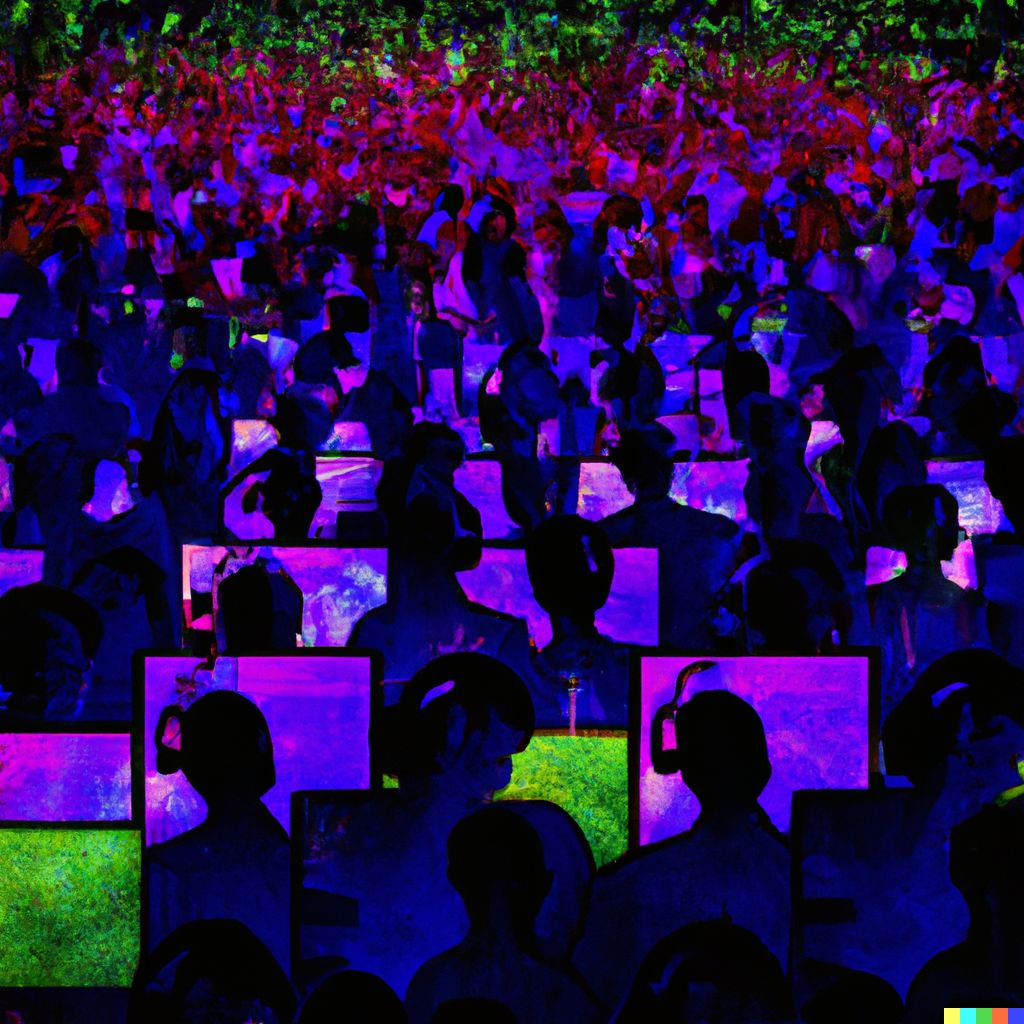
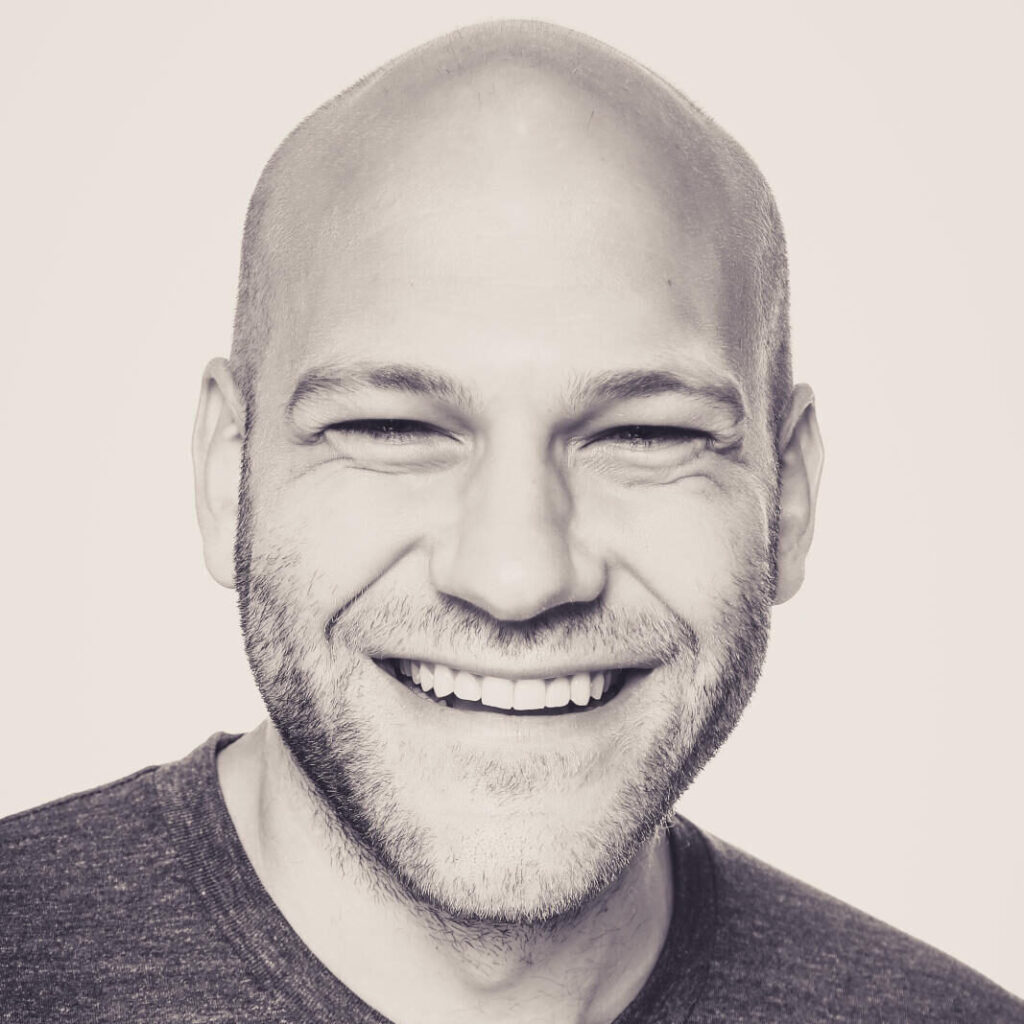
🔬 Research Summary by Orestis Papakyriakopoulos, a research scientist in AI ethics at Sony AI. His research provides ideas, frameworks, and practical solutions towards just, inclusive and participatory socio-algorithmic ecosystems.
[Original paper by Orestis Papakyriakopoulos, Anna Seo Gyeong Choi, William Thong, Dora Zhao, Jerone Andrews, Rebecca Bourke, Alice Xiang, and Allison Koenecke]
Overview: The lack of diversity in datasets can lead to serious limitations in building equitable and robust Speech-language Technologies (SLT), especially along dimensions of language, accent, dialect, variety, and speech impairment. To encourage standardized documentation of such speech data components, we introduce an augmented datasheet for speech datasets, which can be used in addition to “Datasheets for Datasets.”
