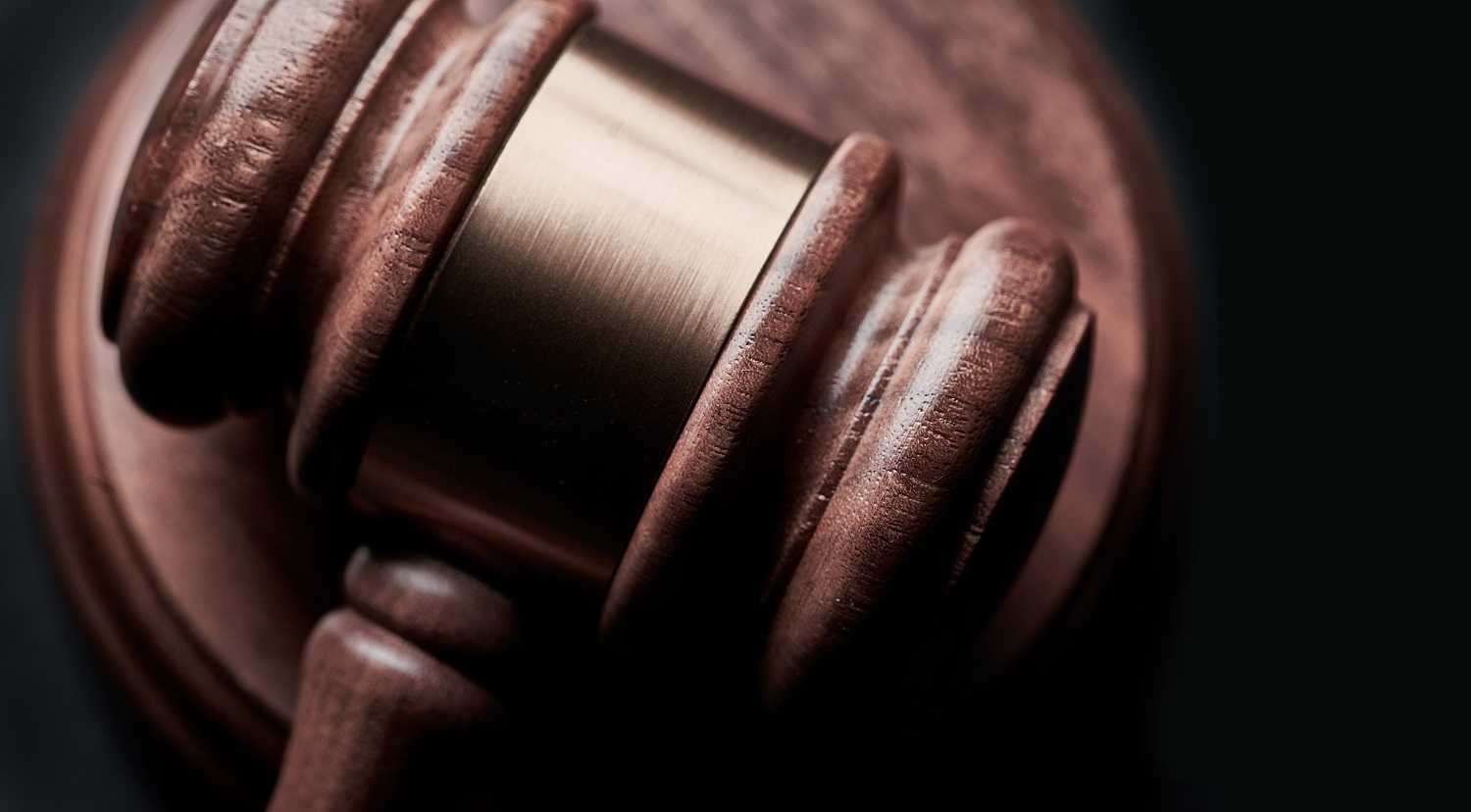
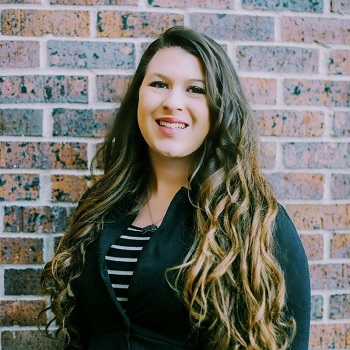
Paper contributed by Brooke Criswell (@Brooke_Criswell). She’s pursuing a PhD. in media psychology, and has extensive experience in marketing & communications.
