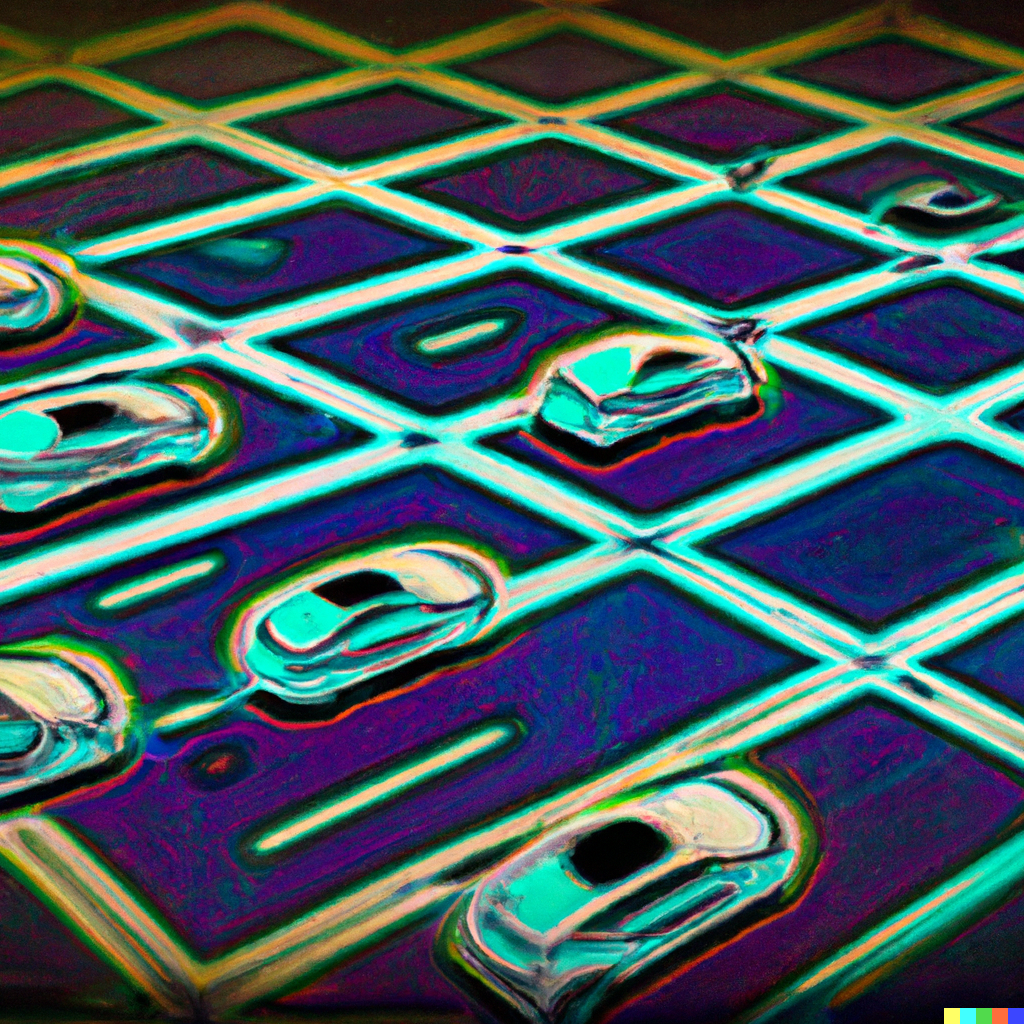
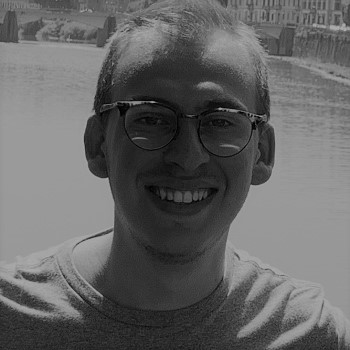
By Anthony De Luca-Baratta (Philosophy & Political Science, McGill University)
This paper explores how traditional consequentialism falls short in scenarios involving autonomous vehicles and social robots. A modified version dubbed ‘risk consequentialism’ is put forward for consideration as an approach that can help guide policy decisions in grave risk scenarios while accommodating a high-uncertainty future.
Topics discussed: consequentialism, grave risk scenarios, the trolley problem, autonomous vehicles, social robots & empathy, AI safety.
