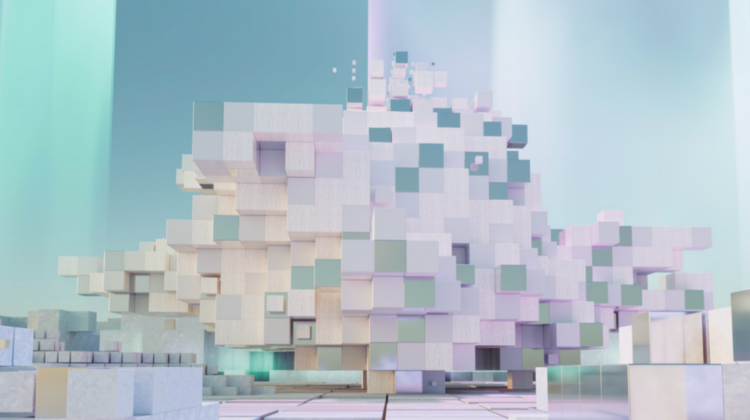
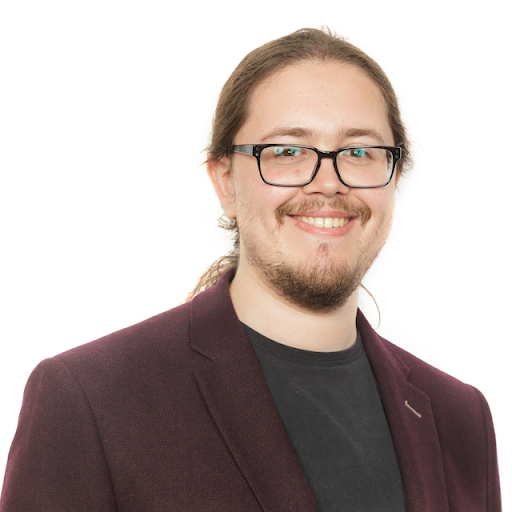
🔬 Research Summary by Ismael Kherroubi Garcia. Ismael is trained in business management and philosophy of the social sciences. He is the founder and CEO of Kairoi, the AI Ethics and Research Governance Consultancy, and founder and co-lead of the Responsible AI Network (RAIN).
[Original paper by Jodie Pimentel (International WELL Building Institute) and Ismael Kherroubi Garcia (Kairoi)]
Overview: How do we safeguard people’s health in built environments where AI is adopted? Research led by the International WELL Building Institute (IWBI) and Kairoi sets out a framework for built environment sectors to deploy and adopt AI in ways that are beneficial for people’s health and well-being.
Introduction
Most of us spend the vast majority of our lives indoors – inside offices, homes, schools, shops…1 The industries that service these spaces – the built environment sectors – are increasingly interested in the prospects of AI.2 This matters because those industries are vast and diverse, including architecture, engineering, construction, facilities operations and real estate.
The adoption of AI in built environments warrants careful thought. On the one hand, there may be undue excitement among built environment sectors that lack a deep understanding of AI technologies.3 On the other hand, built environments deal with critical infrastructure, possibly rendering some AI systems “high risk” – and, therefore, subject to robust requirements – according to the EU AI Act:
“[…] It is appropriate to classify as high-risk the AI systems intended to be used as safety components in […] the supply of water, gas, heating and electricity.” 4
With this, it is crucial that built environment sectors adopt and deploy AI in a way that is thoughtful, mitigates risks, and strives for positive changes in how organizations operate and how people lead their lives.
Key Insights
At the complex intersection of AI, built environments, and human well-being, the International WELL Building Institute asked: How can AI applications promote a people-first approach to buildings and organizations?
By identifying AI Champions with diverse perspectives, drawing on rigorous research, and applying Kairoi’s AI Ethics Canvas, the team identified authoritative principles and pathways to develop a framework considering “baseline behaviors” and “aspirational goals” when making AI-related decisions.
Part from the UN Global Compact
New AI tools should not distract built environment sectors from good business practices, and the UN Global Compact sets out industry standards to which IWBI and many of their stakeholders subscribe.5
The following table collates instances that demonstrate the relevance of the UN Global Compact when making AI-related decisions:
Impact area | Principle | Example of relevant AI risk |
Human Rights | Businesses should support and respect the protection of internationally proclaimed human rights. | AI tools may be trained on enormous amounts of data regardless of privacy and intellectual property rights.6 |
Businesses should make sure that they are not complicit in human rights abuses. | AI technology could contribute to digital violence against women and girls.7 | |
Labor | Businesses should uphold the freedom of association and the effective recognition of the right to collective bargaining. | AI tools may be used for surveillance and to counter unionization.8 |
Businesses should uphold the elimination of all forms of forced and compulsory labor. | AI tools may be sustained through deeply questionable employment practices.9 | |
Businesses should uphold the effective abolition of child labor. | AI data-labeling services have hired young teens.10 | |
Businesses should uphold the elimination of discrimination in respect of employment and occupation. | AI tools have perpetuated discrimination in staff recruitment.11 | |
Environment | Businesses should support a precautionary approach to environmental challenges. | Organizations should be aware of methods for monitoring the energy consumption for developing the AI tools they use.12 |
Businesses should undertake initiatives to promote greater environmental responsibility. | AI can be used to spread disinformation about climate change at scale.13 | |
Businesses should encourage the development and diffusion of environmentally friendly technologies. | Despite its potential for analyzing climate data, the infrastructure supporting AI has significant environmental impacts.14 | |
Anti-corruption | Businesses should work against corruption in all its forms, including extortion and bribery. | ”Corrupt AI” refers to the “abuse of AI systems by (entrusted) power holders for their private gain”.15 |
Aspire for the UN Sustainable Development Goals (SDGs)
AI also means new possibilities for built environment sectors, which can promote the UN SDGs by deploying and adopting AI thoughtfully. To translate global aspirational goals into practical guidance, Kairoi’s four pillars of responsible AI serve as a bridge between principles and action:
- Better communications: Articulate AI-related decisions to diverse stakeholders – from investors and funders to policymakers and the broader public – in accurate and thoughtful ways. This promotes AI literacy and combats AI hype.