Artificial intelligence (AI) is everywhere and in every industry. Technological advances can enhance people’s everyday lives and produce some amazing outcomes at rapid speed. However, AI also has the potential to be biased and harm individuals, depending on the algorithms’ usage and design. Many industries including the judicial system are now incorporating AI into their decision making. The claim is that using machines takes the biases that humans have, out of the equation, and so the decisions have to be objective. However, it has been shown time and time again that it is not true (O’Neil, 2016). This paper explores how artificial intelligence is being used in the court room for predicting criminal behavior, length of sentences, and determining who is likely to recommit a crime. Data scientists are also being hired within the judicial system to manage these machines; however, media psychologists are better fit and need to be involved in the process. Data scientists are not trained in human cognition and human behavior. In fact, before algorithmic techniques, the risk was assessed clinically by psychologists (Agrawal et al., 2019).
The legal system cannot rely solely on artificial intelligence because the machines do not understand the entire context or situations in the society like a human trained in law does. New technology should be used but with the right people interpreting the data and understanding the meaning. AI should be used as a tool for probability to help guide decisions but not make the final decisions that define people’s life.
Artificial Intelligence
Artificial intelligence has been around since the 1950s. In 1956, the idea of a machine that could imitate human reasoning was given its name AI and implemented by John McCarthy (Childs, 2011). McCarthy tried to develop a language able to translate human reasoning through computer instructions and worked at MIT.
AI has come a long way from then. The world runs on AI. Now, it is making important decisions that affect the rest of someone’s life. AI is made up of various components, including machine learning, deep learning, and neural networks. In many instances, AI has been compared to the human brain (O’Neil, 2016). These components aim to understand the fundamental principles of learning as a computational process that combines tools from computer science and statistics. Machine learning is explicitly used to find patterns and predict outcomes (Perrot, 2017). Neural networks are based on modeling neurons and feeding a network of training data to find patterns (Perrot, 2017).
Algorithms are built within artificial intelligence to perform a specific task. They can be described as various ways a computational procedure or set of instructions defines and takes a set of values as inputs and produces a set of values as an output (Agrawal et al., 2019).
“Black boxes” has become a term coined for describing machine learning algorithms because they operate in a way that nobody can understand. The algorithms adjust repeatedly and weight inputs differently to improve the accuracy of their predictions and so people have a hard time understanding how and why the algorithms reach the outcomes they do (Deeks, 2019). This has become an issue in understanding decisions made by machines, especially in the judicial system.
The lack of transparency and accountability of predictive models being used in the decision making for determining who will become a criminal, how long someone guilty should be sentenced for, and who is going to recommit a crime has severe consequences (Mckay, 2019). Is it fair for a machine to decide the fate of the future while not explaining why or how the decision was made? This scenario is already happening across America.
Case Study Examples
Risk assessments have become a critical part of the criminal justice system and law enforcement. Case law demonstrates engagement with risk-related terminology, including risk management, risk profile, risk factors, risk behavior, and risk recidivism (Mckay, 2019). Now, AI is being used to gauge predicting risky behavior in human beings. Risk assessments are used at different criminal procedure decision points such as bail, sentencing, and parole (Agrawal et al., 2019).
AI-informed decision-making and prediction happen when algorithms are applied to datasets with tasks that are automated with using neural networks and deep learning to make decisions that the court uses (Agrawal et al., 2019).
