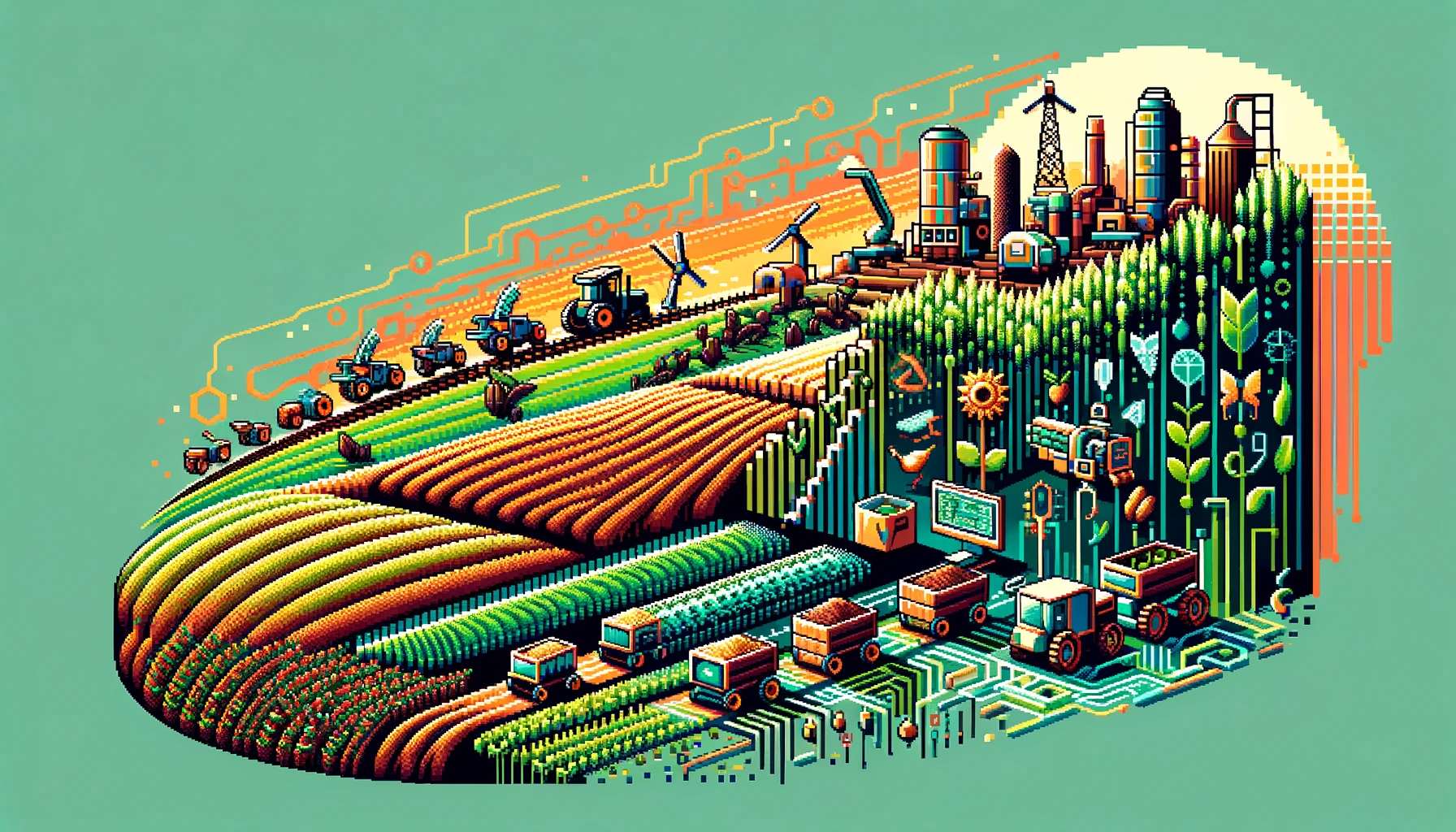
🔬 Original article by Aixa Lacroix from Encode Justice Canada
This is a part of our Recess series in which university students from across Canada briefly explain key concepts in AI that young people should know about: specifically, what AI does, how it works, and what it means for you. The writers are members of Encode Justice Canada, a student-led advocacy organization dedicated to including Canadian youth in the essential conversations about the future of AI.
