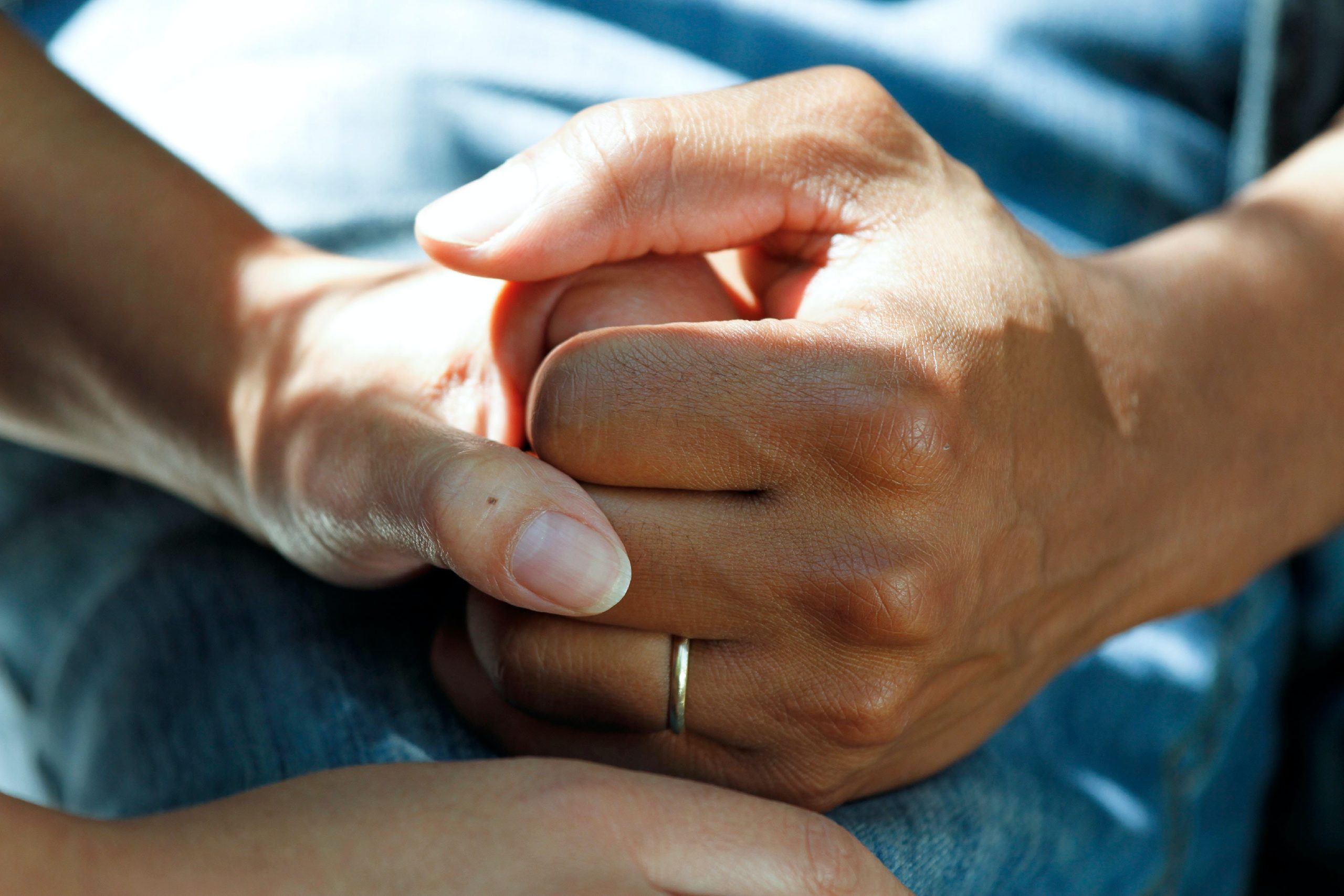
🔬 Original article by Nils Aoun, Chloé Currie, Itai Epstein, and Chaaron Nahar from Encode Justice Canada
This is a part of our Recess series in which university students from across Canada briefly explain key concepts in AI that young people should know about: specifically, what AI does, how it works, and what it means for you. The writers are members of Encode Justice Canada, a student-led advocacy organization dedicated to including Canadian youth in the essential conversations about the future of AI.
