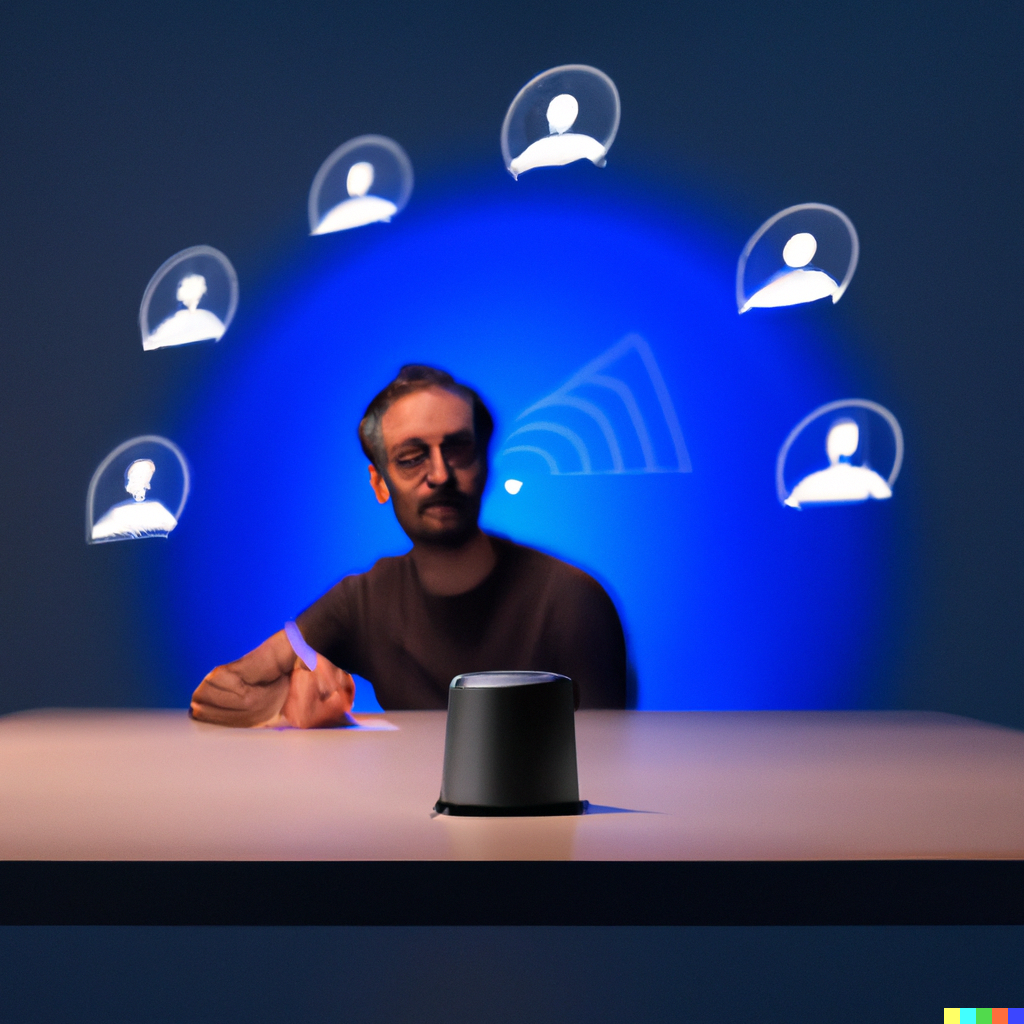
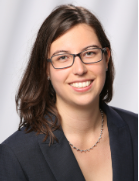
🔬 Research Summary by Anna Leschanowsky, a research associate at Fraunhofer IIS in Germany working at the intersection of voice technology, human-machine interaction and privacy.
[Original paper by Casandra Rusti, Anna Leschanowsky, Carolyn Quinlan, Michaela Pnacek(ova), Lauriane Gorce, and Wiebke (Toussaint) Hutiri]
Overview: Datasets lie at the heart of data-driven systems. This paper uncovers the influence of dataset practices on bias, fairness, and privacy within data-driven systems, particularly in the context of speaker recognition technology. The authors analyze usage patterns and dynamics from a decade of research in this field and demonstrate how datasets have been instrumental in shaping speaker recognition technology.
Introduction
Imagine a world where your voice is the only key you need, rendering passwords and PIN codes obsolete. This future is within reach thanks to the widespread adoption of speaker recognition technology across sectors like banking, immigration, and healthcare.
But have you ever wondered about the underlying drivers that enable this remarkable technological advancement?
