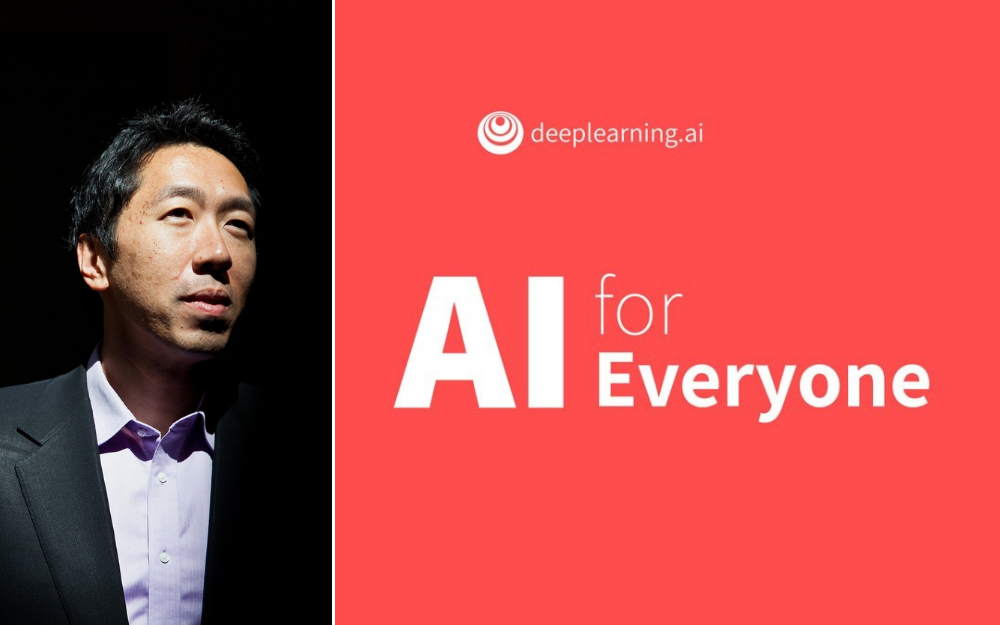
At the end of every AI event this year, a common question from audiences has been “What can I do to get into AI”?
People want to use artificial intelligence as a lever to boost their career success and create more value in the world. Up until now, our best answer has been “read everything you can, and discuss your strongest ideas at AI-related meetups”. It’s an answer that’s vague, but probably familiar to you.
The truth is, nobody had done the hard work of deconstructing AI and carefully curating the small handful of AI mental models that beginners need to master — at least, until Andrew Ng and his team at deeplearning.ai created their new course AI for Everyone on Coursera.
“Congratulations on finishing this course. You’re now significantly ahead of many large companies’ CEOs in your understanding of AI and your ability to plan for the rise of AI.”
– Andrew Ng (AI For Everyone)
Those are Ng’s parting remarks at the conclusion of the course. It’s a bold statement — to suggest that a 7 hour course will leave you more knowledgeable about AI than most CEOs. But this is from the man that
coined the phrase “AI is the new electricity”, in his talk at Stanford’s Graduate School of Business. The reason that he can make that statement confidently is because of the work he and his team have put in. The truth is that Ng is uniquely qualified to create this AI 101 course.
He has invested a significant amount of time in thinking about how to effectively facilitate a culture of lifelong learning – that’s why he founded Coursera. He has also specifically invested time in doing AI education right – that’s why his Machine Learning course on Coursera (offered by Stanford) has a 4.9 star rating, and more than 24 000 reviews.
And that expertise shows in the organization and presentation of the content within the course. The selection of topics covered within each module are tangible: the principles are clear, the examples are concrete, and he doesn’t shy away from simplifying ideas to effectively communicate them. For example, when explaining how deep learning is used for facial recognition, he used visuals to intuitively describe how each layer of a neural network is responsible for detecting increasingly complex patterns: edges in the first, parts of a face in the second, and face shapes in the third.