Introduction
Agriculture, one of the world’s oldest and most vital industries, has been a fundamental part of human civilization since approximately 23,000 BCE, which marked the beginnings of plant cultivation [1]. Today, as the global population surges, so does the demand for food, propelling agriculture into a new era of challenges and innovation. Historically reliant on extensive manual labor, the sector is increasingly presented with novel solutions in response to the climate crisis, stricter environmental legislation, labor shortages, and a growing global population [2]. The emergence of new digital technologies prompts a crucial question: can Artificial Intelligence (AI) carve out a deserving and promising niche in traditional industries like agriculture? While the primary focus of this article is on the United States, it recognizes that the challenges faced by the agricultural industry and the equitable implementation of AI are indeed global concerns.
A Century of Transformation: Technology in Modern Agriculture
The shift from labor-intensive methods in the early 20th century to a technology-driven approach has dramatically reshaped agriculture in the United States. This transformation is evident in the workforce dynamics: while agriculture once absorbed a substantial portion of the labor force, only 1.2% of the U.S. workforce was engaged in farming as of 2022 [3]. Notwithstanding the shift in employment, technological advancements have led to a remarkable increase in productivity; the United States Department of Agriculture (USDA) reported that in 2021, U.S. farm output was almost triple that of 1948 [4].
The USDA attributes this sustained increase in agricultural productivity to technical changes propelled by research and development (R&D) from both public and private sectors [4]. Amidst these advancements, AI is recognized as a potential game-changer, with the capacity to significantly transform agricultural practices. The Food and Agriculture Organization of the United Nations (FAO) spotlights three pivotal areas where AI is emerging in farming: (1) agricultural robotics, (2) soil and crop monitoring, and (3) predictive analytics [5]. These domains, each with its array of potential applications, represent the emerging frontiers where AI might redefine the pathways to agricultural efficiency and sustainability, albeit with an understanding of the challenges and complexities involved.
AI In Practice: Real-World Applications
Agricultural Robotics
In the realm of agricultural robotics, AI-driven automation holds the potential to redefine traditional farming methods. In crop production, autonomous machines such as weeding robots are starting to be commercialized, while drones can gather information for both crop management and input application [6]. Although the precise spread of these advancing technologies is still becoming clear, recent investments have catalyzed the shift from theoretical blueprints to concrete realities. Take, for instance, Carbon Robotics, a US leader in AI-powered robotics that builds empowering and innovative agriculture tools for farmers. In February 2022, Carbon Robotics launched the first and only commercial laser-weeding robot, which currently serves over a dozen farms across the country [7]. Their deep-learning technology enables precision weed control for large-scale specialty crops. It is powered by high-resolution cameras and modern computing that detect weeds and crops in real-time to boost crop yield, cut farming costs, and support sustainable farming [8]. These robotic systems mitigate the need for manual labor and empower farmers to manage larger areas with improved effectiveness. Carbon Robotics is just one of many startups emerging in the space, driving the commercialization of state-of-the-art AI developments. While mechanization is not a novel concept, the impact of startups like Carbon Robots underscores AI’s potential in transforming agricultural practices, particularly as new competition emerges and the cost of technological development gradually decreases.
