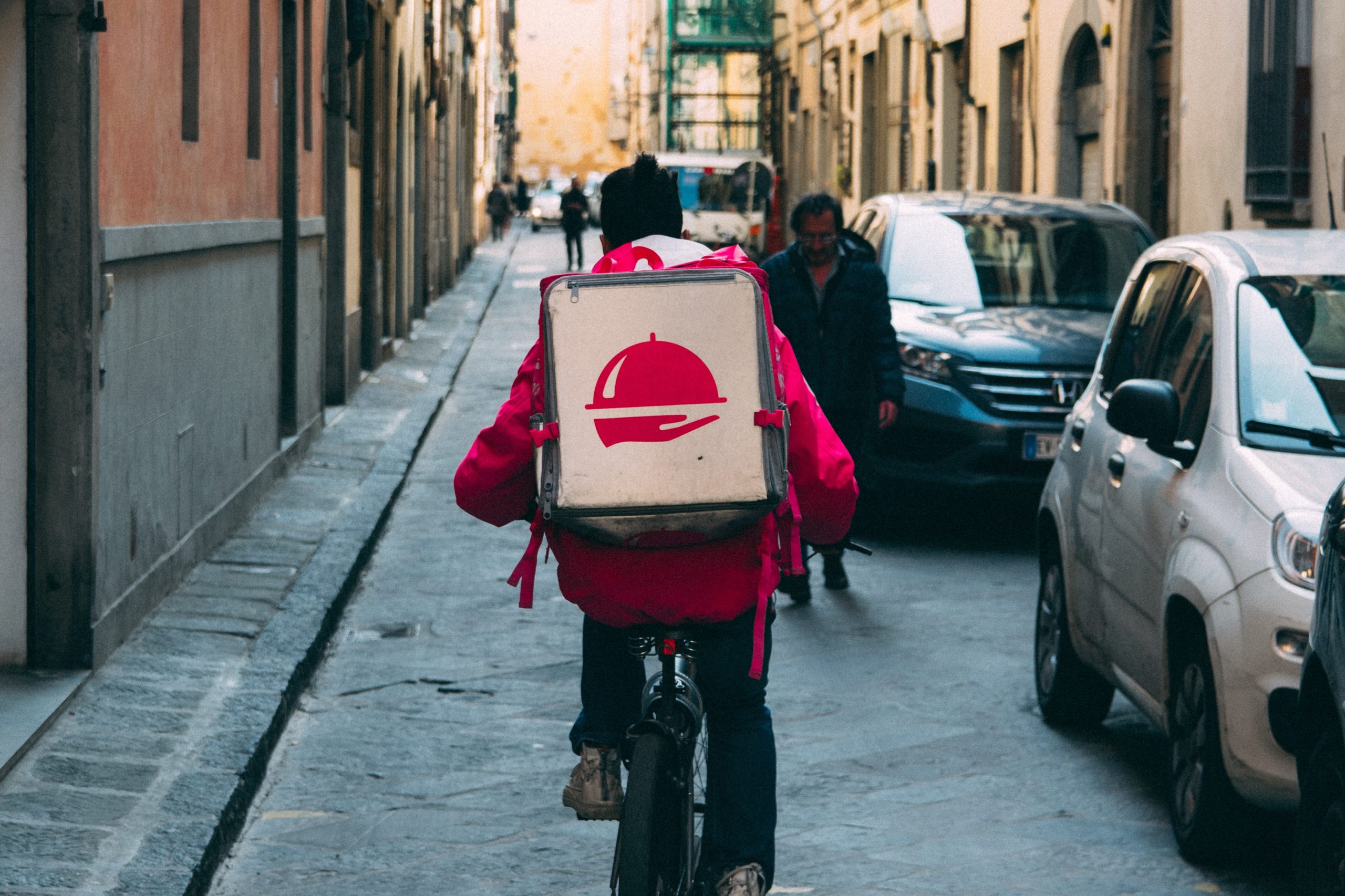
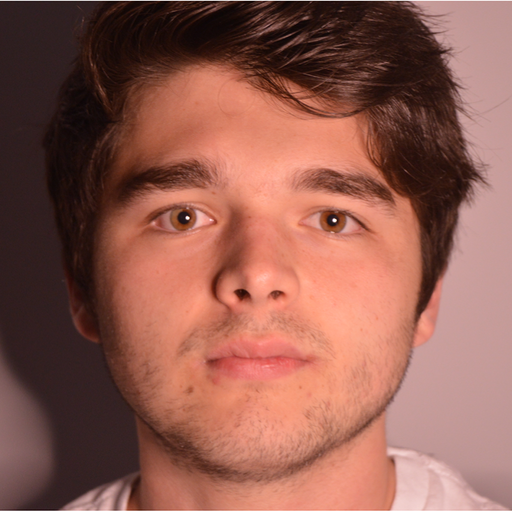
đŹ Research summary by Connor Wright, our Partnerships Manager.