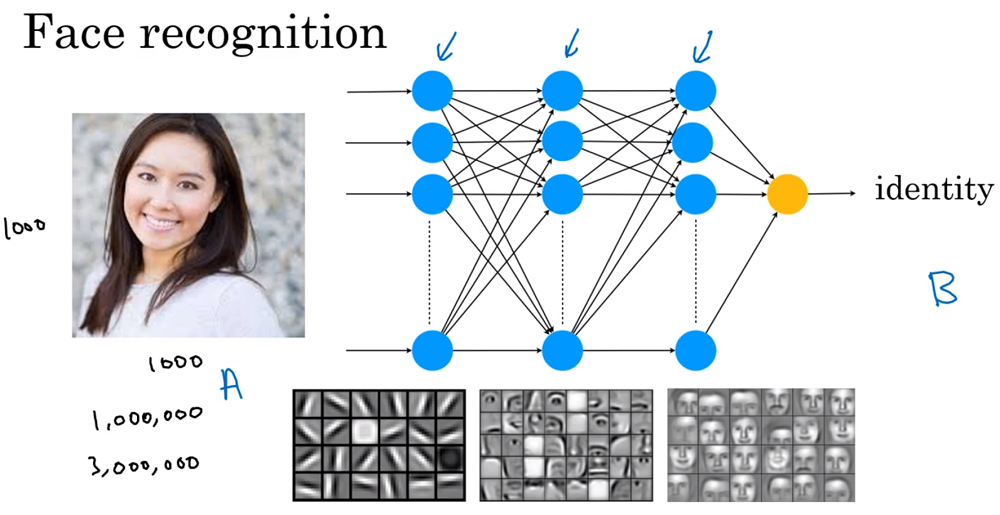
It’s quite refreshing to see Andrew Ng take the reins and lead by example by dedicating 25% of the course towards discussing the societal implications of AI, including AI ethics – something that’s notably missing from most AI curriculums. In the same vein, we commend the accessibility to the course through Coursera’s financial aid for those who need it – it truly is AI For Everyone.
Practically speaking, AI For Everyone is a 7-hour video course with a multiple-choice quiz at the end of each module allowing you to test your knowledge. Some modules have optional deep dives into side-quests: like surveys of major AI techniques and application areas. There are 4 modules:
- What is AI?
- Building AI projects
- Building AI in your company
- AI and Society
Below is a high-level preview of each module: use it as a pre-read to prep for the course, or to decide whether you want to do it in the first place.
Module 1: What is AI
Ng explains that AI will be creating additional $13T of value per year by 2030, and will transform every sector in the economy – we’d better pay attention. At the heart of AI implementation right now is machine learning – having a system learn A-B mappings using data: in other words, taking in data and outputting a result. Data is information that can be presented in structured formats (think spreadsheets) or unstructured formats like audio, video, and text. The more data you have at your disposal, the more powerful the algorithms will become. And data can also be used for data science – distinct from machine learning and primarily used to uncover insights to improve decision-making.
The reason for the surge in artificial intelligence is due to relatively recent advances in deep learning – a subset of machine learning that uses multiple layers of interconnected “neurons” (inspired by how brains work) to create outputs in much more sophisticated ways than what was previously feasible. Ng proposes a simple heuristic to judge whether or not an AI project is technically feasible: is it something that humans can do in 1 second of thought? For example, look at an image of a car and tell how far away it is – a crucial task in self-driving vehicles. He also touches on the weaknesses of AI in its current state: it’s data-hungry and doesn’t work well in complex tasks requiring integration of information across different domains.
Module 2: Building AI Projects
Here’s the series of steps that all machine learning projects go through: First, you gather data. Then you train the algorithm using the data you collected. Finally, you deploy the algorithm in the real world to learn more so you can improve it again. Data science is slightly different: you gather data, extract important insights, and then create a report that can help a business leader improve their managerial decision-making. Specific examples of each are provided in the course.
