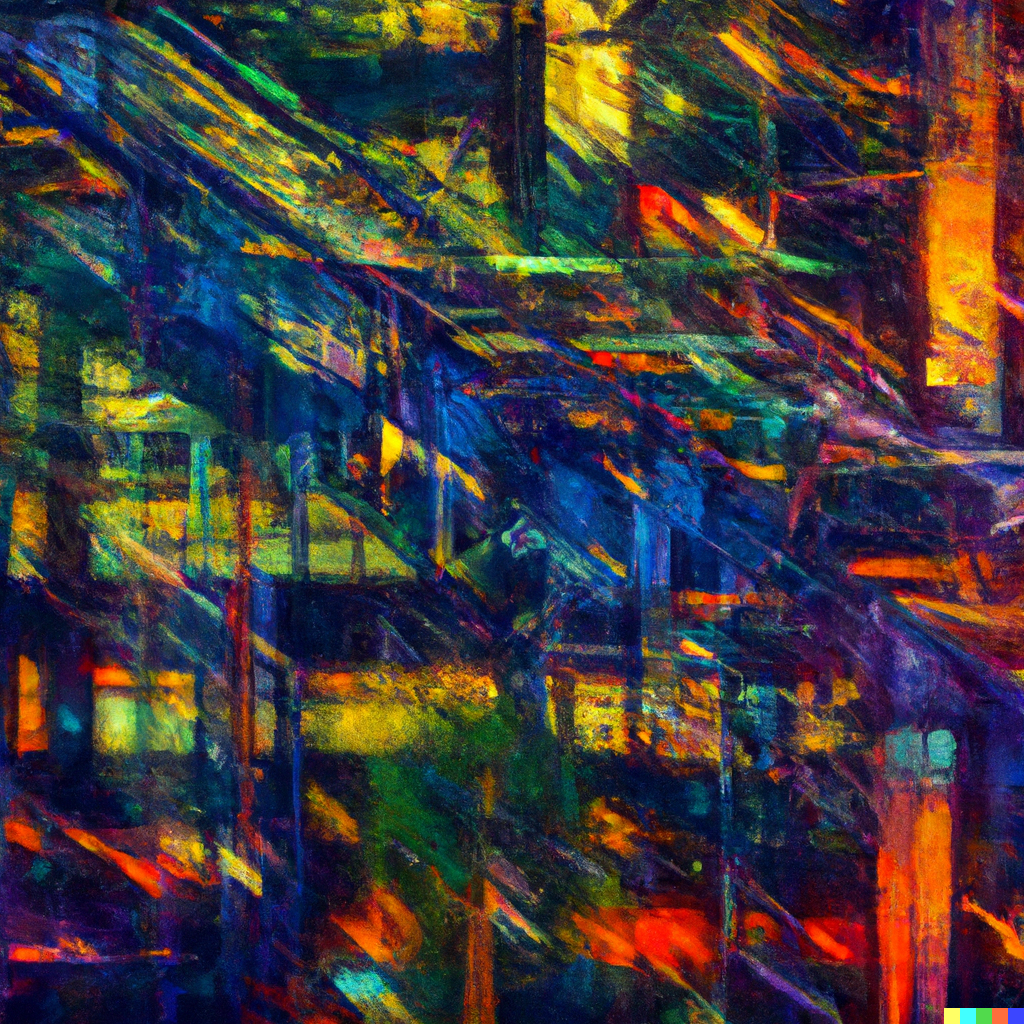
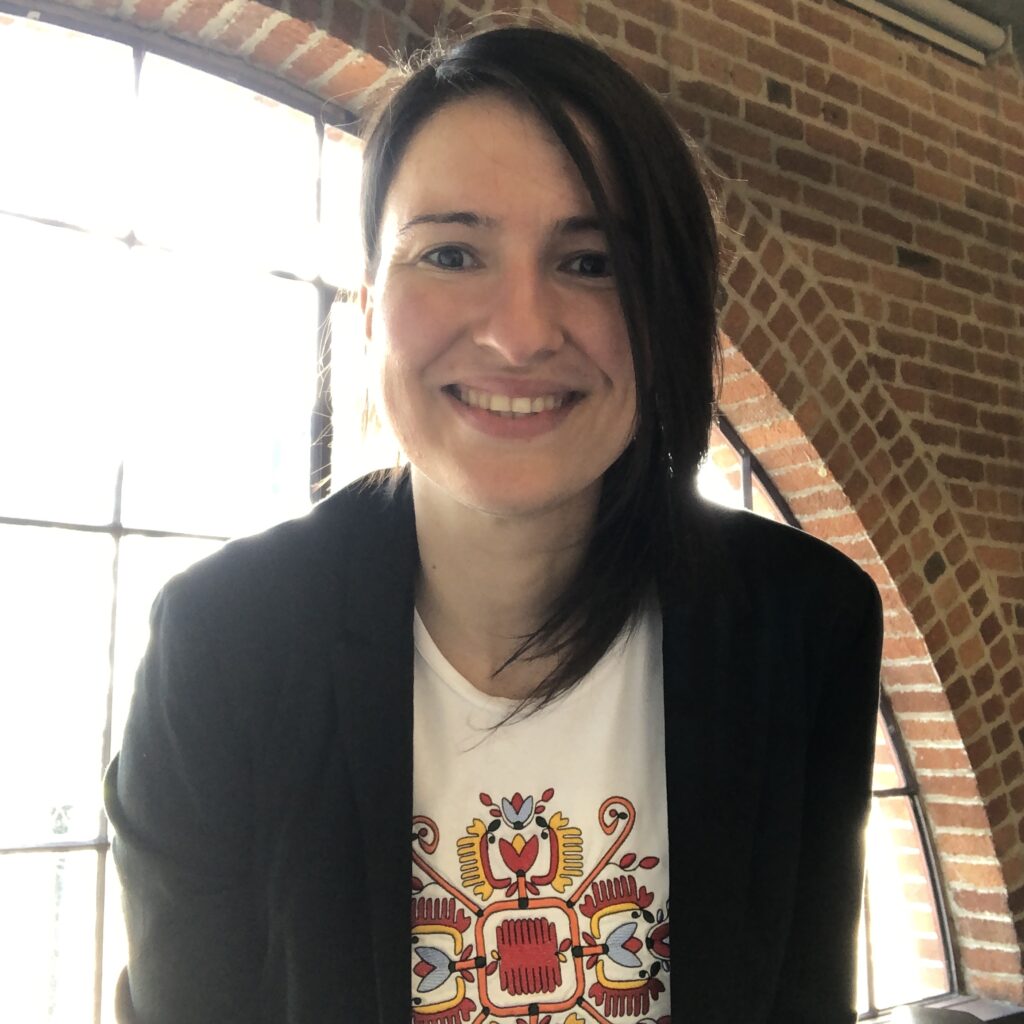
🔬 Research Summary by Bogdana Rakova, a Senior Trustworthy AI fellow at Mozilla Foundation, previously a research manager at a Responsible AI team in consulting, leading algorithmic auditing projects and working closely with ML Ops, ML development, product, and legal teams on strategies for human-centered model evaluation, documentation, AI literacy, and transparency.
[Original paper by Bogdana Rakova and Roel Dobbe]
Overview: In this paper, the authors argue that algorithmic systems are intimately connected to and part of social and ecological systems. Similarly, there are growing parallels between work on algorithmic justice and the scholarship and practice within environmental and climate justice work. We provide examples and draw on learnings from social-ecological-technological systems analysis to propose a first-of-its-kind methodology for environmental justice-oriented algorithmic audits.
Introduction
What questions arise when you think about the intersection of environmental and algorithmic justice? Data centers’ carbon footprint, water, and energy costs are some of the considerations in the news recently. Together with Roel Dobbe, we spent the last couple of years researching and learning from interdisciplinary fields to help practitioners grapple with questions such as:
- How might AI systems interfere with material resource flows, and in what ways might that burden local communities who depend on those resources?
- What are the potential impacts, risks, and threats of AI systems’ material flow and ecological dependencies and their computational infrastructure along the lifecycle – from mineral extractivism to data centers and infrastructures to electronic waste dumps?
- What information is shared with whom about the ecological impacts? What is considered data in building and auditing AI systems? What agency and power is given to engaged communities and stakeholders to shape the audit or design of AI?
Similar questions need to be addressed throughout the lifecycle of an AI system, both in cases where AI is used in environmental sustainability projects and when practitioners are engaging with environmental aspects of an algorithmic audit.
We conduct a broad literature review and discuss these and other questions through a detailed analysis of emerging themes within the Environmental Justice (EJ) movement. Then we turn to the field of complexity science in search of strategies and best practices for practitioners involved in algorithmic auditing. We provide policy recommendations that support practitioners looking to address these questions practically. These are: (1) broaden the inputs and open up the outputs of an audit, (2) enable meaningful access to redress, and (3) guarantee a place-based and relational approach to the process of evaluating impact. Beyond algorithmic auditing, we believe our findings could provide meaningful insights for diverse stakeholders involved in designing, developing, and evaluating AI-enabled products and services.
