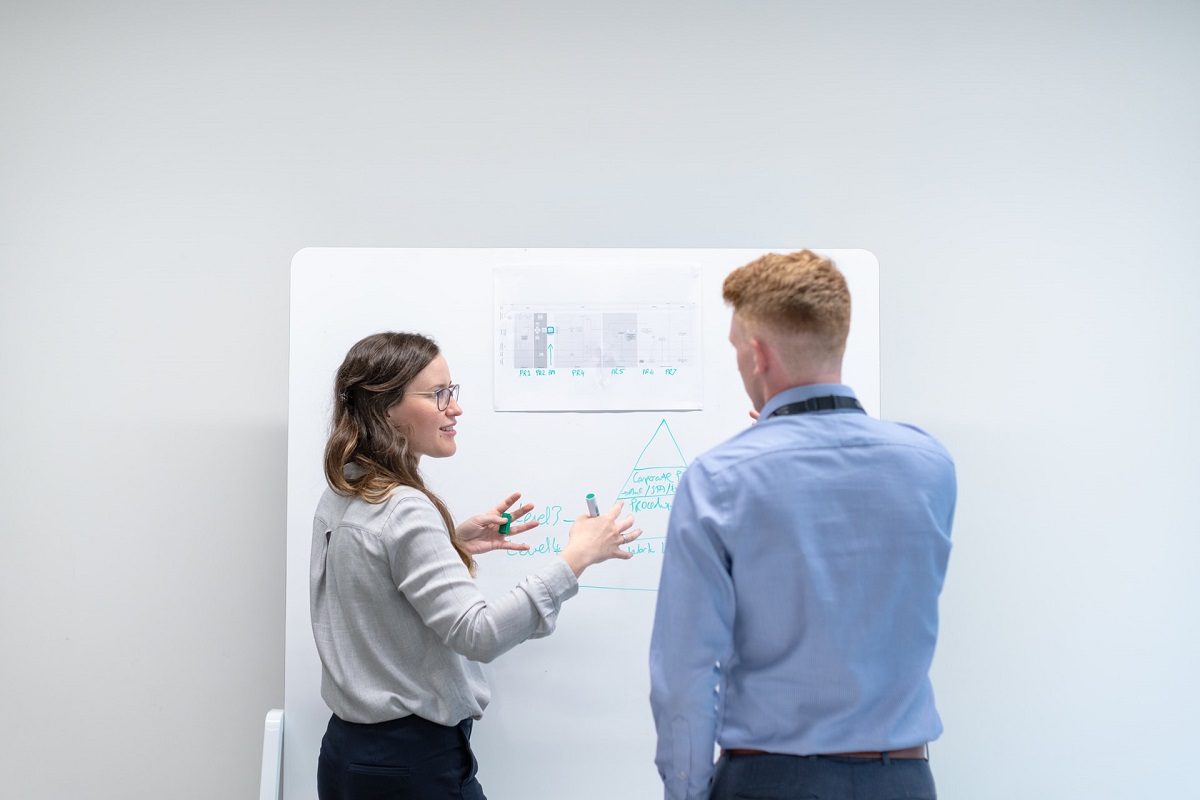
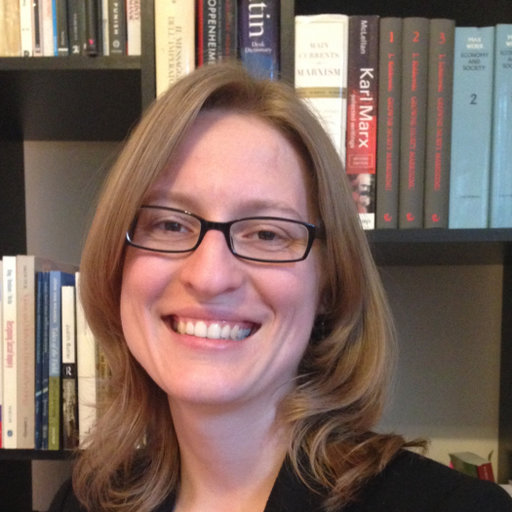
🔬 Research summary by Dr. Iga Kozlowska (@kozlowska_iga), a sociologist working on Microsoft’s Ethics & Society team where she guides responsible AI innovation.
✍️ This is part 9 of the ongoing Sociology of AI Ethics series; read previous entries here.
[Original paper by Jacob Metcalf, Emanuel Moss, Elizabeth Anne Watkins, Ranjit Singh, and Madeleine
Clare Elish]
Overview: Algorithmic Impact Assessments (AIAs) are a useful tool to help AI system designers, developers and procurers to analyze the benefits and potential pitfalls of algorithmic systems. To be effective in addressing issues of transparency, fairness, and accountability, the authors of this article argue that the impacts identified in AIAs need to as closely represent harms as possible. And secondly, that there are accountability forums that can compel algorithm developers to make appropriate changes to AI systems in accordance with AIA findings.