Soil and Crop Monitoring
Beyond robotics, soil and crop monitoring represent another significant area where AI can impact the agricultural industry. Leveraging data sets, AI algorithms can offer critical insights into key variables like soil moisture and early detection of plant diseases or pest infestations. Highlighting the urgency of sustainable resource management, the World Economic Forum (WEF) notes that twice as much water will be required for food production in 2050, with nearly one-third of agricultural production today taking place in water-stressed regions [9]. Within the United States, the USDA’s Drought Monitor indicates that approximately 55% of rice production is within an area experiencing drought as of January 2024 [10]. Thus, the need for resource-optimization solutions in light of factors like climate change is clear. Field trials have shown that precision irrigation, which enables the variation of water input using sensor measurements across the farm at a fine granularity, can increase farm productivity by as much as 45% while reducing the water intake by 35%. Techniques to vary other farm inputs like seeds and soil nutrients have also proven beneficial [11].
A notable advancement in crop monitoring is evidenced by a 2017 Cornell University study, which used a technique called ‘transfer learning’ for AI-based image recognition to detect diseases in cassava plants, the third-largest source of carbohydrates for human food [12]. The study achieved an impressive accuracy rate of 95-98% for identifying various pests, utilizing just 2,756 images—a stark contrast to traditional neural networks that require millions of images for training. This innovative approach highlights a fast, affordable, and easily deployable digital plant disease detection strategy, requiring only a smartphone with a pre-installed app. This technology signifies a significant stride toward reducing barriers to technology adoption, particularly as farmers across the globe could leverage this type of technology without internet access.
Furthermore, a systematic review of the impact of computer vision and AI on the production of key grain crops (corn, rice, wheat, soy, and barley) revealed substantial enhancements across various metrics, notably in pest identification and disease detection. For example, identifying aphids—small sap-sucking insects on wheat that notoriously cause significant crop damage and disease transmission—was markedly improved through a digital image-based system utilizing support vector machines. Researchers achieved an identification accuracy of 86.81% and an error rate of 8.91%, surpassing the effectiveness of the other methods examined [13]. This advancement in pest detection is particularly impactful, considering that grains constitute over half of the global caloric intake, underscoring AI’s potential scale and significance in enhancing grain crop monitoring [14].
Predictive Analytics
Predictive analytics, a fundamental component of precision farming (PF), stands as a final stand-out transformative force in agriculture. This approach harnesses historical information, current environmental conditions, and other data to forecast crop yields, evaluate risks, and offer strategic farming recommendations [15]. Such predictive insights are invaluable, enabling farmers to make informed decisions regarding planting, harvesting, and market planning, thereby optimizing the agricultural supply chain’s efficiency and profitability [16].
Reflecting the growing adoption of PF, the USDA’s Agricultural Resource Management Survey indicated that between 30 and 50 percent of U.S. corn and soybean acres utilized PF technologies during 2010-12, a figure that has likely increased since. Private enterprises are playing a pivotal role in this shift towards data-driven agriculture, with one such example being OneSoil, a Swiss-based company founded in 2017. OneSoil claims that 4.5% of the world’s arable land across 57 countries is registered on their platform [17]. Their mission to enhance the profitability and sustainability of farmers and agricultural companies through satellite imagery and field analytics demonstrates agriculture’s dynamic, ongoing transition into a more digitized era.
Sowing Innovation: from Nascent Potential to Future Possibilities
The promise of AI in agriculture shines bright, yet the journey from theoretical potential to widespread adoption in the field is fraught with complexity. If academic studies paint a picture of immense potential, why has AI not yet been widely adopted in the agricultural industry?
From an academic standpoint, one clear barrier – notably, not one unique to agriculture – is that while some AI-based models perform well under controlled settings, they fail to produce satisfactory results in real-time scenarios [18]. Moreover, a 2023 publication assessing the trends in deep learning techniques for plant pathology exposes the critical need for a concise dataset to improve the performance and accuracy of deep learning algorithms [18]. Intriguingly, the same study advocates for a shift towards transfer learning methods, echoing a stance that Cornell University put forward nearly seven years prior. This evinces the early stage of current research as both convolutional neural network (CNN) models, known for their proficiency in analyzing visual imagery by mimicking the human visual cortex, and transfer learning continue being studied.
