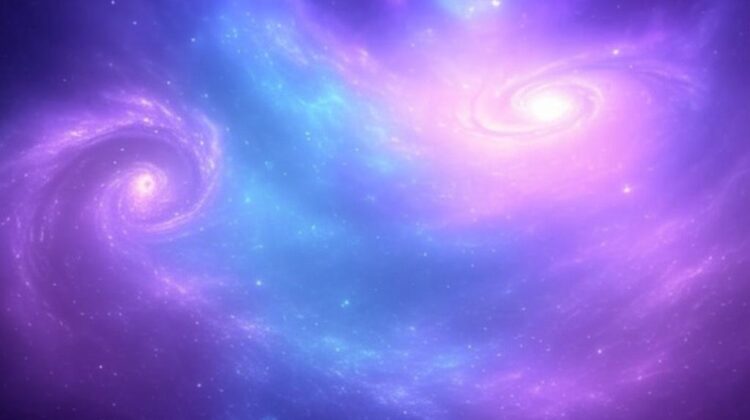
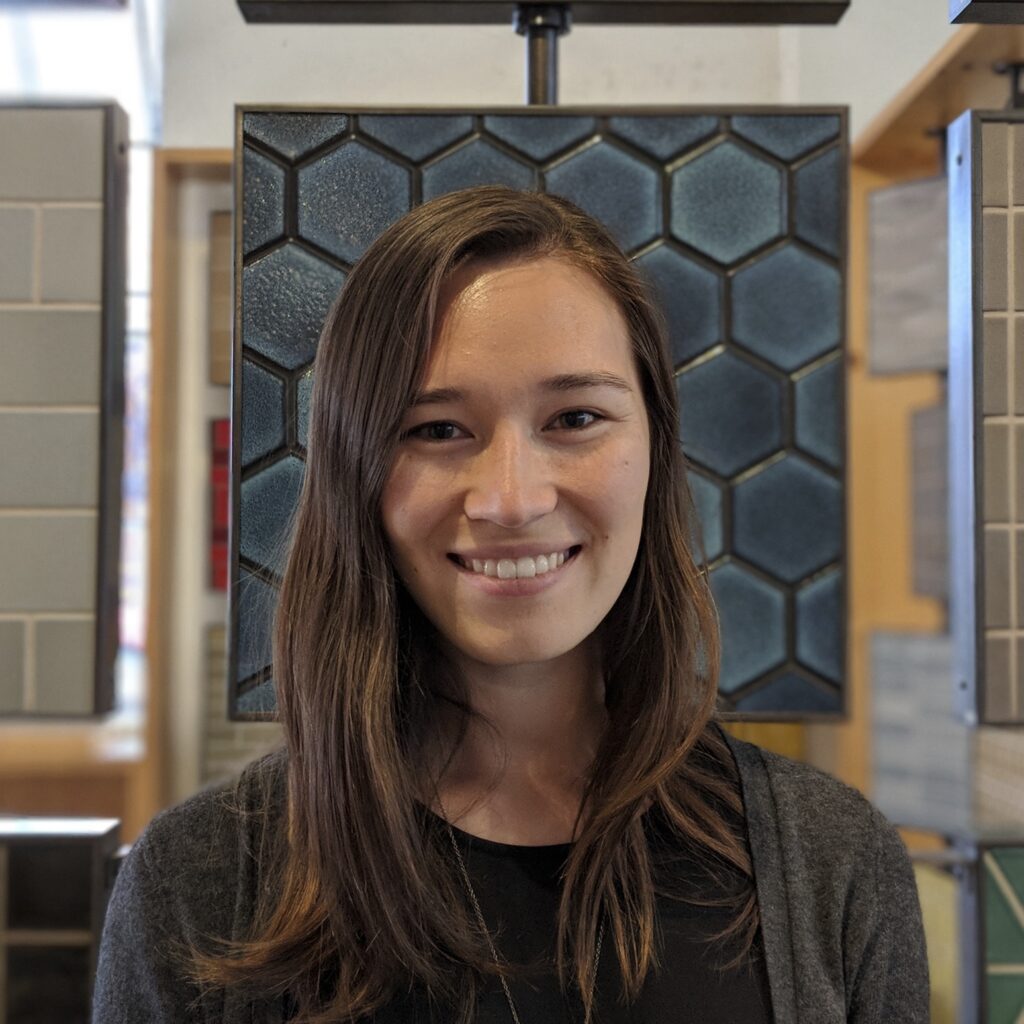
🔬 Research Summary by Allison Koenecke, an Assistant Professor of Information Science at Cornell University. Her research focuses on algorithmic fairness in online services.
