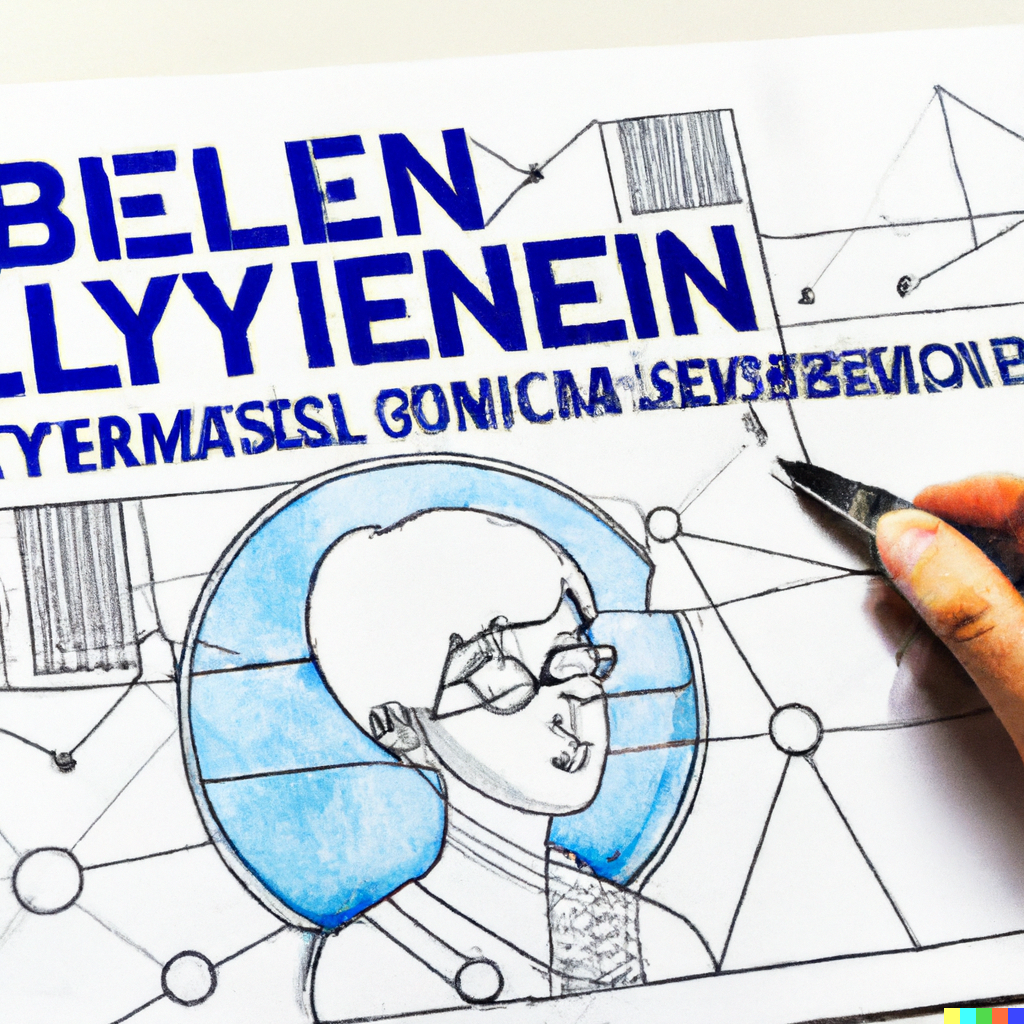
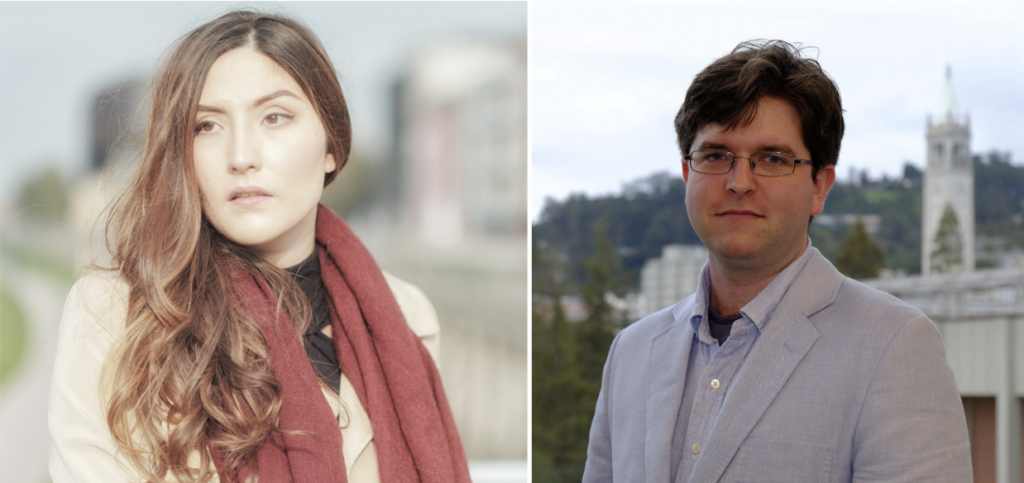
🔬 Research Summary by Megan Welle Brozek and Thomas Krendl Gilbert
Megan is the CEO and co-founder of daios, a deep tech AI ethics startup, and a background in the philosophy and methodology of science.