Ng also touches on the important Venn diagram you need to think about when strategizing about which AI project you want to build. Any worthy AI project should be at the intersection of what’s technically feasible, and what brings value to the business or society. This requires diligent research before jumping into a project – it’s better to spend a few hours thinking about whether something’s worth pursuing, than to spend 100 hours building something that isn’t possible or useful for anyone. A guiding principle he provides is “think about automating tasks, not jobs” – the strongest value-add for AI right now is automating routine tasks to free up people to work on tasks that require strategy, creativity, and social skills. This is similar in spirit to what Kai-Fu Lee suggests in his book AI Superpowers.
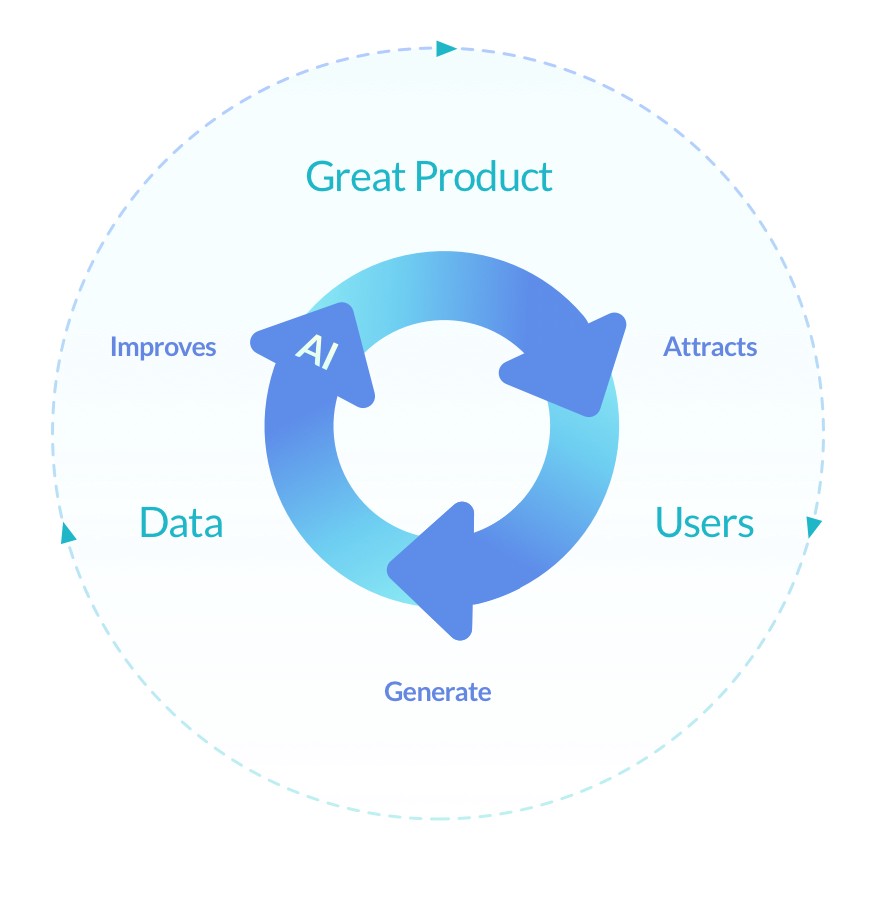
Module 3: Building AI in your Company
Ng details several case studies including smart speakers and self-driving cars: he does a live breakdown of what the project would entail and where machine learning would fit. This leads into Ng’s famous AI Transformation Playbook – a recipe for startups and large companies alike to level up with AI.
The playbook is composed of 5 steps:
- Execute a pilot project to gain momentum: Whether it’s in-house or outsourced, build a small AI project within 6-12 months to get a feel for AI-specific projects. Lean towards technical feasibility instead of what’s most valuable for a business in this initial stage.
- Build an in-house AI team: Begin by recruiting a strong AI team leader who can work cross-functionally to help other departments build AI tools, as well as to build platforms that can be used company-wide.
- Provide broad AI training: Different positions should focus their learning efforts on the most relevant aspects that will help them do their job better. (See table below)
- Develop an AI strategy: Use the virtuous cycle of AI (better product leads to more customers leads to more data leads to better product) to create an industry-specific advantage that you are uniquely positioned to defend against new entrants on the market.