Introduction
In this paper, I will argue, on consequentialist grounds, that the primary ethical concern that should inform and guide both the design of AI systems and society’s regulation of the treatment of social robots is the mitigation of risk. I will show that traditional consequentialism is unable to serve as an adequate guide for decision-making in these two projects because it is ill-equipped to deal with decision-scenarios in which there is a high level of uncertainty about the possible outcomes of one or more of the actions available to the agent, but in which there is a high level of certainty that at least one of the possible outcomes would involve grave harm.
I call such decision-scenarios inscrutable grave risk scenarios. I will argue that decision situations in the design of ethical AI and the regulation of social robots are such scenarios. Finally, I will argue that an appropriately altered version of traditional consequentialism, which I call risk consequentialism, is better equipped to deal with such cases. Risk consequentialists, when faced with inscrutable grave risk scenarios, should seek to minimize the risk of the occurrence of the outcomes that they know would cause grave harm.
I will begin with a brief description of what I will call traditional consequentialism. This description is not meant to be a summary of all consequentialist thought. Rather, it is meant as an attempt to capture what I see as the two basic underlying principles that are necessary components of all consequentialist theories.
The first principle that all consequentialists must accept is that “certain normative properties depend only on consequences” (Sinnott-Armstrong).
Secondly, traditional consequentialism presupposes either some ability to make reasonable probabilistic calculations about the future or the availability of some rule of thumb that can reliably be employed in decision situations in which the future is inscrutable. For example, Jeremy Bentham, John Stuart Mill, and Henry Sidgwick, the fathers of classic utilitarianism or “hedonistic act consequentialism”, all argued for an ethical theory that sought to maximize net pleasure (Sinnott-Armstrong). Bentham, Mill and Sidgwick are in the historical group of consequentialists that do not believe that agents should consciously attempt to calculate the amount of pleasure that would result from the different actions available to them every time they make a decision. Consequentialists like them argue that pleasure maximization (or the maximization of one or more other goods), is the criterion by which an action should be judged post facto.
Every day decisions are to be made using some other decision procedure (Sinnott-Armstrong). Some consequentialists of the same school of thought propose following one’s moral intuitions, believing that “these intuitions evolved to lead us to perform acts that maximize utility, at least in likely circumstances” (Sinnott-Armstrong). Others posit rule consequentialism as a guide to decision making, arguing that agents should follow rules that maximize the good (Hooker). Those consequentialists from the probabilistic school argue that “moral rightness depends on foreseen, foreseeable, intended, or likely consequences, rather than actual ones”, suggesting that moral agents should make decisions that maximize the probable or foreseeable good rather than the actual one (Sinnott-Armstrong).
In summary, traditional consequentialism posits that the moral status of actions depends solely on those actions’ consequences. Further, moral agents should seek to maximize the good either by applying rules of thumb that they believe will tend to do so if consistently followed, or by always making decisions that will maximize the good according to some probability calculation or reasonable belief about foreseeable consequences.
Where traditional consequentialism falls short, and why
Traditional consequentialism, as I have summarized it, is an inadequate guide for decision making in scenarios that meet two criteria:
a) One or more of the possible outcomes of the actions available to the agent in the decision scenario involve grave harm.
b) Making probabilistic calculations about the likely outcomes of the actions available to the agent is impossible or near impossible.
I will refer to such problematic scenarios as “inscrutable grave risk scenarios”. The term “grave risk” is meant to capture the possibility of the occurrence of the very grave negative consequences of one or more of the actions available to the agent in a decision-scenario, referenced in criterion a). The definition of “grave risk” that I will use here takes its essence from the second of Sven Ove Hansson’s four definitions of risk: “the cause of an unwanted event which may or may not occur” (Hansson). In other words, for the purposes of this paper, an action will be deemed a risk if it fits the above definition.
An action will be deemed a grave risk to a given society if the subset of that society that will be affected by its potential negative consequences is large enough to be a legitimate cause of concern for any member of that society. For example, the risk of legalizing drunk driving in some city is a grave risk to the inhabitants of that city because such legislation would apply to every driver of every car on every road. Therefore, since everyone will, at some point, use at least a few of those roads, every inhabitant of that city would be justified in feeling afraid for their safety if drunk driving were legalized.