Key Insights
Emergent Challenges in the Algorithmic Auditing Ecosystem
As in any research, it is important to acknowledge our own positionality and how it impacts our work. In reviewing recent work on challenges in algorithm auditing, we took a complex systems lens. Thus, (AI) systems are configurations of dynamic interacting social, technological, and environmental elements which we can understand in terms of their structures and functions. We need to investigate – what are their boundaries?; how are interactions across these boundaries constructed and constituted?; what are their functional outputs and consecutive impacts? (See Melissa Leach et al.).
Operationalizing audits
To disentangle constitutive relations in a system, we must understand (a) the role of power dynamics among actors who determine the system boundaries, (b) the system’s internal dynamics, and (c) interactions with what’s external to the system boundaries. In the context of designing and evaluating AI systems, these questions remain ever more prevalent. There are growing accounts of existing challenges in operationalizing meaningful algorithmic auditing in practice. For example, lack of buy-in for conducting an audit, lack of enforcement capabilities, a dislocated sense of accountability, reliance on quantitative tools instead of qualitative methods, and the impacts of advocating for broader participation without directly addressing existing power asymmetries.
Complex environmental costs
AI systems depend on massive computational resources, and the implications go beyond carbon footprint. Increasingly, recent research has investigated the environmental footprint of the computational infrastructure required to build, deploy, and use an AI system and the cost of disposing of it once it has exhausted its lifespan. Data centers play a central role in the life cycle of current AI systems. The water and carbon costs of data centers directly affect the livelihoods of the people living in the territories where they are built. Communities all over the world have engaged in protests against the construction of new data centers in their neighborhoods. (See a landscape review by the AI Now Institute). Furthermore, scholars have challenged the fuzzy notions of AI for sustainability and sustainable AI, arguing the need for a relational approach to understanding the world wide web of carbon and the factors influencing the carbon emissions of machine learning models.
Just Sustainabilities
In conducting AI impact assessments, we need to ask what is our model of justice. We invite practitioners to ground this question in the parallels in the field of algorithmic justice and the longstanding theory and practice within the EJ movement. Theory and practice in the EJ movement have long grappled with struggles related to (1) distributive justice – equity in the distribution of environmental risk; (2) recognitional justice – recognition of the diversity of participants and experiences in affected communities; and (3) procedural justice – opportunities for participation in the political processes that create and manage environmental policy. As such, its legacy serves as a model of justice, both in its normative orientation and evolution as a field and a movement. In a recent EJ annual review, Agyeman et al. provide a comprehensive overview of EJ literature and goals in advancing climate solutions that link human rights and development in a human-centered approach, placing the needs, voices, and leadership of those who are most impacted at the center.
In our analysis of EJ literature, we seek to center Agyeman, Bullard, and Evans’s conception of “just sustainabilities,” which distills the convergence of social justice and environmental sustainability. They write that just sustainabilities aim to “ensure a better quality of life for all, now, and into the future, in a just and equitable manner, while living within the limits of supporting ecosystems.” The relationships between the environment and justice are complex and mutual.
The evolution of methodologies and multiple interpretations of EJ has contributed to emergent themes within the EJ frame and discourse, including (1) focus on the practice and materiality of everyday life; (2) the impact of community, identity, and attachment; and (3) growing interest in the relationship between human and non-human assemblages. In the paper, we analyze these themes in EJ and existing work in the algorithmic justice and algorithmic auditing discourse.
Algorithmic systems are ontologically indistinct from Social-Ecological-Technological Systems
In view of emergent barriers to meaningful auditing that improves justice outcomes, we turn to Social-Ecological-Technological Systems (SETS) analysis. SETS have emerged as a tool for complex dynamic systems analysis, central to more radical approaches to understanding planetary boundaries and sustainability studies. The SETS framework investigates the couplings or relationships between a system’s social, ecological, and technological dimensions. Ecological justice scholars Melissa Pineda-Pinto et al. define these as:
- social-ecological couplings refer to human–nature or social-ecological relationships, feedback, and interactions that impact human well-being and ecosystem services;
- social–technological couplings refer to how technology and human social systems interact, shaping social norms, values, and belief systems;
- ecological–technological couplings refer to the different ways in which climate and biophysical systems impact technology [and vice-versa], such as the implications of extractive and polluting technological infrastructures;
Drawing from this body of work, we argue that algorithmic systems are ontologically indistinct from SETS. That is: (1) they are dependent on social and ecological resources to be built, used, and maintained; (2) they contribute to carbon and ecological costs through their dependency on computational infrastructure and their role in fossil fuel production; and (3) any problem that is solved through AI has underlying technical, social, and institutional components and their interactions and infrastructures depend on and impact other social-ecological-technological systems.
