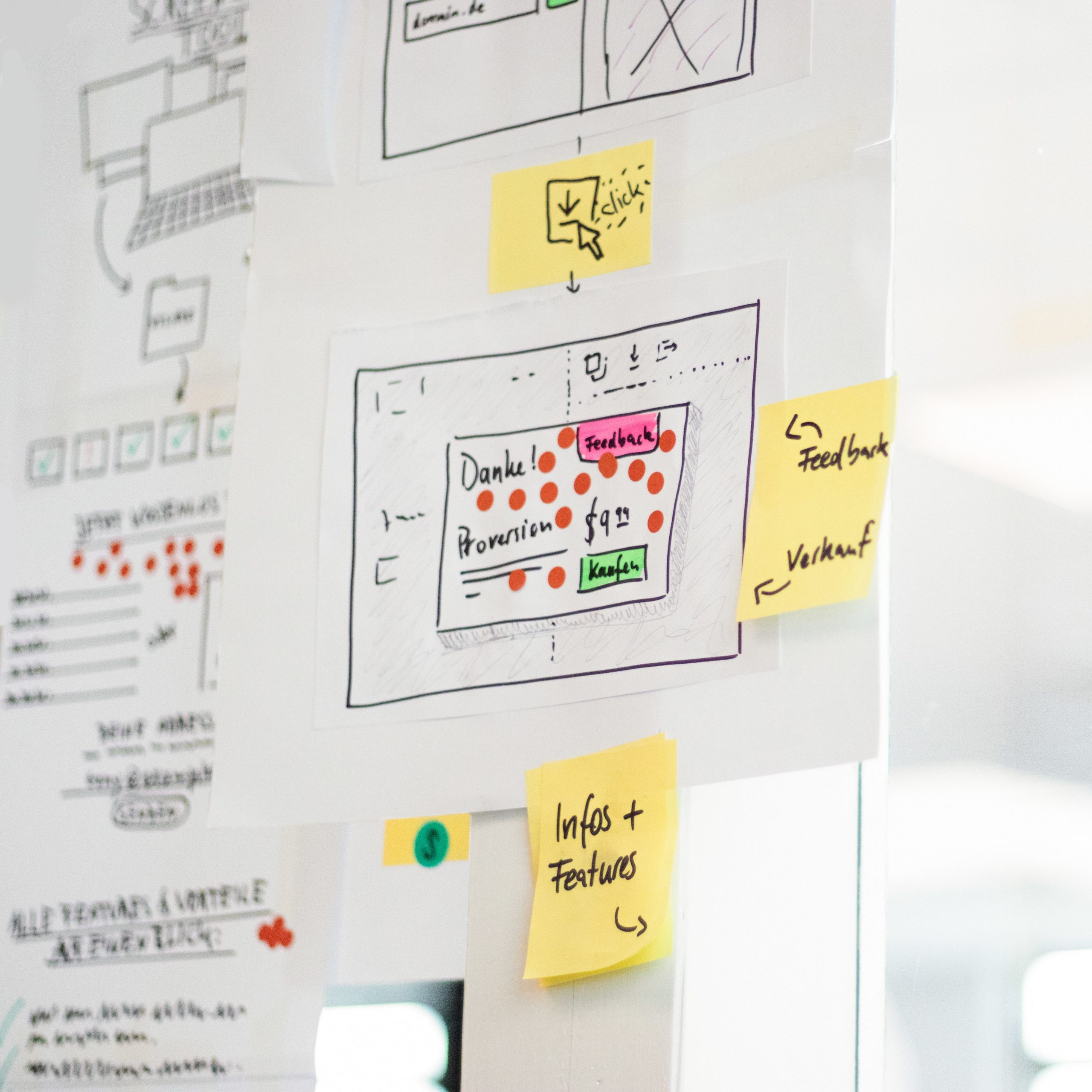
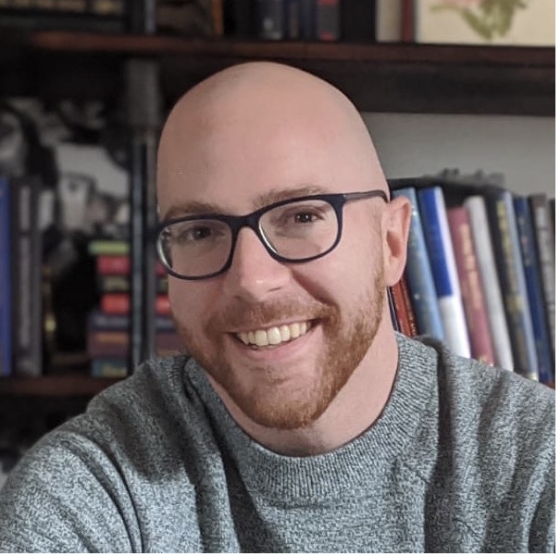
🔬 Research Summary by Michael A. Madaio, a postdoctoral researcher at Microsoft Research, where his research is at the intersection of HCI and FATE (Fairness, Accountability, Transparency, and Ethics) in AI.