Broadly, while academic research opens new optimistic horizons of understanding, these insights often remain confined to journal pages, untouched by the hands of those working in the field. A stark gap exists in the literature: comprehensive review papers that holistically address AI’s application in agriculture, considering the sector’s multifaceted stages, such as cultivation, monitoring, and harvesting, are notably absent [19]. This leads to another formidable obstacle: market adoption. The introduction of AI-driven tools—be it robots or sophisticated software—necessitates time for development, refinement, and acceptance within the intricate web of agricultural companies and workers along the supply chain.
However, analyzing the industry landscape reveals that it is not just startups advancing the development of AI in agriculture. Major players like Microsoft, Google, and IBM also make their mark with various projects [20, 21, 22]. In 2017, Microsoft introduced FarmBeats, aiming to democratize AI for farmers globally by facilitating data-driven farming through low-cost sensors, drones, and vision and machine learning algorithms. FarmBeats later transitioned into a business-to-business offering on Azure Marketplace, Microsoft’s platform for solutions from independent software vendors. Although FarmBeats was retired in September 2023, its legacy continues with the Azure Data Manager for Agriculture, a revamped, sophisticated version currently in preview. As observers at this pivotal moment, we have the privilege of witnessing AI’s evolution in agriculture—a journey that encompasses academic research, the development of applications, and the very gradual embrace of these innovations in the market. Both small startups and established tech giants are at the forefront, participating in the early stages of AI’s potential integration into the agricultural landscape. This is also where the role of governance and policy becomes critical, as it shapes the trajectory of AI deployment. Addressing the challenges in data management and investment in AI by governmental bodies is, therefore, pivotal for advancing and accepting AI-driven solutions in agriculture, ensuring that the promise of these technologies is fully realized in practice.
Navigating Crossroads: The Role of Government in Data Management and AI Investment
Evaluating the current state of agricultural AI reveals a crucial intersection of technology and policy. In a landmark move in 2021, the U.S. Department of Agriculture’s National Institute of Food and Agriculture (USDA-NIFA), alongside the U.S. National Science Foundation (NSF) announced a substantial $220 million for AI Research Institutes [23]. Yet, the absence of the term ‘artificial intelligence’ in NIFA’s Strategic Plan for 2022-2026, unveiled in September 2022, raises eyebrows, especially given their commitment to “refine and define measures for the implementation strategies in each objective” [24]. Moreover, the USDA, adhering to Executive Order (13960) from December 8, 2020, compiled an inventory detailing its current and planned AI applications in alignment with the agency’s mission. This compilation, last refreshed in May 2023, presents a mere 39 use cases, with scant details provided [25].
Scrutinizing the recent USDA-NIFA awards intensifies these concerns. A search for ‘artificial intelligence’ yields a notable $10,000,000 award to the University of California under the “Agriculture and Food Research Initiative” (AFRI) program area [26]. Yet, the organization’s 2020 Annual Review falls short of shedding light on the ramifications of this substantial investment, particularly regarding clearly quantified or qualified impact [27]. This opacity, likely a byproduct of imprecise performance reporting, is particularly troubling when the outcomes of such significant investments remain ambiguous, even years post-announcement.
This prevalent information void poses a true barrier to comprehending the influence of government-endorsed AI technologies. For AI to truly be a catalyst in the agricultural sector, it must seamlessly integrate into the existing systems and infrastructures workers utilize across the agricultural supply chain. This integration is crucial to addressing resource limitations, addressing escalating market demands, and navigating challenging climatic conditions. Initiatives could start with promoting open-access research and embracing transparent record-keeping methods in contrast to current practices. The government must proactively regulate both public and private AI advancements, steering them towards a trajectory that amplifies positive societal impacts.
Conclusion
The development of inclusive, accessible AI technology is crucial in addressing the myriad challenges the agricultural sector faces. Concurrently, the importance of regulations governing the transparent use of AI and agricultural data cannot be overstated – particularly as AI-powered agricultural technology continues growing from its current stage. Organizations like the FAO and USDA-NIFA have committed to developing AI solutions that support sustainable, positive outcomes. Still, as of today, there remains a lack of clear, systematic review and quantification of their impact.