Similarly, detonating an atomic bomb in Times Square at some time over the next year is a grave risk to New Yorkers, since any one of them might be within the affected area at the time of the detonation, given the centrality of the location of Times Square. Conversely, detonating an atomic bomb on Pluto would not be a grave risk to any society on Earth. Of course, by this definition, an action may be a grave risk to one society but not to another. For example, weaponizing smallpox in a laboratory in Indonesia may be a grave risk for Indonesians, but may not even register on the risk radar of Peruvians. For the purposes of this paper, the society in question will be any society in which questions of the ethical design of AI and the regulation of social robots arise.
The “inscrutable” in “inscrutable grave risk scenario” is meant to capture the impossibility of making predictions about the outcomes of the available actions in such scenarios. For example, a decision scenario in which an agent must decide whether or not to rescue a drowning child from a swimming pool when the only other able bodied adult in the vicinity is the child’s grandmother who does not know how to swim is not inscrutable.
If the agent decides to rescue the child, he has very good reason to expect the child to survive. If he does not intervene, he has very good reason to believe that the child will drown. Conversely, if an agent is on the roof of an abandoned building at 3 AM, it is pitch black outside, and there is fog obscuring his view of the ground below, his decision to either throw a brick off the roof or to refrain from doing so is inscrutable. He has no meaningful way of calculating the probability that that brick specific will land on someone’s head, or on a passing vehicle, though he can be sure that not throwing the brick would bring those probabilities down to zero. Inscrutable grave risk scenarios, as mentioned above, are situations in which the action in question is both a grave risk and inscrutable.
While traditional consequentialism may not be an adequate guide for decision making in such scenarios, an adequately altered version of traditional consequentialism can be. Firstly, the version of consequentialism that I will propose will leave the first principle of traditional consequentialism untouched: consequences will retain their status as the unique locus of normative value. The second component of traditional consequentialism will also remain intact for decision scenarios that are not inscrutable grave risk scenarios. In other words, unless a decision scenario meets the two criteria outlined above, the agent could revert to traditional consequentialism as a guide, either by applying some rule of thumb, or by choosing the action that, to the best of her knowledge, will maximize the good according to some probability calculation or some belief about foreseeable consequences.
The reason traditional consequentialism cannot adequately handle inscrutable grave risk scenarios is because it has no mechanism through which to take the consequences of the decisions available to the agent in such scenarios into account. The first principle of traditional consequentialism (and of any consequentialist theory), is that the normative value of an action resides in its consequences. Take the case of the agent deciding whether or not to throw the brick off the roof of a building in the middle of a foggy night. Let us imagine him deciding to throw the brick off the roof and it hitting a passerby on the head, killing her instantly.
A consequentialist must believe that the action of throwing the brick off the roof had some normative value. To deny this assertion would be to deny the value of the victim’s life, or the loss of utility resulting from her death. Now, let us rewind the tape to the moments before the brick was thrown. How would the traditional consequentialist tell the agent to act in the face of the total unpredictability of the consequences of the actions available to him? If the traditional consequentialist cannot advise him one way or the other, she would be admitting that her own moral theory has absolutely nothing to say about a decision that, a mere few seconds later, will prove to have terrible moral consequences by the lights of her own theory. While this is not, strictly speaking, paradoxical, it is deeply unsatisfying.
One might respond that in this particular case, moral intuition or rule consequentialism would solve the problem. One should simply adopt the rule that one ought never to throw bricks off buildings without knowing what lies below. After all, most people understand the basic mechanics of throwing bricks off buildings, and the basic risks associated with doing so. Perhaps one’s moral intuitions would, in fact, save the hypothetical woman’s life. This is a fair critique of my chosen example. But let us consider an abstract case in which the inscrutability were so pronounced that moral intuitions would either radically diverge, or would simply be absent. Now, let us stipulate that the action in question is a grave risk. What should the consequentialist do? Traditional consequentialism cannot answer this question. If we let the inscrutability of the decision scenario in question get arbitrarily large (way larger than that of the brick scenario), and if the consequences of making the wrong choice are grave enough (much graver than the one death in my example), no pre-existing rule will be able to guide the moral agent, since if such a rule already existed, the scenario would not be inscrutable. Such situations are admittedly rare, but as I will now show, the design of ethical AI and the regulation of the treatment of social robots are such scenarios.