A 2016 report by ProPublica showed that the machine learning-based program used in Florida courts had racial biases implemented in them. A woman named Borden was charged with burglary and petty theft for $80 worth of items, and a man named Prater was charged with theft worth $86.35. Borden had misdemeanors on her record as a juvenile while Prater was convicted of armed robbery and attempted armed robbery and served five years in prison for another armed robbery. When both people were inputted into the machine to determine who was higher at risk for recommitting a crime. Borden showed up at a higher risk score of eight than Prate, who was scored at a three. The difference was that Borden is black, and Prater is white. In fact, two years later, the computer algorithm got it backward, and Borden had not been charged with any new crimes, and Prater is serving a new eight-year prison term for stealing thousands of dollars’ worth of electronics (Larson & Angwin, 2016).
ProPublica (2016) went through 7,000 cases and found the score proved remarkably unreliable in forecasting violent crime: Only 20 percent of the people predicted to commit violent crimes actually went on to do so. The researchers also found that the formula was particularly likely to falsely flag black defendants as future criminals, wrongly labeling them this way almost twice the rate as white defendants and white defendants were mislabeled as low risk more often than black defendants (Larson & Angwin, 2016).
The algorithm used to create the Florida risk scores is a product of a for-profit company, Northpointe. Northpointe disagreed with their report and claimed they do not ask about race information. However, in the 137 questions, they ask or pull from criminal and public records, show bias toward people of color. For example, one of the questions is, “was one of your parents ever sent to jail or prison?” In the United States, police lock-up far more people of color than any other country because of stereotypes and personal biases (Larson & Angwin, 2016). More than two million people are incarcerated in the United States, and a disproportionate amount of these individuals are African Americans (Avery, 2019). The future prediction is calculated from the already selected facts about facts by deciding these 137 questions would provide the appropriate outcome that determines people’s life.
Another law case People v. Chubbs (2015) in California, where Billy Ray Johnson was imprisoned based on evidence from software developed by a private company True Allele, refused to reveal how the software worked. The California Appeals Court rules in favor that companies as True Allele were not required to show how they worked and came to their conclusion.
There was another case of Glenn Rodriguez, a prisoner with a nearly perfect record, who was denied parole due to an incorrectly calculated COMPAS score and got a lot of media attention about going through the process where an algorithm defined his fate (Wexler, 2017).
When making decisions based on a score, what message does that send to prisoners who may have already been discriminated against from the institutional system? It reinforces various narratives of stereotypes that add one more hurdle and barrier to equality in society.
Data and Meaning
 Designers of algorithms must decide how much weight is added to a specific value, which includes bias. Who are the computer programmers to decide? Cathy O’Neil (2016) describes in her book that “models are opinions embedded in mathematics and reflect goals and ideology.”Â
Defining the goals and the problems when deciding what training data to collect and how to label the data are also among matters designers use when creating an algorithm (Završnik, 2019). Compiling databases and creating algorithms for prediction always require decisions that are made by humans. Determining how this data is collected, cleaned, and prepared matters.
These algorithms in the legal, judicial system use data from occurrences in the past when people know the system has been stacked against minorities for centuries. The past data does not take into considerations why the data is the way it is. Technology cannot change the future if the machines are already using biased data collected. The world is continuously evolving, and there must be room for people to break the cycle and not be continuously stigmatized under the disguise of technology. Biases must be taken into consideration.
Data does not have meaning until a human makes meaning out of it by putting it in context. A data set is simply just numbers. It does not tell the story of why something is happening the way it is. A human must interpret and apply data to a real-life situation.
Risk assessments show probabilities, not certainties (Završnik, 2019). These risk assessments should be used as a tool, not as a definite decision-maker. They measure correlations and not causations. They also cannot judge whether the correlations are real or “ridiculous” (Završnik, 2019). Algorithms are created and trained by data, not “clean of social, cultural, and economic circumstances” (Završnik, 2019). Even the concepts of averages, standard deviations, probability, equivalences, regression sampling, and correlation are all the “result of historical gestation punctuated by hesitations, retranslations, and conflicting interpretations” (Završnik, 2019).