[Original paper by Michael Madaio, Lisa Egede, Hariharan Subramonyam, Jennifer Wortman Vaughan, Hanna Wallach]
Overview: Various tools and processes have been developed to support AI practitioners in identifying, assessing, and mitigating fairness-related harms caused by AI systems. However, prior research has highlighted gaps between the intended design of such resources and their use within particular social contexts, including the role that organizational factors play in shaping fairness work. This paper explores how AI teams use one such process—disaggregated evaluations—to assess fairness-related harms in their own AI systems. We identify AI practitioners’ processes, challenges, and needs for support when designing disaggregated evaluations to uncover performance disparities between demographic groups.
Introduction
It is increasingly clear that AI systems can perform differently for different groups of people, often performing worse for groups that are already marginalized in society. Disaggregated evaluations are intended to uncover performance disparities by assessing and reporting performance separately for different demographic groups. This approach can be seen in the Gender Shades project, which found disparities in the performance of commercially available gender classifiers, and in work from the U.S. National Institute of Standards and Technology on performance disparities in face-based AI systems.
However, prior work on the fairness of AI systems suggests that practitioners may have difficulty adapting tools and processes for their own systems, and that tools and processes that do not align with practitioners’ workflows and organizational incentives may not be used as intended, or even used at all.
In this paper, we therefore ask:
- RQ1: What are practitioners’ existing processes and challenges when designing disaggregated evaluations of their AI systems?
- RQ2: What organizational support do practitioners need when designing disaggregated evaluations, and how do they communicate those needs to their leadership?
- RQ3: How are practitioners’ processes, challenges, and needs for support impacted by their organizational contexts?
In the following sections, we discuss several key findings from semi-structured interviews and structured workshops that we conducted with thirty-three practitioners from ten teams responsible for developing AI products and services at three technology companies (you can find more details about our methods and findings in our CSCW’22 paper).
Key Insights
Challenges when designing disaggregated evaluations
We find that practitioners face challenges when designing disaggregated evaluations of AI systems, including challenges choosing performance metrics, identifying the most relevant direct stakeholders and demographic groups on which to focus (due to a lack of engagement with direct stakeholders or domain experts), and collecting datasets with which to conduct disaggregated evaluations.
Challenges when choosing performance metrics
For some teams, choosing performance metrics for disaggregated evaluations was straightforward because they used the same performance metrics that they already used to assess the aggregate performance of their AI systems. Some teams noted that there were standard performance metrics for their type of AI systems (e.g., word error rate for speech-to-text systems), making their decisions relatively easy.
However, most teams did not have standard performance metrics, and some teams felt their typical performance metrics were inappropriate to use when assessing the fairness of their AI systems. Choosing performance metrics for disaggregated evaluations was therefore a non-trivial task, requiring lengthy discussions during the workshop sessions about what good performance meant for their AI systems, how aggregate performance was typically assessed, and whether or how this should change for disaggregated evaluations.
More generally, we find that decisions about performance metrics are shaped by business imperatives that prioritize some stakeholders (e.g., customers) over others (e.g., marginalized groups). Participants described how tensions that arose during discussions about choosing performance metrics—even for teams that had standard performance metrics—were often indicative of deeper disagreements among stakeholders about their values and the goals of their AI systems.
Challenges when identifying direct stakeholders and demographic groups
Participants wanted to identify the direct stakeholders and demographic groups that might be most at risk of experiencing poor performance, and they wanted to do so by engaging with direct stakeholders and domain experts to better understand what marginalization means for different demographic groups in the geographic contexts where their AI systems were deployed.
However, participants described how their typical development practices usually only involved customers and users, and not other direct stakeholders who may be affected by their systems. Moreover, many teams only engaged with users when requesting feedback on their AI systems (e.g., via user testing) instead of engaging with users to inform their understanding of what marginalization means for different demographic groups in a given context or to inform other decisions made when designing disaggregated evaluations. In the absence of processes for engaging with direct stakeholders or domain experts, participants described drawing on the personal experiences and identities represented on their teams to identify the most relevant direct stakeholders and demographic groups on which to focus. However, this approach is problematic given the homogeneous demographics of many AI teams.