Case study: Decision scenarios that will arise in the design of self-driving cars
To illustrate this point, let us first examine some decision scenarios that will inevitably arise in the design of self-driving cars. The design of autonomous vehicles is an illustrative example of the inability of traditional consequentialism to deal with questions arising out of the attempt to design ethical AI. In this case, the agents in question are the designers of such cars. As Awad et al. point out in The Moral Machine Experiment, “autonomous vehicles will cruise our roads soon, necessitating agreement on the principles that should apply when, inevitably, life-threatening dilemmas emerge” (Awad et al. 59). In the rest of their article, the authors report their findings on social expectations of how autonomous vehicles should resolve such moral dilemmas.
According to the results of their global survey, “the strongest preferences are observed for sparing humans over animals, sparing more lives, and sparing young lives” (60). While these results vary slightly with culture (for example, cultures that are more collectivist show less of a preference for younger lives than more individualistic cultures), there is surprisingly widespread agreement about those basic principles (62). Evidently, such basic principles are not enough to go on when deciding how to program an autonomous motor vehicle, because not all moral dilemmas are straightforward choices between sparing the life of a young person or that of an old person, or between sparing more lives or less lives. However, such simple examples are sufficient to illustrate why traditional consequentialism does not have the tools to deal with certain important questions in the design of ethical AI.
According to Tettamanti et al., self driving cars have the potential to save 30 000 lives per decade in the United States alone, due to a reduction in traffic accidents (Tettamanti et al. 249). This fact is crucial to the position I am advancing here, since it leaves no room for doubt that, from a consequentialist point of view, it is morally desirable for self-driving vehicles to go into widespread use. Clearly, as mentioned above, according to the moral intuitions of a significant proportion of the world’s population, self-driving cars should be programmed along broadly consequentialist lines, at least as it pertains to the moral preference for saving more rather than less lives.
Presumably, the designers of the autonomous vehicles share this widely held moral intuition. Thus, according to traditional consequentialism, there is simply no room for doubt that autonomous vehicles should be programmed along consequentialist lines. After all, whether through a straightforward utilitarian calculus, or whether through the following of our moral intuitions, it would seem that programming self-driving cars any other way would be a straightforward violation of consequentialism, and would require some moral principle other than a concern for consequences for it to be justified.
This conclusion is problematic, however. From the perspective of an average consumer, given a trolley problem-like scenario in which an autonomous vehicle had the “choice” to either swerve into a crowd of pedestrians and save its passenger, or kill the passenger and save the pedestrians, the former choice would be preferable. Granted, this assertion is not supported by empirical evidence, but the hypothesis that consumers would be more reluctant to purchase a vehicle that would intentionally sacrifice their own lives seems, if not certain, then highly plausible. The obvious question then is: would the knowledge that self-driving vehicles were programmed along consequentialist lines lead to less purchases of such vehicles overall, thus resulting in a very high number of preventable traffic deaths?
If the answer to this question is yes, then the consequentialist should be in favour of programming autonomous vehicles that save the driver’s life at all costs, since doing so would result in more purchases of autonomous vehicles, thus resulting in more lives saved overall. The problem, however, is that we simply do not know the answer to this question.
This ignorance, along with the thousands, possibly millions, of lives that are at stake, are what make the decision on how to program self-driving cars an inscrutable grave risk scenario. Firstly, any society in which thousands of people die every year in unnecessary traffic accidents is a society in which anyone has good reason to fear being one of them. Secondly, while all consequentialists agree on the desirability of saving as many lives as possible, we simply do not know whether the optimal path to this result is designing consequentialist autonomous vehicles, or passenger-saving autonomous vehicles. The consequences of either of these choices are utterly unpredictable, and the upshot of making the wrong choice is thousands, possibly millions, of lives lost. Moral intuitions are of no help, since this decision scenario is brand new, and moral intuitions about it are bound to diverge radically. Rules of thumb will be of no help either. What pre-established moral rule can possibly help us decide how to design autonomous vehicles to maximize the number of purchases thereof? Traditional consequentialism is clearly ill-equipped to deal with this risk.
Other inscrutable grave risk scenarios where traditional consequentialism is the wrong tool
This insight extends beyond the realm of designing autonomous vehicles. While some decision-scenarios in AI design will surely not be inscrutable grave risk scenarios (the construction of machine-learning algorithms that play chess or recommend medical treatments, for example), many surely will be. For example, until we have some way of understanding the possible constitutions of the minds of Artificial General Intelligences (AGI’s) and their possible motivations, traditional consequentialism will not be able to guide us in making decisions about how to design them, or even whether or not to design them.
