
What are the potential harms of reinforcement learning?
For all its potential benefits, RL poses unique challenges. Human designers still have to specify an environment for the system to learn to distinguish types of feedback. This environment has rewards that the system is trying to maximize, which the designer hopes will approximate good behavior. Specifying these rewards incorrectly may cause the system to adopt behaviors and strategies that are risky or dangerous in particular situations. For example, a self-driving car may ignore pedestrians if it is only rewarded for not hitting other cars. On the other hand, a fleet of cars may learn to aggressively block merges onto certain highway lanes in the name of making them safe. If the RL system has not been set up to learn with feedback well, then the system could do great damage to the domain (in this case, public roadways) in which it operates. We conclude the following:
- Misspecifying rewards will cause the system to learn behaviors that are at odds with the normal flow of activity. This will tend to contort the dynamics of human domains around the system.
- As RL-driven AI systems become more capable, they will strive to control domains as well as behave well within them. This trend has an inherent affinity with monopoly power.
- The tendency towards monopolization is not well-captured by existing regulations and forms of oversight. New checks and balances are needed to ensure these design risks are minimized.
- Distinct design risks will manifest for particular human activities. RL-appropriate regulations must be domain-specific, and incorporate ongoing feedback from stakeholders to ensure safety.
How can Reward Reports help address these challenges?
Reward Reports are intended to engage practitioners by revisiting design questions over time, drawing reference to previous reports and looking forward to future ones. As pivotal properties may not be known until the system has been deployed, the onus is on designers to sustain documentation over time. This makes Reward Reports into changelogs that both avoid the limitations of simple, yes-or-no answers and illuminate societal risks incrementally. Moreover, Reward Reports serve as an interface for stakeholders, users, and engineers to continuously oversee and evaluate the documented system. Hence, Reward Reports are a prerequisite to accountability for the system’s dynamic effects.
A Reward Report is composed of multiple sections, arranged to help the reporter understand and document the system. A Reward Report begins with system details that contain the information context for deploying the model. From there, the report documents the goals of the system and why RL or ML may be a useful tool. The designer then documents how it can affect different stakeholders. Reports must also contain technical details on the system implementation and evaluation. The report concludes with plans for system maintenance as additional dynamics are uncovered.
Between the lines
Reward Reports build on the documentation frameworks for “model cards” and “datasheets” proposed by Mitchell et al. and Gebru et al. As a form of ongoing rather than one-off documentation, they will support standards for good system behavior beyond fairness or accuracy tradeoffs. Furthermore, Reward Reports make use of RL’s technical language to approach design problems more dynamically. However, we outline Reward Reports as living documents that track updates to design choices and assumptions behind what any particular automated system is learning to do, RL or otherwise. They are intended to capture and make sense of the longer-term effects of automated systems on human domains, filling an important gap in AI ethics and public policy.
Many questions remain about the applicability of this framework to different RL systems, behaviors that are difficult to interpret, and static vs. sequential uses of machine learning. At a minimum, Reward Reports are a major opportunity for practitioners to deliberate on these questions and begin the work of deciding how to resolve them in practice with stakeholders.