Introduction
Chloé Currie
Artificial intelligence (AI) has been introduced into a number of diverse industries that are integral to society. Healthcare, in particular, has benefited from the use of AI as the technology has been used to complete more mundane tasks, such as automating personal medical histories and diagnosis through medical imaging. [1] These tasks, which previously diverted experts’ attention from medical emergencies, highlight the constructive ways in which AI has been used in our society. Although AI in healthcare can present certain benefits, there are ethical implications to the use of machine learning, particularly regarding the lack of transparency and patient-doctor connection that AI represents. As AI becomes more prevalent within healthcare, these ethical dilemmas must be combatted to ensure the integrity of the industry.
The Use of Artificial Intelligence in Healthcare
Chaaron Nahar
AI has gained momentum in several industries, including healthcare. Although there is some research on the promising ways AI can be incorporated into medicine, current applications of AI in healthcare already exist.
One of the ways AI is being used in the healthcare system is to improve the diagnosis process, making it more efficient and able to recognize a pattern of symptoms earlier. An example of such AI programs comes from the Houston Methodist Research Institute, which “reliably interprets mammograms and translates patient data into diagnostic information 30 times faster than a human doctor, with 99 percent accuracy.” [2] Though often overlooked, the implementation of AI at the diagnostic stage is not without reason. According to the American Cancer Society, half of the mammograms performed in the US yield false positives. [3] Therefore, “one in two healthy women [are] told they have cancer.” [4]
AI algorithms can remove the fear of false diagnoses, reducing health complications and collateral costs for all parties involved. AI models are not only being used to decipher imagery-based results (e.g. CT and MRI scans). Instead, machine learning algorithms also accurately evaluate laboratory results such as “blood and biochemical examination records” at the Shengjing Hospital of the China Medical University. [5] With the increasingly expanded range of data recognition, AI and ML models can also help doctors make informed decisions regarding more uncommon illnesses such as Multiple Myeloma, as was done at the China Medical University. [6]
Another example of AI in healthcare is its presence in consumer direct-to-consumer (DTC) health applications. According to Millenson et al., such applications are used as an at-home substitute for medical services ranging from symptom checking via “sensor-driven” image processing (therefore, bringing the user closer to a self-diagnosis) to recommending preventive measures via interactive ML algorithms. [7] When used for self-diagnosis, DTC healthcare apps are most commonly developed for dermatological issues, followed by triage and general diagnosis of various health conditions. These services, now easily accessible through our phones, can either offer accurate medical suggestions to improve diagnosis or incorrectly generated answers to users’ questions. They are not heavily regulated by governmental agencies such as the Food and Drug Administration (FDA), with “the current FDA commissioner [stating] that apps helping consumers self-diagnose are an innovation that regulations should not impede.” [8]
Besides diagnostic apps, Millenson et al. maintain that “health management” applications focusing on preventive medicine receive more attention in the market. A quick glance at the Apple App Store or Google Play will show that applications promoting a healthier lifestyle remain popular among consumers. For these applications, AI models may gather data from the user regarding their health habits – including one’s sleeping patterns, menstrual cycle, or physical activity – and make predictions on optimal courses of action for the user moving forward.
With more practice, these innovative applications of AI may become more common in hospitals worldwide and encourage the medical industry to use AI to its advantage when helping patients stay healthy.
The Benefits of AI in Medicine
Itai Epstein
Research in medicine by people is at a plateau. For years, changes in medicinal practices have been stagnant. Doctors and medical researchers work for countless hours on research and development to find the next ground-breaking cure or solution. Even if found, the regulation and approval process is often gruesomely long due to the potentially harmful effects of medicine on humans. However, many breakthroughs have occurred through AI, solving problems that have yet to be answered by the medical community. AI brings numerous benefits, but there is skepticism surrounding the use of AI in healthcare as it is often used as part of the critical decision-making process.
AI is already used in many aspects of the medical field. For example, AI is used to speed up the process of connecting patients to doctors, schedule appointments online and digitize health records, and manage medical data. In addition, use of AI gives doctors more time to focus on helping their patients directly.
The main benefit computers have over humans is completing tasks with extreme speed and efficiency. This is particularly helpful in medical applications, where large amounts of data need to be processed. As said by Robert Truog, the current Director of the Center for Bioethics at Harvard University, “according to psychologists, humans can only handle four independent variables and when we get to five, we are lost. Therefore, AI is coming at the perfect time. It can potentially rescue us from data overload,” as the amount of data used by some doctors has risen tenfold over the past few years. [9] Consequently, digitization frees up time for doctors who are otherwise bogged down by the more tedious aspects required of a medical field.