Such scenarios might be the gravest of inscrutable grave risk scenarios, since their potential negative consequences, as Chalmers argues, can lead to the extinction of the human species via an intelligence explosion (Chalmers 33). My assertion here is thus not that traditional consequentialism cannot, in principle, give any meaningful guidelines regarding the design of AI in general. Rather, it is that there are multiple plausible decision scenarios that have arisen and that may arise in the future in the design of AI that constitute inscrutable grave risk scenarios for which traditional consequentialism will be an inadequate decision guide.
Further, traditional consequentialism is ill-equipped to deal with decisions relating to the societal regulation of the treatment of social robots. In her paper Extending Legal Protection to Social Robots: The Effects of Anthropomorphism, Empathy, and Violent Behaviour Towards Robotic Objects, Kate Darling makes the case that society should legally prevent people from mistreating such robots to “help discourage human behaviour that would be harmful in other contexts” (Darling 214). Her argument rests on the fact that what she calls “social robots” “are designed to act as our companions” (216).
Social robots are thus generally given three important properties: physicality (the property of having a physical body), perceived autonomous movement and, most importantly, social behaviour, or the ability to “mimic cues that we automatically, even subconsciously, associate with certain states of mind or feelings” (217-18). As a result, Darling argues, frequent interactions between humans and social robots have the potential to create strong social and emotional bonds between people and their robot companions, perhaps even creating illusions of “mutual relating” (219).
Consequently, studies cited by Darling show that a significant number of people are deeply offended by the “abuse” of social robots (223). Darling thus suggests that banning certain abusive behaviours toward social robots might be warranted. She argues that given the “lifelike behaviour” of social robots (behaviour which will surely become more and more realistic over time), it makes sense for parents to discourage their children from mistreating these robots, since it might become more and more difficult for children to distinguish them from live animals (224). Darling extends this line of reasoning to adult humans, suggesting that “the difference between alive and lifelike may be muddled enough in our subconscious to warrant adopting the same attitudes toward robotic companions that we carry toward our pets” (224).
Crucially, she argues that more empirical data are needed to determine whether this muddling would actually take place. However, if research did in fact show that human minds did not make clear distinctions between live organisms and lifelike machines, then regulation of the treatment of social robots would surely be warranted (224).
As was the case in the design of autonomous vehicles, the decision on whether or not to allow people to mistreat social robots is an inscrutable grave risk scenario. In this scenario, the agents in question are policymakers. As Darling points out, we simply do not know whether or not the mistreatment of social robots would actually lead to widespread desensitization to human suffering. We can be reasonably certain, however, that a society that undergoes such widespread desensitization would be worse at alleviating suffering and taking steps to prevent it than a society in which the desensitization had not taken place.
Society’s decision on how to regulate the treatment of social robots thus clearly fits my definition of grave risk: any member of a society in which human suffering was devalued would be justified in feeling concerned about the devaluation of her own suffering. Such a state of affairs is thus clearly undesirable from a consequentialist point of view. That being the case, traditional consequentialism should have the tools to be able to guide consequentialist policymakers in their decisions on how to regulate the treatment of social robots, and yet it does not.
As was the case with the design of autonomous vehicles, the future consequences of deciding to either regulate or not regulate the treatment of social robots are utterly unpredictable. Social robots are infant technologies, and we do not yet have the tools to test the effects of their mistreatment on societies. It would therefore be meaningless to talk about the foreseeable or probable consequences of deciding whether or not to regulate them.
Moral intuitions will be of no help either, since there will be a radical divergence of intuition on this question. Some, like Deborah G. Johnson and Mario Verdicchio, might contend that in the absence of empirical evidence to support the claim that the mistreatment of social robots would lead to desensitization to human suffering, regulation is unnecessary (Johnson and Verdicchio 298).
Further, they might argue that bringing our standards of treatment of social robots in line with our standards of treatment of animals, as Darling suggests, is not warranted, since animals can suffer and robots cannot (292). Others might agree with Darling, and argue that social robots should be treated like pets. Still others might argue that social robots should be treated like people.