- Relevant technical solutions: Follow practices for the safe, secure and robust design, development and deployment of AI tools and research. Technical strategies help organizations meet industry standards and develop effective AI tools and systems.
- Meaningful public engagement: Enable diverse stakeholders to participate in AI-related decision-making processes. This engenders trust in industry actors developing and adopting AI, which is done in light of real societal needs.
- Robust governance: Ensure legal compliance, engage with AI-related policy-making processes and document decisions. This enables innovation and allocating responsibility and accountability transparently.
Evaluating relevant SDGs in conjunction with the four pillars enabled the research team to brainstorm relevant organizational interventions and solutions. With this, the report suggests 36 clear activities that built environment sector organizations can implement to promote the UN SDGs when making AI-related decisions.
Between the lines
The AI Framework for Healthy Built Environments is grounded in real organizational practices and encompasses many topics that interest diverse built environment sectors. The framework was informed through an iterative process involving diverse staff from across IWBI, and an expert roundtable held in May 2024 at the WELL Conference. During the roundtable, industry leaders welcomed the document’s mention of the environmental footprint of AI technologies, keenly explored data management practices brought to life by case studies and readily discussed the role of AI in ESG disclosures.
With this, the framework sets out pragmatic changes that many organizations may follow. It also sets out a roadmap for its own development. In its final section, the document outlines the ongoing aims of IWBI to explore how AI and equity intersect for built environment sectors and to seek industry-wide partnerships for the promotion of good AI practices. This is key for all AI-related frameworks out there: we cannot simply conclude projects with a report, but must continue to promote its findings to lead positive change.
Footnotes
- Roberts, T. (2016, December 15). We Spend 90% of Our Time Indoors. Says Who?, Building Green, online [accessed 02 June 2024] ↩︎
- Jll.co.uk (2023) How the construction industry is adopting AI, JLL, online [accessed 02 June 2024] ↩︎
- Pimentel, J. & Kherroubi Garcia, I. (2024) Moving Beyond the Hype: Advancing Common Principles for Responsible AI in the Built Environment, WELL, online ↩︎
- European Parliament (2024) European Parliament legislative resolution of 13 March 2024 on the proposal for a regulation of the European Parliament and of the Council on laying down harmonized rules on Artificial Intelligence (Artificial Intelligence Act) and amending certain Union Legislative Acts (COM (2021) 0206 — C9-0146/2021 — 2021/0106 (COD)), online [accessed 02 June 2024] ↩︎
- United Nations Global Compact (n.d.) The Ten Principles of the UN Global Compact, online [accessed 24 March 2024] ↩︎
- United Nations Human Rights Office of the High Commissioner (2024) Taxonomy of Human Rights Risks Connected to Generative AI, B-tech, online [accessed 15 April 2024] ↩︎
- Cerise, S. et al. (2022) Accelerating Efforts to Tackle Technology Facilitated Violence Against Women and Girls (VAWG), UN Women, online [accessed 21 April 2024] ↩︎
- Del Rey, J. & Ghaffary, S. (2020) Leaked: Confidential Amazon memo reveals new software to track unions, Vox, online [accessed 15 April 2024] ↩︎
- Williams, A. et al. (2022) The Exploited Labor Behind Artificial Intelligence, Noema Magazine, online [accessed 15 April 2024] ↩︎
- Rowe, N. (2023) Underage Workers Are Training AI, Wired, online [accessed 15 April 2024] ↩︎
- Dastin, J. (2018) Insight – Amazon scraps secret AI recruiting tool that showed bias against women, Reuters, online [accessed 15 April 2024] ↩︎
- Strubell, E. et al. (2019) Energy and Policy Considerations for Deep Learning in NLP, In the 57th Annual Meeting of the Association for Computational Linguistics (ACL). Florence, Italy, DOI: 10.48550/arXiv.1906.02243 ↩︎
- Climate Action Against Disinformation et al. (2024) Artificial Intelligence Threats to Climate Change, foe.org, online [accessed 15 April 2024] ↩︎
- Gonzalez Monserrate, S. (2022) The Staggering Ecological Impacts of Computation and the Cloud, The MIT Press Reader, online [accessed 15 April 2024] ↩︎
- Köbis, N.C. et al. (2022) The corruption risks of artificial intelligence, Transparency International, online [accessed 15 April 2024] ↩︎