Traditional AI systems are deployed under a technique called supervised learning, in which the system is given an example of what it is trying to classify. Implementing this approach for AI-assisted medical diagnosis has shown tremendous success. For example, an algorithm developed by Google AI Healthcare, LYNA, which specializes in identifying breast cancer tumours from lymph node biopsies, was shown to correctly identify a sample as cancerous or not cancerous 99% of the time. [10] Similarly, the DLAD algorithm, developed by researchers at Seoul National University Hospital and College of Medicine, specializes in analyzing chest radiographs for abnormal cell growth. When comparing its performance to doctors, it outperformed 17 of 18 participants. [11] Generally, for a properly developed and trained AI system, the accuracy is above 90%. AI can be used jointly with doctors in the decision-making process, helping them perform better at their job and helping their patients. [12]
Several AI systems excel at classifying diseases earlier than doctors in various medical specialties. [13] These include predicting heart disease, lung diseases, kidney diseases, and seizure detection. [14] Detecting diseases early is crucial to saving a patient’s life, ensuring they get the proper treatment necessary.
Health professionals can use AI systems in medicine to solve previously unsolvable problems. AI systems have the potential to advance the understanding of fundamental scientific problems. One notable example is Deepmind’s AlphaFold algorithm, which predicts protein structures with high accuracy. Before this breakthrough, the problem of predicting how proteins fold and determining their actions had been worked on for almost 50 years, where its solution remained a mystery.
While the authorization of new medical practices is often slow, the medical community is already reaping the benefits of using AI in existing diagnoses. AI has primarily increased the efficiency of the diagnosis process. Showing great accuracy in classifying imaging from scans of specific diseases, helping save many lives. Now, AI systems are used to solve problems previously thought unsolvable. While not permitted for treatment use, AI is rapidly applied to every other aspect of the medical community. The promises of AI-based personal treatments and AI-assisted surgeries have the potential to transform the field of medicine as we know it. [15] However, we have yet to see and experience the true potential that AI in medicine will bring.
The Ethical Implications of AI Use in Medicine
Nils Aoun
In the last decade, AI has increased in various fields, including medicine. While it does facilitate and accelerate medical processes and help locate health issues more efficiently, AI creates some ethical problems that must be addressed before becoming more widespread.
Machine-learning and AI can have tremendous benefits for a patient’s health, and can be used to treat medical conditions. [16] However, the full benefits of using AI to predict and act cannot be realized without considering the potential ethical issues. For example, researchers have “begun to notice, from implementations in unhealthy care areas, that algorithmic learning can have ethical problems when deployed at a large scale.” [17] Current policy and ethical guidelines for AI technology are falling behind the technological progress AI has made in the healthcare field.
In a perspective piece published March 15 in The New England Journal of Medicine, researchers raised four main concerns:
- Data used to create algorithms can be biased for various reasons; this bias is then reflected in the algorithm, predictions, and recommendations resulting from the analysis. Skewed results are not optimal, yet algorithms might be designed with this intent depending on the motives behind their creation.
- Physicians should understand how algorithms are created as well as the statistical models that predict outcomes. This would allow the physician to have some agency and not simply accept results while becoming dependent on these algorithms; the data should not become the doctor.
- The human aspect of patient care, as well as clinical experience, cannot be disregarded. The datafication of patient health, diagnostics and outcomes does not account for these factors.
- Machine learning-based clinical guidance can introduce a third-party actor, which comes between the physician and the patient. This new middleman challenges the dynamics of responsibility and expectations regarding confidentiality. Ultimately, a machine can look at data but cannot evaluate the output critically. While AI enables efficiency improvements in healthcare delivery, it poses privacy, confidentiality, consent, and patient agency and autonomy problems. [18]
These concerns have prompted the World Health Organization (WHO) to list ethical principles they believe should be at the forefront of AI in healthcare. The WHO values autonomy and believes humans should have “oversight of and the final say on all health decisions.” [19] As mentioned previously, machines should be used by doctors – not replace them. It believes that developers should proactively monitor AI tools to ensure they work properly. Transparency is another issue at the heart of the WHO’s principles. Doctors and other practitioners should understand how algorithms’ decision-making process works to know when to intervene. With machines, accountability becomes an issue, whether related to malfunctioning or coded bias leading to unwanted results. Therefore, there should be mechanisms to trace back responsibility to the manufacturers, doctors, or other entities involved in the decision-making process of medical procedures. Lastly, the WHO promotes the sustainable use of AI, in a way that allows for its long-term use and modification when needed in the healthcare industry.
Overall, AI provides great use cases in healthcare and benefits both patients and practitioners. However, taking advantage of these benefits, things can go wrong. To limit the flawed use of AI and the potential harms it could lead to, programmers and doctors alike need to be conscious of its limitations and adapt their approaches accordingly. In addition, as its use is becoming more prominent in healthcare, professionals are pointing out key ethical principles that should be respected in practice.