Decision making involves synthesizing various information, including “multimodal sensory inputs, autonomic and emotional responses, past associations, and future goals” next (Fellows, 2004). Different variables must be internally evaluated, including uncertainties, timing, values, cost-benefit, and risk involved to determine which action will be next (Fellows, 2004).
This evaluation process allows decision-makers to form expectations in terms of probabilities and confidence in the results of the perceived situation. These subjective values allotted to the different options allow for comparison of outcomes, including consequences. Cognitive biases can sometimes cloud one’s judgment, especially when it comes to making decisions. Cognitive biases can happen during the creation of schemas that directly impact how decisions are made in certain realms. While there are many types of biases, some subconscious and conscious all different kinds of biases can influence how one sees the world and, therefore, how one makes decisions when designing an algorithm. Prediction can be useful because it is input within decision-making. However, the prediction has no value in the absence of a decision. Prediction is also not the only element of making a decision.
The issue is not using algorithms and machine learning to guide decision making; the issue is telling the public that these machines take out all human biases and are completely, 100 percent objective when that has been proven time and time again not to be accurate.
Risk Assessment and Crime
Crime is a social phenomenon with multiple definitions and interpretations throughout history (Isaac, 2018). Since the early 1930s, the United States Department of Justice Crime reporting data has been on crimes known by the police and documented. However, still in 2020, crime is not being documented adequately and truthfully by many police departments. A most recent case that has received a lot of news attention is Breonna Taylor. Louisville Police Department left the police report blank on the injuries section even though she was shot eight times and killed (Stieb, 2020).
And many people know that rape is amongst the lowest crime to be reported due to various reasons. According to the Department of Justice, Office of Justice Programs, Bureau of Justice Statistics, National Crime Victimization Survey from 2010-2016 (2017), only 230 out of every 1,000 sexual assaults are reported to the police.
Therefore, there must be considerations for the implications of missing data and those who are left out of the data and the effects it may have on society and individual’s lives. A machine does not know about missing data. However, a human who is socially aware, making legal decisions, should be more aware. Put merely, crimes recorded and documented by the police departments are not a complete census of all criminal offenses, nor do they constitute a representative random sample. Furthermore, models of artificial intelligence really heavily on the training datasets to estimate predictions and are unable to adjust for institutional biases embedded within policing data (Isaac, 2018).
New technology should be adopted into society, especially when it can do amazing things that replicate human abilities. However, the design of the technology and its use must be able to withhold criticism. The mere showing of a risk assessment score influences judge’s decision making. Eckhouse et al., (2018) claims that by showing the risk score, it influences the judge’s decision by focusing on the potential recidivism over and above other relevant factors considered when not showing the risk score. Carlson (2017) also discusses a case where the COMPAS risk score was so high that the sentencing judge overturned the plea deal and sentenced the offender to two years, whereas the judge acknowledged without seeing that score, he would have only imposed a one-year sentence.
Human rights are being challenged with the implementation of artificial intelligence in courtrooms. There is no way to know if there is protection from legally protected discrimination. It is also challenging equality before the courts and the right to a fair and public hearing because there is no proven way to show the algorithms’ validity to fairness or objectiveness. In fact, this paper has shown multiple instances when algorithms have not been objective.
Conclusion
Humans are no exception to imperfect decision making. However, the difference between human decision making and computer decision making is that one can hold the judge who made the decision, accountable for their actions. Whereas with the computer, the company is not liable and has no accountability. They are legally protected not to have to share how their machine came to the conclusion it did. As a private company, the algorithms are given propriety protection. Researchers are not even allowed to attempt to audit algorithms without getting faced with a lawsuit from the private company. In the case of Loomis v Wisconsin, the company used to make a decision about his prison sentence formulated by the COMPAS algorithm, stated that it prevents judges, defendants, and researchers from vetting the algorithms and evaluating the fairness (Eckhouse et al., 2018) This has to change. A judge can be asked why they decided the way they did and must use evidence, claims, and logic.