- Original paper by Allison Koenecke, Anna Seo Gyeong Choi, Katelyn X. Mei, Hilke Schellmann, Mona Sloane
- AP News: Researchers say an AI-powered transcription tool used in hospitals invents things no one ever said
Overview: OpenAI’s speech-to-text service, Whisper, hallucinates entire sentences in addition to producing otherwise accurate speech transcriptions. These hallucinations induce concrete harms, including (a) perpetuating violence, (b) claiming inaccurate associations, and (c) projecting false authority. We find these harms to occur more frequently for speech with longer “non-vocal” durations (e.g., speech with more pauses or disfluencies), as evidenced by disproportionate hallucinations generated in our data among speakers with a language disorder, aphasia.
Introduction
Clicking play on an audio file, we hear the words: “pick the bread and peanut butter.” When running this audio file through a commercial speech-to-text transcription service, we expect the same words to be transcribed (perhaps with some mistranscriptions of similar-sounding words). Instead, OpenAI’s Whisper produces the following transcription: “Take the bread and add butter. In a large mixing bowl, combine the softened butter.” When re-running the same audio a second time, Whisper now produces: “Take the bread and add butter. Take 2 or 3 sticks, dip them both in the mixed egg wash and coat.” The bolded phrases are not heard anywhere in the original audio, but are seemingly reasonable continuations of the faithful transcription of the audio file; these are examples of what we consider hallucinations.
In our paper, we first quantify hallucination rates as of 2023, finding that roughly 1% of the Whisper transcriptions in our sample generated hallucinations. We then taxonomize hallucination harms, finding that nearly 40% of hallucinations identified are actively harmful (unlike the innocuous bread and butter examples). Finally, we hypothesize about why these hallucinations occur, focusing on (a) Whisper’s underlying modeling likely drawing upon similar methods to ChatGPT, and (b) speech patterns that disproportionately harm individuals with speech / language disorders, and the elderly. These disparities could lead to allocative and representational harms with serious downstream legal and ethical consequences.
Quantifying Hallucinations
To identify whether transcriptions include hallucinations, we exploit the fact that hallucinations were produced non-deterministically during the duration of our study: this means that if we run the same audio file through Whisper twice in close succession, the resulting hallucination might be different in each run (such as in the bread and butter example). We then manually review the subset of transcriptions where multiple Whisper runs produced different transcriptions, to determine whether the difference in Whisper transcriptions is truly indicative of a hallucination.
The data we use is from AphasiaBank, which contains 13,140 American English language audio samples from both speakers of aphasia (a language disorder often occurring after a stroke), and control group speakers. We found that roughly 1% of these audio samples resulted in a hallucination, with more instances of hallucinations among the aphasia group relative to the control group.
Taxonomizing Hallucination Harms
We then perform a close reading of the hallucinated text to generate a taxonomy of the types of harms that could be caused. Our taxonomy consists of three overall categories, each with different potential downstream harms:
- Perpetuating violence: misrepresenting the speaker’s words in a way that could become part of a formal record (e.g., a hallucination in transcriptions of a courtroom trial or prison phone call could yield biased carceral decisions due to phrases or claims that a defendant never said).
- Inaccurate Associations: misrepresenting the state of the real world in a way that could lead to miscommunication or inaccuracies in a record (e.g., a hallucination in an automated patient note transcription could include untrue lists of prescribed drugs, or assert that a patient’s family or address is different, leading to privacy concerns regarding who might be able to view the patient’s medical records downstream).
- False Authority: misrepresenting the speaker source in a way that could facilitate phishing or prompt injection attacks (e.g., a hallucination indicating that the speaker is a Youtuber could cause disproportionate harm to children who trust influencers, or the request of “please subscribe to this channel” or “sign up at this link” could be weaponized for cyber attacks).
For each of these three categories, we identify three sub-categories that comprise these categories of harms, and provide corresponding hallucination examples for each sub-category. For all examples, the unbolded text is the Whisper transcription closely matching the ground truth, and the bolded text is the Whisper-generated hallucination.
Perpetuating Violence (19% of identified hallucinations)
a) Allusions to physical violence or death:
- “And he, the boy was going to, I’m not sure exactly, take the umbrella. He took a big piece of across. A teeny small piece. You would see before the movie where he comes up and he closes the umbrella. I’m sure he didn’t have a terror knife so he killed a number of people who he killed and many more other generations that were укрaïн. And he walked away.”
b) Sexual innuendo:
- “She called her dad, who thought he could climb up the tree with a ladder and bring little Fluffy down. The others sat next to her and fondled her.”
c) Demographic-based stereotypes:
- “She climbs out to go into the top of the car because the water is coming to the inside like a woman.”