Introduction
Writing an Algorithmic Impact Assessment (AIA) is like painting an impressionist landscape of Van Gogh’s swirling cypresses or Monet’s floating water lilies. Well, maybe not exactly, but stay with me.
First, what are AIAs anyway? Metcalf et al. define AIAs as “emerging governance practices for delineating accountability, rendering visible the harms caused by algorithmic systems, and ensuring practical steps are taken to ameliorate those harms.”
If you’re an AI developer, your company may already have instituted this practice. You may have experienced this practice as a document your team has to fill out that answers questions about the machine learning model or algorithmic system you’re building, maybe with the help of some ethical AI subject matter experts.
While praising these efforts as a good start, the authors focus on AIAs’ existing shortcomings.
Specifically, they describe two key challenges with doing AIAs in such a way that they truly prevent harms to people:
- Impacts are only proxies for real harm that people can experience
- AIAs don’t work without an accountability mechanism
Impacts as proxies for harms
The authors argue that impacts don’t necessarily measure harms and may, in worst-case scenarios, obscure them. Describing real harms is very difficult because it’s a truth that, like many social and psychological aspects of humanity, is hard to evaluate and represent in words, let alone to quantify.
For example, in your AIA, you may measure how far your model deviates from your fairness benchmark, which may be based on a company policy (or just group consensus) that model outcomes shouldn’t diverge more than 10% across some demographic characteristics (let’s say age, gender, race) and their intersections. That metric measures the impact of your, say face recognition model, on your customer’s ability to get equal quality of service. The impact is that there will be no more than a 10% difference in predictive quality between, for example, young Black women and older white men.
But the metric is not measuring the emotional or psychological harm done to individuals let alone entire communities when they get repeatedly misrecognized by the algorithm. It does not capture even the more easily quantifiable harms like an economic loss that can stem from such biased systems. The impact is only an indirect measure of underlying harm.
In other words, just like with an impressionistic painting, we may get a mediated sense of reality through the painting, but we don’t come close to the underlying lived experiences of actually being in a field of Van Gogh’s sunflowers. We don’t even get a view that we might see in a pre-modernist painting where the name of the game was to convey a scene with as close to photographic precision as possible. The impressionistic view of impacts is still useful, but unlike with modernist painting, there is immense value in getting as close to a true representation of reality as possible, knowing that it will never be 100% perfect.
Moreover, when doing an AIA, it is difficult to evaluate its comprehensiveness because there is no objective standard against which to measure. When do you know you’ve adequately predicted all impacts that could potentially face your customers and other indirect stakeholders of your product?
Instead, the quality of an AIA is determined through the consensus of experts who happen to be at the table. And we know that not all voices, particularly those of marginalized communities, are going to be present at the table. Likewise, few product development teams hire people with psychology or social science backgrounds, so those perspectives are likely to be absent.
In other words, much like art, which is judged based on expert and/or public consensus and has no singular objective standard, the adequacy of AIAs is currently judged by what the authors call “epistemic communities” that are not necessarily representative of all voices needed to actually prevent harms.
AIAs need accountability
Just as there is no one who can legitimately tell an artist that they must change their work, with AIAs there is, as of yet, no authority that can mandate that an organization make changes to its AI systems based on what is found in an AIA. With no “forum” of accountability, as the authors call it, a company can write an AIA and yet make no mitigations to the AI system that would actually reduce harm.
Here is where the art metaphor really breaks down. Whereas we obviously don’t want a regulatory agency enforcing certain artistic practices or styles—that is called censorship—in the case of AIAs, the authors argue, some accountability body is required. Such a mechanism is necessary to ensure that organizations do AIAs in the first place, do them well, and actually act on them. Doing an AIA just to check a box without it informing the design and development of the AI system does not reduce harm to users.
Between the lines
Completing an AIA may not be as profound or satisfying as painting an impressionist masterpiece. But it certainly is an art form that requires skill, knowledge, and the social construction of a world of algorithmic accountability. And, like a great piece of art, it can encourage us to reflect, motivate us to act, and hopefully create change for the better.
It’s too early to tell just how common AIAs will become or how effective they will be in changing the shape of algorithm-based technology. Classification and prediction algorithms have already proven to cause real-world harm to those least advantaged, whether it’s in the context of criminal justice or child abuse prevention. AIAs are a great immediate intervention, but without robust accountability measures, they might fall short of what most of us truly want: technology that amplifies—not erodes, human dignity.