Meanwhile, people just have to accept the decision given by a computer. People deserve to know why they are living the life they are living based on the judicial system. Instead, they are protecting the commercial interests of the private company. This challenges the principle of procedural justice, open justice, and individualized justice. The process when using an algorithm is mostly invisible and nobody can check the validity and reliability, yet people’s lives are at stake. This is not justice.
With the potential of algorithms to change the law’s nature and course, there is a need for responsible, transparent, and ethical algorithm design. There is also a need to ethically audit algorithms. Carlson (2017) suggests that instead of placing reliance on the private commercial sector and protecting their agenda, governments should develop their own actuarial and algorithms to hold up to the same accountability a judge would be held to.
The idea that criminal justice has been a human institution focused on human behavior and human threats means that it has aspired to obtain accountability, impartiality, and transparency. The incursion of secret algorithms created by private for-profit companies to be incorporated with judicial officials’ public duties challenges the presumed independence of the justice system.
References
Agrawal, A., Gans, J. S., & Goldfarb, A. (2019). Artificial Intelligence: The Ambiguous Labor Market Impact of Automating Prediction. Journal of Economic Perspectives, 33(2), 31–50. https://doi.org/10.1257/jep.33.2.31
Avery, J. (2019). An Uneasy Dance with Data: Racial Bias in Criminal Law. Southern California Law Review.
Carlson, A. M. (2017). The Need for Transparency in the Age of Predictive Sentencing Algorithms. Iowa Law Review.
Childs, M. (2011, October 31). John McCarthy: Computer scientist known as the father of AI. The Independent. https://www.independent.co.uk/news/obituaries/john-mccarthy-computer-scientist-known-as-the-father-of-ai-6255307.html.
Deeks , A. (2019). The Judicial Demand for Explainable Artificial Intelligence. COLUMBIA LAW REVIEW, 119(1829).
Department of Justice, Office of Justice Programs, Bureau of Justice Statistics, National Crime Victimization Survey, 2010-2016 (2017);
Eckhouse, L., Lum, K., Conti-Cook, C., & Ciccolini, J. (2018). Layers of Bias: A Unified Approach for Understanding Problems With Risk Assessment. Criminal Justice and Behavior, 46(2), 185–209. https://doi.org/10.1177/0093854818811379
Fellows, L. K. (2004). The Cognitive Neuroscience of Human Decision Making: A Review and Conceptual Framework. Behavioral and Cognitive Neuroscience Reviews, 3(3), 159–172. https://doi.org/10.1177/1534582304273251
Isaac, W. S. (2018). Hope, Hype, and Fear: The Promise and Potential Pitfalls of Artificial Intelligence in Criminal Justice. OHIO STATE JOURNAL OF CRIMINAL LAW, 15.
Larson, J., & Angwin, J. (2016, May 23). Machine Bias. ProPublica. https://www.propublica.org/article/machine-bias-risk-assessments-in-criminal-sentencing.
Mckay, C. (2019). Predicting risk in criminal procedure: actuarial tools, algorithms, AI and judicial decision-making. Current Issues in Criminal Justice, 32(1), 22–39. https://doi.org/10.1080/10345329.2019.1658694
O’Neil, C. (2016). Weapons of math destruction: how big data increases inequality and threatens democracy. Penguin Books.
Perrot, P. (2017). What about AI in criminal intelligence? From predictive policing to AI perspectives. European Police Science and Research Bulletin, (16).
Stieb, M. (2020, June 11). The Police Report on the Killing of Breonna Taylor Is Almost Entirely Blank. Intelligencer. https://nymag.com/intelligencer/2020/06/police-report-for-killing-of-breonna-taylor-is-nearly-blank.html.
Wexler, R. (2017, June 13). When a Computer Program Keeps You in Jail. The New York Times. https://www.nytimes.com/2017/06/13/opinion/how-computers-are-harming-criminal-justice.html.
Završnik, A. (2019). Algorithmic justice: Algorithms and big data in criminal justice settings. European Journal of Criminology, 147737081987676. https://doi.org/10.1177/1477370819876762