As AI is expected to play an increasingly critical role in adapting agriculture to the challenges of climate change, it is essential for key stakeholders – including government bodies and leading private organizations – to consider current limitations in research and real-world applications of this domain. The future of agriculture with AI promises increased efficiency and sustainability – an ambitious goal that we are seeing the beginnings of today and whose future is yet to be determined.
Sources
[1] Snir, A., Nadel, D., Groman-Yaroslavski, I., Melamed, Y., Sternberg, M., Bar-Yosef, O., & Weiss, E. (2015). The Origin of Cultivation and Proto-Weeds, Long Before Neolithic Farming. PLoS ONE, 10(7). https://doi.org/10.1371/journal.pone.0131422
[2] Javaid, M., Haleem, A., Khan, I. H., & Suman, R. (2023). Understanding the potential applications of Artificial Intelligence in Agriculture Sector. Advanced Agrochem, 2(1), 15-30. https://doi.org/10.1016/j.aac.2022.10.001
[3] U.S. Department of Agriculture: Economic Research Service. (2024, January 12). Ag and Food Sectors and the Economy. USDA ERS. https://www.ers.usda.gov/data-products/ag-and-food-statistics-charting-the-essentials/ag-and-food-sectors-and-the-economy/?topicId=b7a1aba0-7059-4feb-a84c-b2fd1f0db6a3#:~:text=Agriculture%20and%20its%20related%20industries,percent%20of%20total%20U.S.%20employment
[4] U.S. Department of Agriculture: Economic Research Service (2024, January 12). Productivity Growth in U.S. Agriculture (1948–2021). USDA ERS. https://www.ers.usda.gov/data-products/agricultural-productivity-in-the-u-s/productivity-growth-in-u-s-agriculture/
[5] Mayer, P. (2020, September 24). Artificial Intelligence best-practices in agriculture can help bridge the digital divide while tackling food insecurity. Food and Agriculture Organization of the United Nations (FAO). https://www.fao.org/newsroom/detail/Artificial-Intelligence-best-practices-in-agriculture-can-help-bridge-the-digital-divide-while-tackling-food-insecurity/en#:~:text=24%20September%202020%2C%20Rome%20,achieve%20food%20and%20nutrition%20security
[6] Food and Agriculture Organization of the United Nations (FAO) (2022). In Brief to The State of Food and Agriculture 2022. Leveraging automation in agriculture for transforming agrifood systems. FAO. https://www.fao.org/3/cc2459en/cc2459en.pdf
[7] International Agri-Center (n.d.). Carbon Robotics LaserWeeder. World Ag Expo. Retrieved January 1, 2024, from https://wae23.mapyourshow.com/8_0/exhibitor/exhibitor-details.cfm?exhid=Carbon
[8] Carbon Robotics (n.d.). LaserWeeder Implement: Precision weed control for large-scale specialty crops. Retrieved January 1, 2024, from https://carbonrobotics.com/laserweeder
[9] World Economic Forum (2018, January). Innovation with a Purpose: The role of technology innovation in accelerating food systems transformation. WEF. https://www3.weforum.org/docs/WEF_Innovation_with_a_Purpose_VF-reduced.pdf
[10] United States Department of Agriculture (2024, January 16). Ag in Drought. U.S. Drought Monitor. https://www.usda.gov/sites/default/files/documents/AgInDrought.pdf
[11] Vasisht, Deepak & Kapetanovic, Zerina & Won, Jongho & Jin, XinXin & Chandra, Ranveer & Sinha, Sudipta & Sudarshan, Madhusudan & Stratman, Sean. (2017). FarmBeats: An IoT Platform for Data-Driven Agriculture. https://www.microsoft.com/en-us/research/wp-content/uploads/2017/03/FarmBeats-webpage-1.pdf