Conclusion
Chloé Currie
AI can be used to create ease within our everyday lives. The automation of specific aspects of the healthcare industry have allowed for doctors to focus on patients directly without worrying about the tedious tasks involved in administration. Although the introduction of AI has benefited some aspects of the healthcare sector, its full implementation continues to be a topic of debate. As AI becomes more ingrained in society, so do the fears regarding its ethical implications. With regards to the use of AI in healthcare, these ethical implications could cause detrimental harm to people’s physical wellbeing. In order to ensure the safe introduction of more AI and machine-learning mechanisms in the healthcare industry, increased accountability for how these machines are employed is necessary to ensure the ethical use of the technology.
Notes
[1] Bohr, A. & Memarzadeh, K. (2020, June 26). The rise of artificial intelligence in healthcare applications. In A. Bohr & K. Memarzadeh (Eds.), Artificial Intelligence in Healthcare (pp. 25–60). chapter, Academic Press.
[2] Griffiths, S. (2016, August 26). This AI software can tell if you’re at risk from cancer before symptoms appear. WIRED UK. Retrieved March 14, 2022, from https://www.wired.co.uk/article/cancer-risk-ai-mammograms.
[3] Ibid.
[4] Ibid.
[5] Yan, W., Shi, H., He, T., Chen, J., Wang, C., Liao, A., Yang, W., & Wang, H. (2021). Employment of artificial intelligence based on routine laboratory results for the early diagnosis of multiple myeloma. Frontiers in Oncology, 11. https://doi.org/10.3389/fonc.2021.608191.
[6] Ibid.
[7] Millenson, M., Baldwin, J., Zipperer, L. & Singh, H. (2018). Beyond Dr. Google: the evidence on consumer-facing digital tools for diagnosis. Diagnosis, 5(3), 95-105. https://doi.org/10.1515/dx-2018-0009.
[8] Ibid.
[9] Powell, A. (2020, December 4). Risks and Benefits of an AI Revolution in Medicine. Harvard Gazette. Retrieved March 15, 2022, from https://news.harvard.edu/gazette/story/2020/11/risks-and-benefits-of-an-ai-revolution-in-medicine/.
[10] Powell, A. (2019, June 19). Artificial Intelligence in Medicine: Applications, Implications, and Limitations. Science in the News. Retrieved March 14, 2022, from https://sitn.hms.harvard.edu/flash/2019/artificial-intelligence-in-medicine-applications-implications-and-limitations/.
[11] Ibid.
[12] Powell, A. (2020, December 4). Risks and Benefits of an AI Revolution in Medicine. Harvard Gazette. Retrieved March 15, 2022, from https://news.harvard.edu/gazette/story/2020/11/risks-and-benefits-of-an-ai-revolution-in-medicine/.
[13] Greenfield, D. (2020, March 9). How AI Technologies Accelerate Progress in Medical Diagnosis. Robotics & Automation News. Retrieved March 15, 2022, from https://roboticsandautomationnews.com/2020/03/09/how-ai-technologies-accelerate-progress-in-medical-diagnosis/31184/.
[14] Briganti, G. & Le Moine, O. (2020, February 5). Artificial Intelligence in Medicine: Today and Tomorrow. Frontiers. Retrieved March 15, 2022, from https://www.frontiersin.org/articles/10.3389/fmed.2020.00027/full.
[15] Powell, A. (2019, June 19). Artificial Intelligence in Medicine: Applications, Implications, and Limitations. Science in the News. Retrieved March 14, 2022, from https://sitn.hms.harvard.edu/flash/2019/artificial-intelligence-in-medicine-applications-implications-and-limitations/.
[16] Esteva A., Kuprel B., Novoa R.A. (2017). Dermatologist-level classification of skin cancer with deep neural networks, Nature 542(7639), 115-118.
[17] Hannon, P. (2018, March 14). Researchers say use of artificial intelligence in medicine raises ethical questions. Stanford Medicine. Retrieved March 7, 2022, from https://stan.md/3CxTSXB.
[18] Rigby, M.J. (2019, February). Ethical dimensions of Using Artificial Intelligence in Health Care. AMA Journal of Ethics. Retrieved March 7, 2022, from https://bit.ly/3HWcbqr.[19] Wetsman, N. (2019, June 30). WHO outlines principles for ethics in health AI. The Verge. Retrieved March 7, 2022, from https://bit.ly/3hVj8xv.