Clearly, there is no moral intuition upon which all can agree in this decision situation. Rules of thumb will be of no help either. We simply do not yet have a set of moral rules that tell us how society should treat lifelike, but unconscious machines that feel to us uncannily like human beings or non-human animals. Once again, traditional consequentialism is clearly ill-equipped to help us deal with this inscrutable grave risk scenario.
A modified version of consequentialism that can accommodate ignorance of the future and the consequences of grave risk
While traditional consequentialism is incapable of serving as a guide to decision-making in some decision scenarios regarding the design of ethical AI and the regulation of the treatment of social robots, a version of consequentialism that can accommodate ignorance of the future while still being able to factor in the consequences of grave risks will be capable of doing so. I will call such an updated theory “risk consequentialism”. Risk consequentialism holds that the primary ethical concern that should inform and guide agents in inscrutable grave risk scenarios, including both the design of AI systems and society’s regulation of the treatment of social robots, is the mitigation of grave risk.
In other words, in inscrutable grave risk scenarios, the risk consequentialist will prioritize the avoidance of the worst possible outcome and the resulting severe loss of utility. This prescription does not require any knowledge of probabilities, since it does not require the agent to know with any degree of certainty that action A will prevent the worst possible outcome whereas action B will not. Rather, it demands that the agent choose that action that seems the least likely to lead to that outcome, even if doing so goes against his moral intuition.
This prescription follows straightforwardly from the first principle of all consequentialist moral theories, namely that the moral status of actions depends solely on those actions’ consequences. If the consequentialist agent places moral value on all consequences, then she must find some way of incorporating unpredictable consequences into her decision procedure, especially if they result in great loss of utility, as they always do in the worse possible outcomes of inscrutable grave risk scenarios. The only way of placing value on the possible consequences of the actions available to the agent in inscrutable grave risk scenarios is to make the strongest possible attempt to avoid the worst possible outcome, even in the absence of probabilistic knowledge. Not doing so would be opening the door to severe loss of utility without good reason, and would thus be a devaluation of that loss.
In the case of the design of autonomous vehicles, the agents in question understand the loss of life that will result from consumers being reluctant to purchase self-driving cars. They have good reason to believe that this worst-case scenario will have a higher chance of occurring if consumers believe that their cars would willingly sacrifice their passengers’ lives, though they do not know this for sure. Again, even in the absence of probabilistic knowledge, any reasonable person could be forgiven for having a strong intuition that consumers are less likely to purchase an autonomous motor vehicle that would intentionally kill them.
Therefore, as risk consequentialists, they should design the cars to protect the passengers’ lives at all costs to incentivize their purchase, thereby saving more lives. This prescription is not based on any probability calculation. It is, at best, an educated guess, but it at least makes an attempt to mitigate the grave risk of thousands of needless traffic accidents. This attempt is better than what traditional consequentialism could have produced, since traditional consequentialism has no meaningful way of even talking about this scenario.
Of course, this prescription is subject to change in the face of new evidence. For example, if it were discovered that consumers actually had no preference for vehicles that were programmed to save their passengers’ lives at all costs over consequentialist vehicles, then the decision scenario in question would no longer be inscrutable, and traditional consequentialism could serve as an adequate decision-guide.
A second important caveat to the above prescription is that the decision to mitigate future grave risk cannot have any side effects that cancel out the aforementioned utility gain. For example, if it were discovered that non-consequentialist autonomous vehicles would actually kill more people than they would save, then again, the decision scenario would no longer be inscrutable, because the agents in question would have some knowledge about the foreseeable consequences of one of their choices. The prescription to mitigate risk thus only applies in situations in which doing so would have no negative consequences that outweigh the negative consequences whose probability of occurrence risk consequentialism would attempt to minimize.
How risk consequentialism can help guide policy decisions
Risk consequentialism can also serve as a decision guide for policymakers in deciding how to regulate the mistreatment of social robots. As mentioned above, Darling tacitly concedes that her argument could, in principle, be rendered moot if research showed that the mistreatment of lifelike machines like social robots had no impact on human behaviour toward live organisms. This admission opens her argument to the objection that because, by her own admission, it is not based on empirical evidence, it should not be taken seriously.