Assessment priorities that compound existing inequities
During the workshop sessions, each team identified many more direct stakeholders than could be the focus of a single disaggregated evaluation (particularly given the variety of the use cases and deployment contexts that they wanted to consider). As a result, participants discussed how they would prioritize direct stakeholders and demographic groups. Their priorities were based on the perceived severities of fairness-related harms, the perceived ease of data collection or of mitigating performance disparities, the perceived public relations (PR) or brand impacts of fairness-related harms on their organizations, and the needs of customers or markets—all heuristics that may compound existing inequities.
For instance, teams wanted to prioritize direct stakeholders and demographic groups based on the severities of fairness-related harms, but they found it difficult to understand whether or how performance disparities might translate to quantifiable harms, especially without engagement with direct stakeholders. In addition, many teams discussed prioritizing based on the PR impacts of fairness-related harms, asking what it would mean for their organization if particular direct stakeholders or demographic groups were found to experience poor performance, envisioning specific types of harms as “headlines in a newspaper.” However, this approach of making prioritization decisions based on brand impacts is a tactic that may ignore concerns about direct stakeholders’ experiences in order to uphold an organization’s image. In addition, these approaches are inherently backward looking, as they focus on performance disparities that are uncovered after AI systems have been deployed. In contrast, reflective design approaches encourage practitioners to reflect on values and impacts during the development lifecycle.
Finally, teams described prioritizing direct stakeholders and demographic groups based on the needs of customers and markets, thereby shaping disaggregated evaluations in ways that may compound existing inequities by reifying the social structures that led to performance disparities in the first place. For instance, prioritizing customers may lead to a focus on members of demographic groups that are already over-represented and privileged in the geographic contexts in which a given AI system is deployed. In addition, prioritizing direct stakeholders and demographic groups using “strategic market tiers,” although already widely used to prioritize product deployment to new geographic contexts, may reinforce existing inequities across geographic contexts.
Needs for organizational support
Teams wanted their organizations to provide guidance about and resources for designing and conducting disaggregated evaluations. In particular, participants wanted guidance on identifying the most relevant direct stakeholders and demographic groups on which to focus, and guidance on the types of fairness-related harms that might be caused by their AI systems. Teams also wanted organization-wide strategies for collecting datasets with which to conduct disaggregated evaluations. Participants felt that their organizations should help teams understand how to collect demographic data in ways that might allow them to adhere to their privacy requirements, addressing challenges at an organizational level, rather than at a team level.
However, organization-wide strategies for collecting datasets (and for identifying relevant direct stakeholders and demographic groups) might be difficult to establish given the diversity of AI systems, use cases, and deployment contexts. Moreover, the economies of scale that motivate the deployment of AI systems to new geographic contexts based on strategic market tiers may lead to homogenized understandings of demographic groups that may not be reflective of all geographic contexts.
In addition, participants described needing to advocate for resources (e.g., money, time, personnel) for designing and conducting disaggregated evaluations. Despite organizations’ stated fairness principles and practitioners’ best intentions when designing disaggregated evaluations, the reality of budgets for collecting datasets, as well as budgets for other activities (such as engaging with direct stakeholders and domain experts) constrain what teams are able to achieve. Participants repeatedly told us that their organizations’ business imperatives dictated the resources available for their fairness work, and that resources were made available only when business imperatives aligned with the need for disaggregated evaluations.
Implications for assessing fairness of AI systems
Our findings raise questions about the ways in which business imperatives impact disaggregated evaluations, in turn leading to negative consequences for the people most likely to experience poor performance. Indeed, such priorities should make us skeptical that organization-wide guidance on identifying direct stakeholders and demographic groups or organization-wide strategies for collecting datasets will actually reflect the needs of marginalized groups.
These findings also suggest that the scale at which AI systems are deployed may impact disaggregated evaluations due to a lack of situated knowledge of what marginalization means in different geographic contexts. Many of our participants reported challenges relating to the scale at which AI systems are deployed. Participants on every team shared that they felt pressured to expand deployment to new geographic contexts, and we saw the impact of these pressures on nearly every decision made when designing disaggregated evaluations. Our findings reveal implications of this tension, as many participants reported deploying AI systems in geographic contexts for which they have no processes for engaging with direct stakeholders or domain experts. Without such processes, practitioners draw on their personal experiences and identities, their perceptions about fairness-related harms (including the perceived severities of those harms), and even their own data—all workarounds that may perpetuate existing structures of marginalization.
Between the lines
This study contributes to the growing literature on practitioners’ needs when operationalizing fairness in the context of AI system development, including the role that organizational factors play in shaping fairness work. As researchers develop new tools and processes for identifying, assessing, and mitigating fairness-related harms caused by AI systems, it is critical to understand how they might be adopted in practice. Our findings suggest the need for processes for engaging with direct stakeholders and domain experts prior to deployment to new geographic contexts, as well as counterbalances to business imperatives that can lead to pressures to deploy AI systems before assessing their fairness in contextually appropriate ways.