[12] Ramcharan, A., Baranowski, K., McCloskey, P., Ahmed, B., Legg, J., & Hughes, D. (2017). Using Transfer Learning for Image-Based Cassava Disease Detection. Frontiers in Plant Science, 8, 1852. https://doi.org/10.48550/arXiv.1707.03717
[13] Patrício, D. I., & Rieder, R. (2018). Computer vision and artificial intelligence in precision agriculture for grain crops: A systematic review. Computers and Electronics in Agriculture, 153, 69-81. https://doi.org/10.1016/j.compag.2018.08.001
[14] Awika, J. M. (2011). Advances in Cereal Science: Implications to Food Processing and Health Promotion. American Chemical Society, 1-13. https://doi.org/10.1021/bk-2011-1089.ch001
[15] Eli-Chukwu, N. C. (2019). Applications of Artificial Intelligence in Agriculture: A Review. Engineering, Technology & Applied Science Research, 9(4), 4377–4383. https://doi.org/10.48084/etasr.2756
[16] A. Sharma, A. Jain, P. Gupta and V. Chowdary (2020). Machine Learning Applications for Precision Agriculture: A Comprehensive Review. IEEE Access, vol. 9, pp. 4843-4873, 2021, https://doi.org/10.1109/ACCESS.2020.3048415.
[17] (n.d.). About OneSoil. OneSoil. Retrieved January 1, 2024, from https://onesoil.ai/en/about
[18] Salman, Z., Muhammad, A., Piran, M. J., & Han, D. (2023). Crop-saving with AI: Latest trends in deep learning techniques for plant pathology. Frontiers in Plant Science, 14. https://doi.org/10.3389/fpls.2023.1224709
[19] Wakchaure, M., Patle, B., & Mahindrakar, A. (2023). Application of AI techniques and robotics in agriculture: A review. Artificial Intelligence in the Life Sciences, 3, 100057. https://doi.org/10.1016/j.ailsci.2023.100057
[20] Microsoft (n.d.). FarmBeats. Microsoft Garage. Retrieved January 5, 2024, from https://www.microsoft.com/en-us/garage/wall-of-fame/farmbeats/
[21] Google (2023, October 19). How farmers are using AI in the field. Google Public Policy. Retrieved January 5, 2024, from https://publicpolicy.google/article/wadhwaniai-ai-farmers-agriculture-food-waste/
[22] IBM (n.d.). IFarming. Retrieved January 5, 2024, from https://www.ibm.com/case-studies/ifarming-watson-cloud
[23] U.S. Department of Agriculture’s National Institute of Food and Agriculture (USDA-NIFA) (2021, July 19). USDA-NIFA and NSF Invest $220M in Artificial Intelligence Research Institutes. USDA-NIFA. https://www.ibm.com/case-studies/ifarming-watson-cloud
[24] U.S. Department of Agriculture’s National Institute of Food and Agriculture (USDA-NIFA) (2022, September). NIFA Strategic Plan 2022-2026. USDA-NIFA. https://www.nifa.usda.gov/sites/default/files/2023-01/NIFAStrategicPlan_22-26_0123.pdf
[25] U.S. Department of Agriculture (USDA) (n.d.). Inventory of USDA Artificial Intelligence Use Cases. USDA. https://www.usda.gov/data/AI_Inventory
[26] U.S. Department of Agriculture’s National Institute of Food and Agriculture (USDA-NIFA) (n.d.). Recent Awards. USDA-NIFA. https://portal.nifa.usda.gov/lmd4/recent_awards?report_title=Recent%20Awards&from_site=NIFA&search_label=Awards%20Listing
[27] U.S. Department of Agriculture’s National Institute of Food and Agriculture (USDA-NIFA) (n.d.). AFRI 2020 Annual Review. USDA-NIFA. https://www.nifa.usda.gov/afri-2020-annual-review