One might argue that if we have no reason whatsoever to believe that the mistreatment of social robots will reliably lead to the mistreatment of sentient beings, there is no reason to prevent the mistreatment of machines that cannot even suffer (unless, of course, such evidence does emerge). In fact, it is not even clear that “mistreatment” is the right word to use. After all, we do not talk of mistreating PlayStations and microwaves. This argument is consistent with traditional consequentialism.
This objection could have been dealt with in advance if Darling had rested her argument more heavily on the desire to mitigate the risk of desensitization. Under this risk consequentialist version of her argument, the empirical data that we already have are sufficient to warrant the regulation of our treatment of social robots, because they suggest that there is at least a possibility that people who mistreat social robots would, in fact, experience some form of desensitization to the suffering of sentient beings.
Numerous case studies referenced by Darling in her article show that “we form emotional attachments to robots that are surprisingly strong” (Darling 216). A telling example is the case of a military robot shaped like a stick insect that diffuses landmines by stepping on them. As Darling recounts in her paper, the soldiers who trained with the robot were very uncomfortable watching it work, even going so far as calling its use by the military “inhumane” (217). This case and others like it suggest that there is some similarity at the level of our emotional attachments between the way we relate to sentient beings and the way we relate to social robots, even those that do not mimic humans.
If this similarity extends to the way in which our perceptions of social robots would change in a context of personal or societal mistreatment of them, then it is a very reasonable hypothesis that desensitization to the mistreatment of social robots would extend to desensitization to the mistreatment of other beings with whom we relate to in similar ways, including humans. Thus, Darling could have argued that given the seriousness of the risk associated with widespread desensitization to mistreatment (after all, we might, at some point in the future, be interacting with social robots on a daily basis), we should regulate their mistreatment until evidence emerges that there is an important difference between the way we perceive the mimicked suffering of robots and the actual suffering of sentient beings, and that this difference eliminated the risk of desensitization to the mistreatment of those beings. This argument, more satisfying from a consequentialist perspective, follows directly from the principle of the minimization of risk.
Conclusion
In summary, traditional consequentialism is ill-equipped to deal with what I have called inscrutable grave risk scenarios. The alternative that I have proposed, risk consequentialism, holds that the primary ethical concern that should inform and guide agents in inscrutable grave risk scenarios, including both the design of AI systems and society’s regulation of the treatment of social robots, is the mitigation of grave risk. In inscrutable grave risk scenarios, the risk consequentialist will seek to minimize the probability of the occurrence of the worst possible outcome and the resulting severe loss of utility. In the case of the design of autonomous vehicles, the risk consequentialist should seek to maximize purchases, even if this means designing the vehicles to save the passenger’s life at all costs. In the case of the regulation of social robots, in the absence of evidence on whether or not the mistreatment of social robots will lead to desensitization to human suffering, risk consequentialist regulators should ban the mistreatment of social robots anyway, since this will minimize the risk of such mass desensitization.
References
Awad, Edmond et al. (2018). The Moral Machine Experiment. Nature. 563, pp. 59–64.
Chalmers, David (2010). The Singularity: A Philosophical Analysis. Journal of Consciousness Studies, 17(9-10) Excerpt, pp. 1–15 & 19–56.
Darling, Kate (2016). Extending Legal Protection to Social Robots: The Effects of Anthropomorphism, Empathy, and Violent Behavior Towards Robotic Objects. In R. Calo, M. Froomkin, & I. Kerr (eds.), Robot Law. Edward Elger, pp. 213-233.
Hansson, Sven Ove, “Risk”, The Stanford Encyclopedia of Philosophy (Fall 2018 Edition), Edward N. Zalta (ed.), URL = <https://plato.stanford.edu/archives/fall2018/entries/risk>.
Johnson, Deborah G. & Mario Verdicchio (2018). Why Robots Should Not Be Treated Like Animals. Ethics and Information Technology, 20(4) pp. 291–301.
Sinnott-Armstrong, Walter, “Consequentialism”, The Stanford Encyclopedia of Philosophy (Winter 2015 Edition), Edward N. Zalta (ed.), URL = <https://plato.stanford.edu/archives/win2015/entries/consequentialism/>.
Tettamanti, T., Varga, I. and Szalay, Z. (2016) “Impacts of Autonomous Cars from a Traffic Engineering Perspective”, Periodica Polytechnica Transportation Engineering, 44(4), pp. 244-250. doi: https://doi.org/10.3311/PPtr.9464.