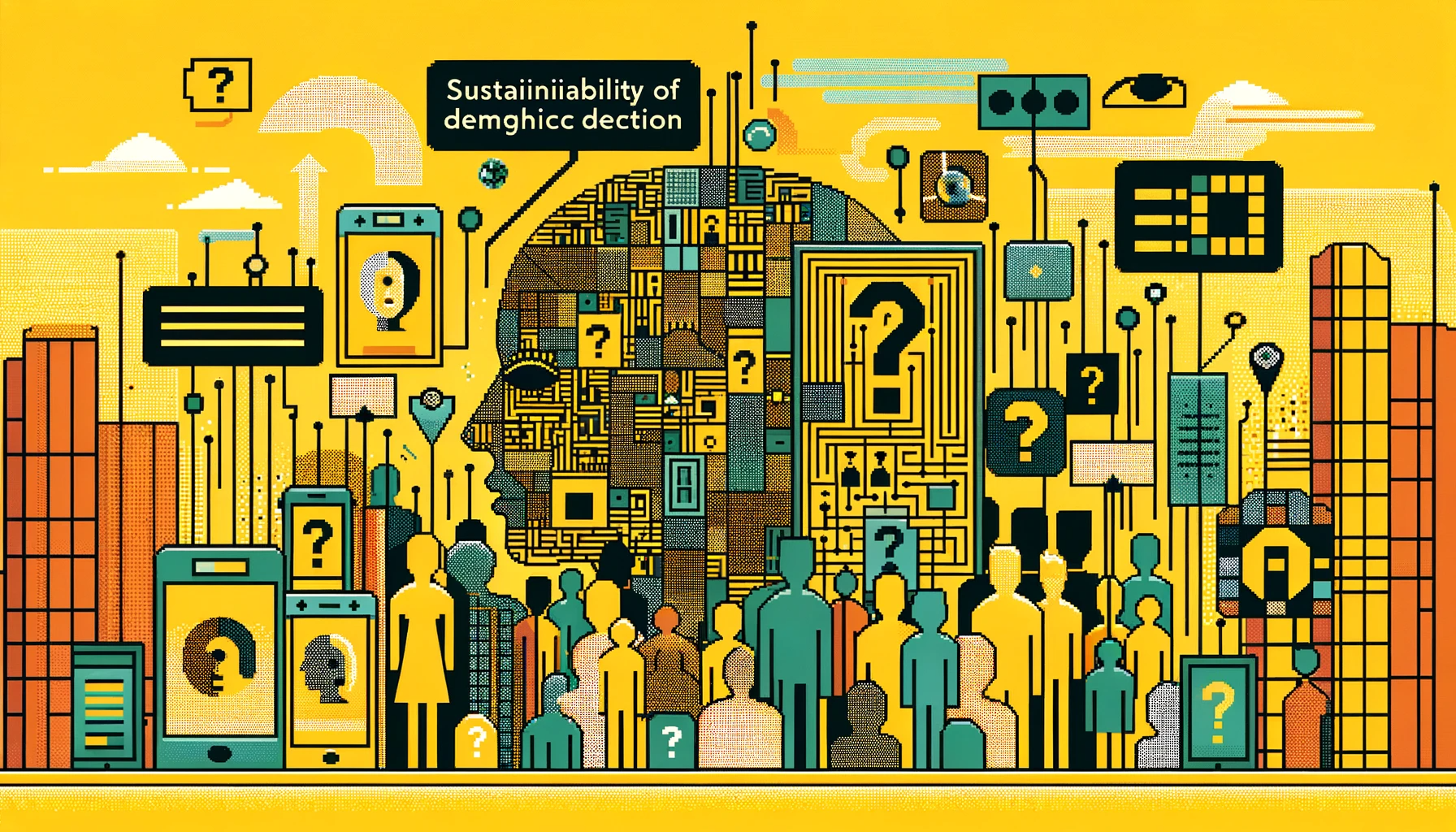
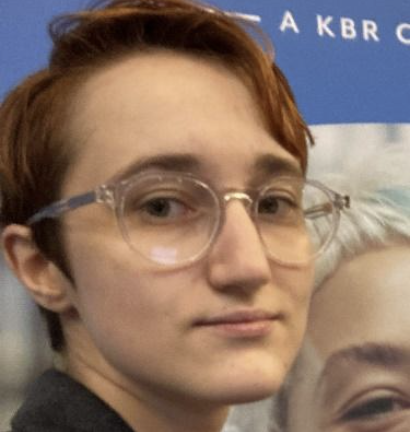
🔬 Research Summary by Beckett LeClair, a Senior Engineer at Frazer-Nash Consultancy and vocal proponent for ethical AI futures.