Tom is a Postdoctoral Fellow at Cornell Tech’s Digital Life Initiative, has a Ph.D. in Machine Ethics and Epistemology from the University of California, Berkeley, and leads AI Ethics research at daios
[Original paper by Thomas Krendl Gilbert, Megan Welle Brozek, Andrew Brozek]
Overview: The fixation on bias and compliance within the AI ethics market leaves out actual ethics: leveraging moral principles to decide how to act. This paper examines the current AI ethics solutions and proposes an alternative method focused on ground truth for machines: data labeling. The paper builds a foundation for how machines understand reality, unlocking the means to integrate freedom of individual expression within AI systems.
Introduction
The hype around AI has greatly increased with the emergence of large language models (LLMs) such as ChatGPT, Stable Diffusion, Midjourney, etc. More people can understand and experience AI as a technology, but trust in AI still needs to be higher.
Many AI ethics solutions exist to improve trust between users and AI, but most solutions have a myopic focus on bias and compliance with current legal standards. Neither of these approaches captures what it means to be ethical: behaving according to moral principles or, in other words, doing what is right.
This paper emphasizes the relative absence of ethics within AI ethics. We analyze current AI ethics solutions and describe an alternative method that allows for the creation of a neutral adjudicator able to identify and alter ethical values within AI systems. The paper also elaborates on the philosophical starting points underlying creating products and operations within daios to help realize a true moral character.
Key Insights
The Present Landscape of AI Ethics Solutions
The first section evaluates the industry’s current landscape of AI ethics solutions. One approach is frameworks and governance, such as Responsible AI or Explainable AI, which are a checklist too rigid to keep pace with AI development.
Another approach is to create new development tools that shed light on specific design considerations, such as models or data. Companies such as Arthur, Holistic AI, or Fairplay fall into this category. Although an effective measurement technique for pre-defined harm or risk, these approaches need more ethical efficaciousness when either is absent.
A group of companies also focus on achieving technical breakthroughs for “aligned” AI, such as Anthropic, OpenAI, and Aligned AI. Large language models (i.e., LLMs) use human-in-the-loop techniques (such as reinforcement learning human feedback (RLHF) or constitutional AI) but rely on one set of ethical values that end users may disagree with.
The Interlude: A Need for an Alternative
The myopic focus on bias and compliance in AI ethics is a symptom of a larger problem, conflating ethics with law, technical solutions, and pure theory.
Ethics as law
Compliance with present legal standards and prospective government regulations is necessary, but more is needed, as this perspective pigeonholes ethics into what is permissible rather than what is good.
Ethics is an issue that belongs in civil society, requiring deliberating about right and wrong. Conflating vices with crimes and virtue with conformity leads to unhappiness and civil strife.
Ethics as technical
Ethical AI/MLOps companies often rely on generally accepted definitions of a “good ethical system,” relying on vague terms such as “fairness,” “responsible,” and “bias.” The question becomes, “Fair to whom?” “Responsible to whom?” “Biased against whom?” The answer differs according to different individuals in different contexts. Ultimately, the problem of “whose ethics should be built into this system” is pushed down the road.
Ethics as purely theoretical
Some companies approach AI ethics in a research-only manner, combining philosophy, computer science, economics, and ethics. These companies may be concerned with long-term AI ethics, such as AI as an existential threat.
But AI/ML systems are already being deployed by companies all over the globe. Separating high-level theory and engineering practice ends in research questions becoming speculative and unanswerable in the current paradigm.
An alternative framing
With a proper understanding of ethics, the market widens to teach AI certain ethical values. This is a more active stance in algorithm creation and the vision of daios.
The daios method
We offer an alternative method, which posits that the way data is labeled plays an essential role in how AI behaves and, therefore, in the ethics of the machines themselves.
We get to this conclusion by combining two fundamental insights:
- data determines truth for machines
- ethics is about behaving according to moral principles
A machine executes based on what it has been told to do, dictated by programming. In the case of machine learning, a machine is programmed to learn based on example tasks in the form of training data.
This alternative method allows for a neutral adjudicator between companies and users that can identify and alter ethical values within AI systems.
Axioms for Ethical AI
This section elaborates on the philosophical starting points for creating the daios product and operations within the company.
Technology obscures individual moral choices.
AI technology is Hegelian, or system-oriented, with individuals losing their ability to participate as specific entities but only as data sources in the whole AI feedback loop. Individuals cannot express their identity, agency, and choice.
Practice and theory should be intertwined.
The creative process must be given space to realize its vague urges that may not make sense to eventually come to truths that can be made sense after the fact.
Being ethical requires acting with intent.
Today’s AI is prone to fail whenever there is more than one interpretation of the task–more than one set of possible rules–at hand. In these situations, humans must intentionally intervene and introduce new criteria to distinguish types of values or input labels explicitly.
Observation or judgment is always made from the subject’s point of view.
Any collection of data points is meaningless without a theory arranging it in a particular way. Even if an objective world exists, there is no such thing as objective ethics.
The current daios product
Daios teaches machines morality by co-creating algorithms with end users. The solution connects technical aspects that determine reality for machines, e.g., data, with the human element, such as AI development teams and those otherwise without a voice, the end-users.
Between the lines
Neither legal compliance nor scalable computation is viable for giving AI systems moral virtue. Rather, the path to ethical AI starts with data. Ethics must be built directly into the data on which AI models are trained. Only in this way will the system reflect the subjectivities of those most impacted by its performance and whom it is meant to serve.
Observing the AI’s subsequent performance, we remake those activities and learn more about what we want. A positive feedback loop can emerge between our assumptions about what is good and how the system learns from us over time. Putting ethics into data is not about making AI conform to a rigid moral scheme–it’s about becoming better, more fully-realized versions of ourselves.