- Communicate: you have a responsibility to your stakeholders, customers, and team to transparently update them on your progress.
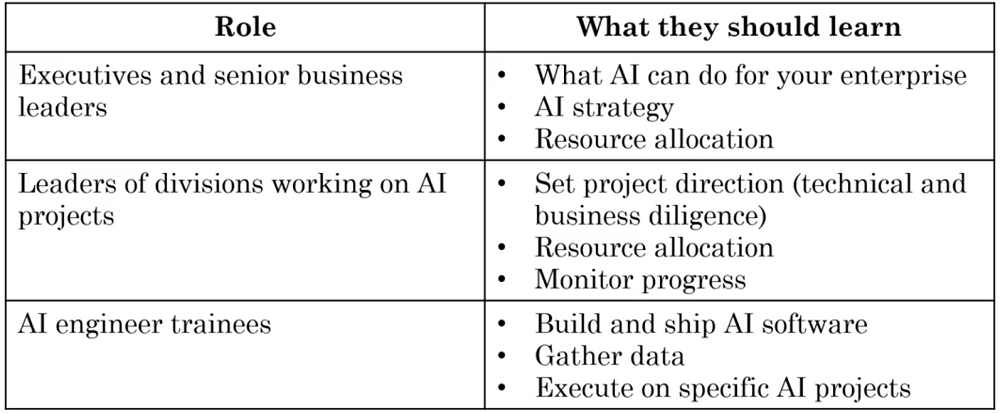
Week 4: AI and Society
A discussion of a technology that can disrupt industries, change judicial decisions, and decide whether or not someone gets a bank loan, is incomplete without talking about ethics. Andrew deeply understands that, because of his deep involvement within the industry at Google Brain, Baidu, and deeplearning.ai. As Andrew says himself in the course, “The topic of AI and Ethics deserves its own 4 week or much longer course”.
The biggest issues he outlines are: discrimination, adversarial attacks on AI systems, adverse uses of AI, impacts on developing economies, and sector mobility within the labor market.
He points out that while combating biased AI systems is difficult, some technical solutions exist: zero’ing out label biases, using inclusive data sets representative of the population, building transparent and auditable systems, and ensuring diversity within the teams working on AI projects. An intriguing point that’s raised is that we have more tools at our disposal for combating AI bias than we do for combating human bias.
While adversarial attacks on AI are inevitable, Ng predicts that this arms race is a different one from those we’ve seen before because most of the economic resources in AI right now are being used to build tools deemed useful by society. Society works better when there is less fraud, for example, because of lowered transaction costs and information asymmetries. As such, not many resources will go into building tools that enable fraud. The barrier to entry and weak incentives may create enough friction to discourage people from building powerful adversarial tools.
For developing economies, Ng acknowledges that low-end manufacturing jobs and agricultural work will quickly be automated. Their strongest tools here will be: leveraging leapfrog opportunities (like China did with mobile payments), focusing on AI to amplify the economy’s uniquely powerful industry verticals (instead of competing with Google), and working with the public sector to invest in a more educated citizen base. Then they can strive towards work that contributes more to the economy and that has uniquely human requirements.
On labor impacts, Ng quickly points out McKinsey’s study predicting that by 2030, more jobs will be created than lost to AI by 2030. To deal with the short-term turbulence on the labor market because of imperfect sector mobility, he recommends conditional basic income – providing a financial safety net for those who are upskilling or re-skilling to be able to effectively contribute to society. His skepticism of universal basic income is rooted in the incentive structure: giving everyone enough money to survive can undermine their desire to do meaningful work.
Note that you don’t have to quit your job and learn to code AI to have a job in the future. Ng recommends doing work at the intersection of AI and your existing unique strengths (whether that’s medicine, law, or communications) – this gives you a unique lens on the world that will prove immensely valuable to employers and society. This requires you to become a lifelong learner, and to equip the mindset that you’re working with AI, not against it.