[Original paper by James Muldoon and Paul Raekstad]
Overview: Despite being seen as the solution to human bias, algorithms foster a relationship of domination and a power structure between bosses and workers. We explore how this is expressed within the gig economy and the subsequent precarious situation for its on-demand workers.
Introduction
Algorithms were initially employed as a solution to human bias. However, increasing reports question whether they can avoid this trap. Studying the effect of âalgorithmic dominationâ (p. 2) on our freedom, the authors focus on the ride hail and food delivery sectors within the Gig economy (based around contractors receiving short-term tasks). Here, focusing on on-demand workers, algorithmic domination is being subjected to a power over which you have no control. Not being a new form of power, algorithmic domination amplifies existing power controls. I will now explore how this amplification is expressed and how it affects the workers involved.
Key Insights
Utilising a Republicanism framework, domination through algorithms is interpreted as âsubject to uncontrolled powerâ (p. 4). Algorithms facilitate the concept in three different ways:
- The control of the flow of information
Bosses can withhold key information from workers. Most apps examined within the literature covered are unidirectional, meaning workers cannot communicate directly with the company or negotiate different parts of their contract as they would do with human management. Even within the limited communication they can engage in, workers are second-guessing whether they receive communications from an algorithm or human agent.
With lesser human engagement involved, workers are less likely to have the chance to negotiate any potential compromises. The unidirectional nature of the apps covered also means that workers do not have access to even their own data, let alone relevant information to inform any work-related decisions they make. Hence, the disparities between âknowledge and computational capacities exceed certain standard employer/employee relationshipsâ (p. 7). Consequently, workers are at the informational behest of those in charge.
- Performance assessment
Bosses can assess the performance of other workers in a more round-the-clock surveillance fashion through the use of algorithms. Furthermore, this automation means that the effects of customer reviews on these workers are profound.
- Who carries out the task and when
Workers are subjected to leadership decisions on who carries out a task and when it gets done. The dynamic pricing utilised within ride hailing and food delivery apps mean that workers cannot contest the remuneration offered for different tasks. As a result, prices fluctuating at peak times allow the company even to control when people work.
In sum, these contexts give rise to âalgorithmic managementâ (p. 6), which is a more pervasive, continuous and intense form of management.
Reflecting on algorithmic domination
It is worth noting that algorithmic domination is not all about the algorithm, but how it perpetuates the already present asymmetric power relations between bosses and workers. Services such as Uber and Deliveroo see themselves as championing ideals of flexible and autonomous labour, yet the algorithm relies on âtechnology, social relationships and economic institutionsâ (p. 7) within these entities to fully take hold. Hence, bosses deciding between opting out or opting-in to sustain such a system becomes the pivotal moral moment.
While prolonging such a situation, companies have vowed to âdo betterâ. Yet, without advocating for systemic change, such promises amount âto a claim about their intentions to use their uncontrolled power in better ways, not to actions that would remove their uncontrolled powerâ (p. 13). Higher pay and better conditions donât eliminate any power relations but serve to blow smoke over the power dynamics at play. Hence, without advocating for changing the rules of the game, âwe should be sceptical of voluntary pledges by tech companiesâ (p. 8).
Between the lines
I found this text both illuminating and frightening at the same time. While the âalgorithmic panopticonâ (p. 13) is not an inevitable fate, the precarious employment conditions are very tangible. Removing the human element from management takes away workersâ ability to fight for themselves, losing the opportunity to negotiate with a fellow being and instead now bang their heads against an automated brick wall. Hence, while there is a superficial sense of liberty involved in the Gig economy, the invisible algorithmic chains still persist.