The authors, who collaborated on the FairEVA project (https://faireva.org), unravel the story behind the datasets that underpin speaker recognition technology and explore their impact on bias, fairness, and privacy. The authors examined almost 700 papers from the past research decade on speaker recognition technology. They demonstrate that datasets primarily emphasize addressing technical challenges, with less attention given to demographic representation concerns. They also highlight changes in data practices since the rise of deep neural networks in speaker recognition, which have raised significant concerns regarding privacy and fairness.
Despite the rapid advancements in this field, pressing ethical questions regarding bias, fairness, and privacy within speaker recognition have remained largely unexplored. Their research underscores the need for ongoing investigations into dataset practices to address these issues.
Key Insights
Bias in Biometrics and Data
Biometric systems are the modern-day gatekeepers of our digital world and use our unique characteristics, like our faces or voices, to safeguard our valuable assets. From unlocking smartphones to accessing services, biometric technology has become integral to our daily lives. These systems are driven by complex machine-learning models and are not immune to bias.
The root cause often lies in the very datasets used to train and evaluate them. Datasets are the building blocks of these models, but they frequently fail to accurately represent the diversity of the real world. While previous studies have delved into dataset usage in the realm of face recognition, this paper takes a different path. The authors review nearly 700 papers presented at a prominent international speech research conference between 2012 and 2021 to investigate the usage of datasets within the speaker recognition research community.
The NIST Speaker Recognition Evaluations
The NIST Speaker Recognition Evaluations (SREs) serve as a crucial benchmarking resource in the world of speaker recognition research. These evaluations are regularly released by the National Institute of Standards and Technology (NIST) to foster the development of speaker recognition technology. The authors explain that “the NIST SREs were both users and drivers of these dataset collections, as annual evaluation challenges required new datasets to evaluate speaker recognition technology in ever more difficult settings.”
Dataset Usage in Speaker Recognition
To identify usage patterns, the authors distinguish between datasets that were used for training speaker recognition systems and datasets used for evaluation of the systems. However, identifying training and evaluation datasets proved challenging due to naming inconsistencies. The authors created “dataset families” that represent datasets more generally to address this.
They uncovered a staggering 185 unique training and 164 unique evaluation dataset families used over the past decade in speaker recognition. Despite this diversity, a handful of datasets, particularly the NIST SRE datasets, have dominated the research field.
One standout observation is the prominence of VoxCeleb datasets. These datasets were brought to life by the Visual Geometry Group (VGG) at the University of Oxford and were crafted by scraping YouTube celebrity videos. They aimed to create a large-scale speaker recognition dataset that captures real-world speech conditions. The VoxCeleb datasets marked a significant milestone as the first large-scale, freely available datasets for speaker recognition.
The authors’ research uncovered a concerning trend where many studies assess their systems using only one dataset, and only a few use more than three. This pattern reflects issues seen in the broader field of machine learning and indicates potential reliability issues within speaker recognition technology.
Dataset collection and bias
Data collection methods have a substantial impact on bias in speaker recognition datasets. The researchers show how the chosen data collection methods can lead to significant representation bias. For example, in some datasets, most participants were college students, creating a dataset skewed toward a younger demographic. In the case of VoxCeleb, which was scraped from YouTube, the researchers argue that the “automated processing pipeline reinforces popularity bias from search results in candidate selection.”
More than Bias: Privacy Threats and Ethical Questions
Finally, the research sheds light on substantial privacy concerns around these datasets. These concerns stem from the content of recorded conversations and the extensive metadata linked to them, which could enable the re-identification of participants. Additionally, web-scraped datasets like VoxCeleb lack consent from data subjects and have raised ethical questions due to the sensitive nature of voice data and its broad applications. These concerns underscore the need for rigorous data protection measures in the speaker recognition field.
Between the lines
Datasets are essential for the development of data-driven systems. Rusti et al. shine a light on dataset usage within the yet unexplored field of speaker recognition technology. Their research highlights how datasets are critical in shaping this technology and raises awareness of potential issues like bias, privacy, and ethics. The authors emphasize the importance of representative evaluation datasets and privacy-preserving voice processing to mitigate privacy risks. As speaker recognition technology becomes increasingly integrated into our lives, ensuring that it works equitably for all users while safeguarding people’s privacy is crucial.
This research serves as a wake-up call, urging the speaker recognition community to be mindful of dataset choices and ethical implications. It prompts us to ask questions about the impact of technology on society and the importance of fairness, transparency, and privacy in the development of AI systems on a broader scale.