Introduction
Many SLTs are used in high-impact scenarios: to support individuals with disabilities (e.g., the speech synthesizer used by Steven Hawking), to make driving safer, to help medical doctors transcribe patient notes, and to transcribe courtroom events.
Prior research studies have shown that such SLT applications can have disparate impacts on different populations, which can largely be attributed to the training data used. For example, a study in 2020 showed that speech-to-text error rates of top commercial speech assistants were twice as high for Black speakers relative to white speakers in the US.
Such biases often emerge because speech data selection & collection is a time and resource-intensive task, especially considering the different kinds of speech. For example, dataset creators and model developers should answer crucial questions about who should be included in the dataset regarding age, accents, dialects, gender & atypical speech. Furthermore, they should also consider data-subjects rights & privacy while making data collection feasible and useful for the SLT application.
Towards this end, we created a datasheet template, which provides explicit questions that speech dataset creators should answer, contributing to more ethical SLTs. Using the template by the research community can lead to more transparent & inclusive dataset creation and sharing.
Key insights
Our primary aim was to develop a set of considerations that can be useful to speech dataset creators and users when developing speech datasets and training machine learning models. Towards this, we performed a large-scale literature review & focused dataset evaluation to uncover existing limitations and detect properties that ethical & inclusive datasets should have. Based on the detected limitations and properties, we supplemented the existing datasheets for datasets with speech-specific questions that can guide researchers and practitioners in developing more robust and trustworthy SLTs.
Limitations of existing datasets & documentation
During our large-scale literature and dataset review, we found serious limitations regarding demographic diversity, numerous ethical questions remained unaddressed, and many dataset properties undisclosed, leading to confusion about dataset utility. For example, we found that less than a handful of datasets contained explicit recordings of non-binary individuals, while age diversity was very limited. Similarly, we found a systematic under-documentation on the speaking style of individuals in the recordings or about the privacy and compensation practices of data subjects during data collection.
Considerations
Based on the detected limitations and issues, we formulated questions that can inform transparent & ethical dataset development in different stages of the process. An overview of these questions for each stage can be found next:
(1) Motivation
How does a dataset creator determine which linguistic subpopulations (age, gender, dialect, nationality, etc.) are the focus of the dataset, as well as for which purpose is the dataset going to be used (e.g., read or spontaneous speech)?
(2) Composition
How much data does a dataset creator collect for each subpopulation? What topics are included in the recordings, and is it ensured that the content does not conflict with the values of data subjects and users (religious, cultural, political values)?
(3) Collection Process
Are speech recordings collected in a way that respects data subjects’ privacy and their well-being? Do dataset creators have the appropriate rights to collect recordings? Under what conditions (e.g., noise) are they collecting the data?
(4) Preprocessing/cleaning/labeling
How are annotators trained to perform the labeling of the data? How familiar are they with the types of speech (e.g., dialect, accent) collected, and how are they resolving differences across annotators? What is the transcription convention used, and how are recordings standardized?
(5) Uses / Distribution / Maintenance
How is sensitive information redacted from the recordings and transcriptions? Is the dataset a subsample of the data collected? What other documentation is available to understand further the data collection process (e.g., agreements signed with data subjects and research methodology)?
Between the lines
Our augmented datasheets provide a blueprint for considerations practitioners should examine and address.
For dataset creators, the datasheets ensure consistency in documenting datasets, increase transparency regarding dataset contents, clarify the motivations and methods behind data collection, and encourage explicit consideration of linguistic subgroups and socioeconomic/demographic categories that are often overlooked.
For dataset users, the benefits include a more comprehensive understanding of dataset usefulness and easier decision-making when selecting data for more robust and inclusive SLTs.
Regardless of whether the datasheet questions are being answered by users or creators, engaging with each question can provide valuable reflexive knowledge on ethical machine learning development. We recommend that dataset creators release a completed augmented data sheet alongside their speech dataset to inform the wider research community about its capabilities and limitations. This release also sets an example for adopting more ethical, inclusive, and transparent machine learning practices.