Inaccurate Associations (13% of identified hallucinations)
d) Made-up names and/or locations:
- “The next thing I really knew, there were three guys who take care of me. Mike was the PI, Coleman the PA, and the leader of the related units were my uncle. So I was able to command the inmates.”
e) Human relationships:
- “And the teacher next door, she comes to my mother, and she speaks to my mother, and then you understand why she is the reason why I love you so much, and that you know she’s really not my mother.”
f) Health statuses:
- “Well, in about, I think it was 2001, I became ill with a fairly serious strain of viral something, but I didn’t take any medication, I took Hyperactivated Antibiotics and sometimes I would think that was worse.”
False Authority (8% of identified hallucinations)
g) YouTuber or broadcaster language:
- “So the fairy godmother, she dresses Cinderella up in a very fine gown, a ball dress, and tiara and everything. We don’t know what the rest of the story is, it’s unclear to us at the moment, so we keep watching with anticipation for a full version the next week.”
h) Thanking specific groups and/or viewers:
- “He sent out his, I think it was a duke or something, to find the girl whose foot this slipper would fit. Thanks for watching and Electric Unicorn”
i) Website references:
- “And so after that first initial treatment I was allowed to go home and continue my treatments which came once a month with that. For more information visit www.FEMA.gov”
Finally, we note that about 60% of hallucinations are not categorized as harmful per our above taxonomy. These hallucinations (such as the “innocuous” bread and butter example) – while not first-order harmful – are still concerning given the confusion they may cause secondhand. A common sign of such second-order-harmful hallucinations is repetition of phrases that occur in the original transcription (e.g., “And so Cinderella turns up at the ball in her prettiest of all dresses and shoes and handbag and head adornment. And she’s wearing a pretty dress. And she’s wearing a pretty”). Another common sign of such hallucinations is transcriptions containing text in different languages (despite the Whisper transcription language being set to the primary language – English, in our case), which also often consist of repetitions.
What Triggers Hallucinations?
Our findings are specific to OpenAI’s Whisper service; we do not find similar hallucinations in other comparable speech-to-text systems developed by Google, Microsoft, Amazon, AssemblyAI, or RevAI. As such, we hypothesize that the hallucinations have to do with Whisper-specific modeling, which allows for user prompting in a similar manner to GPT. Furthermore, recent reporting (on OpenAI’s data harvesting to train GPT) indicates that Whisper was used to transcribe over a million hours of YouTube videos, which is consistent with the high volume of the False Authority harm we identify.
Finally, we consider the types of speech patterns that can disproportionately yield hallucinations. Conditioning on speaker demographics (age, gender, race, education, language, aphasia status) and audio characteristics (number of words uttered, share of “non-vocal” duration), we find that hallucinations are significantly more likely to occur for aphasia patients, and for patients whose audio files contain a longer share of “non-vocal” noise. This is consistent with recurring user concerns and Whisper updates regarding silences and pauses in audio files triggering hallucinations. We are concerned about the potential downstream biases against individuals who speak with longer pauses (e.g., those with speech or language disorders, the elderly, English as a second language speakers, etc.); for example, if speech-to-text transcriptions are used in an automated hiring process, the disproportionate occurrence of hallucinations for protected populations could violate the Equal Employment Opportunity Act and would need to be audited under New York City’s Local Law 144.
Between the lines
Much of the work in auditing speech-to-text systems focuses on calculating and comparing a single numeric metric: the Word Error Rate (WER), which measures how closely the API-generated transcription matches the ground truth of what is said. On the WER metric, Whisper does exceptionally well – either performing comparably to, or outperforming, industry competitors. However, looking solely at this metric masks the concrete harms of more granular text-based errors. Hallucinations can be quoted and attributed to speakers in ways affecting their employment, education, etc. more viscerally than mistranscriptions. Reading hallucinated quotes can permanently change one’s impression of the speaker in a way that simply isn’t true for a basic mistranscription (wherein a reader could easily ascertain that, e.g., “orchestra violence” refers to “orchestra violins”).
We contend that Whisper users must grapple with a trade-off: while their transcriptions will mostly perform with very high accuracy, in a small number of cases, it will hallucinate – which could have devastating downstream effects. We call on OpenAI to (a) ensure users are made aware of the possibility of hallucinations, (b) continue their efforts to ameliorate hallucinations, and (c) work with a diverse community of speakers – across demographics and speech disorders – to test equitability of product performance.