A SETS evaluation framework for AI
We overlay the dimensions of SETS analysis and emerging themes in EJ to motivate a set of questions that practitioners could operationalize in the algorithmic audit process. See them in the table below. Thus, our theory-of-change is centered on the need and utility of SETS analysis to enable interdisciplinary collaborations in disentangling the complex nature of algorithmic impacts in a more-than-human world.
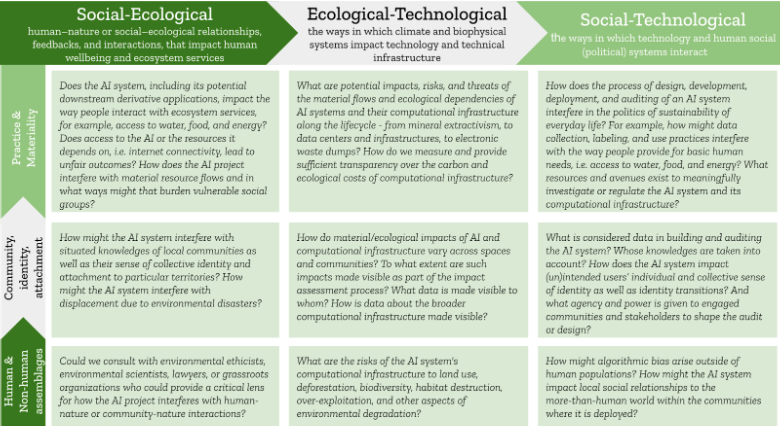
Towards a more effective normative debate and design interventions, we identify how SETS scholars have grappled with these questions and draw policy recommendations that could positively contribute to the evolving algorithmic auditing ecosystem. Giving examples from SETS, we call for broadening the inputs and opening up the outputs of an audit by moving away from narrow and closed risk-based approaches to impact assessment towards broad and open framings such as participatory and deliberative mapping. Within EJ, many community-led initiatives examine how environmental data about harms and injustices moves between communities and policymakers. Similarly, within AI, we identify the need for (1) prototyping mechanisms for reporting algorithmic incidents, controversies, or injustice experienced by (un)intended users of AI systems, (2) considering how such mechanisms are affected by and may contribute to existing power structures, and finally (3) the role of workers in a growing number of calls for solidarity.
Finally, we argue for the need for a place-based and relational approach to the process of evaluating impact. SETS scholars have demonstrated the role of place-based research methods as a key contributor to achieving global sustainability goals (See Balvanera et al.). For example, arguing that transformations towards sustainability are often triggered at the local scale through the co-construction of local solutions in particular territories. By analyzing the strengths and challenges of place-based socio-ecological research, SETS work highlights that a place-based approach enables the consideration of long-term time horizons, capacity building, local communities of practice, and a bridge between local and global sustainability initiatives. Drawing from SETS scholarship and practice, we argue for AI builders and policymakers to enable local communities where the technology is deployed to evolve their own tools and processes for algorithmic audits.
A case study
In a recent Mozilla Mozfest workshop in collaboration with Kiito Shilongo, we explored a case study of using the proposed qualitative SETS framework in the context of AI used in conservation projects in East Africa. Specifically, an AI system called AI TrailGuard was developed by Intel in the US and marketed as technology to stop poachers before they kill. AI TrailGuard is a motion-capturing camera that alerts the ranger team of the presence of people, specific wildlife, or vehicles, in the dark and without an internet connection. Using the SETS evaluation framework, we discussed that calls for AI transparency need to meaningfully account for the social context and political economy of the places where AI is deployed, including the dynamic impacts on non-human beings and ecosystems. Who does this technology empower? For example, in what ways could the AI TrailGuard impact land use, deforestation, biodiversity, habitat destruction, over-exploitation, and other aspects of environmental degradation? How does the AI Trailguard interfere with material resource flows, and how might that burden the communities who depend on the Serengeti or live with the wildlife? Read about the challenges in that space in Kiito’s article on data and tech governance in Namibia.
Between the lines: An Ecosystemic vision for AI
We hope this work provides a starting point for discussion and a useful roadmap for future research, case studies, and practical steps toward improved environmental justice outcomes in how AI systems are developed and used throughout society. We invite you to read the full paper, engage with the SETS evaluation framework, and join us in co-creating an ecosystemic vision for AI through a working group we launched when presenting this work at the FAccT 2023 Conference.