[Original paper by Beckett LeClair, William Parker, and Amanda Young]
Overview: Is demographic detection AI unsustainable in its current form? We investigated some potential use cases alongside the consequences that may result. Our work raised some interesting questions surrounding the sustainability of the technology, particularly in the face of an increasingly diverse and intersectional future for society.
Introduction
A website believes you to be female based on usage pattern analysis and tailors the advertisements served accordingly. A CCTV camera records footage of you traveling to work, guesses your demographics based on your face, and passes this information on to law enforcement. A well-meaning insurance company scans your application to guess your characteristics and monitor for service bias. These are just a few potential uses for AI-based demographic detection. Indeed, some are already familiar to us, with others only on the horizon. Many have already discussed the wealth of ethical, legal, and social implications around the use of this technology, so that will not be covered here. Instead, let us consider a different angle – just how sustainable is it?
I recently co-authored a report for the CDEI, where this was one question we sought to answer. One finding was that demographic trends lead toward new and otherwise unseen categories, requiring the creation and blurring of lines between demographic categories (increasing the likelihood of inaccuracies). Shifting demographic landscapes could offer diminishing accuracy returns for pre-trained models, carrying implications for end users and the wider stakeholder community, including investors. We will likely see increased investment payback time and a higher total cost of misclassifications (where such costs exist). Let us consider some examples.
Key Insights
First, imagine an algorithm that looks at someone’s name and face and infers ethnicity. If the highest-weighted element is the surname, we may expect issues. A surname that might have been identified strongly with a particular ethnic background decades ago may be less indicative today due to increased multiculturalism. For how long will our model continue to be viable? An increasing amount of people are also identifying themselves as having a ‘mixed’ ethnicity. Does our algorithm account for this? What does it think ‘mixed’ looks like, considering the wider variety of phenotypes we could expect this to take in the real world? How do we even approach handling mixed ethnicities when the implications for end use (e.g., bias monitoring) may vary depending on what backgrounds comprise each mix? People with mixed ethnic backgrounds are not a uniform monolith and should not be lumped together as such in the data. The notion of what the ‘average’ member of each ethnic background looks like will shift in any case, presenting more problems for our model.
Next, consider an algorithm that looks at a face and infers gender. When trained on historical data, this model may have learned to associate makeup as a purely female trait. However, fashion trends (particularly among younger generations) are now more open to men wearing heavier makeup, with some products advertising to men specifically. Our model will struggle to identify men wearing makeup correctly. A more impactful issue may present itself if we use the model to monitor for service bias against transgender individuals, who are a protected group in some jurisdictions. Disparate access to medical transition, among numerous other factors, means that our model will likely fail to accurately identify the demographic it is attempting to monitor bias for a significant portion of the time, defeating its deployment purpose. Now imagine this model is presented with a non-binary person, as increasing numbers of people identify as such. It does not have a category for this, nor data of the ‘average non-binary face’ to learn from—the misclassification problem compounds.
There are clear challenges with changing demographics, particularly when it comes to training models to recognize categories that blur the traditional societal expectations for categorization. In many cases, it comes down to internalized notions of identity, which cannot be easily determined by glance.
One workaround researchers have already proposed is using models that learn continuously instead of being trained once. This, however, does not help when we must create new categories where we don’t already have some data to train with. Additionally, these continuous learning models are much harder to approve when contexts are sensitive, as they open up more risks of things such as data poisoning or fluctuating accuracy. On the other hand, one could argue that ongoing learning helps prevent bias creep towards outdated data trends. This is likely to be important to image data, where we would need to consider shifting fashion trends and other cultural norms. Men in antiquity commonly wore what we might consider skirts today, though this only tends to be practiced by a much smaller group of men in Western society today. This may change again in the coming decades if red-carpet stars like Billy Porter and Harry Styles are any indication!
But will introducing new categories for new identities result in the development of new biases against those identities? This is a genuine risk, especially considering current social attitudes and extreme politics. One alternative would be to simply abandon using the technology for this purpose. This is something that has already been covered extensively by other authors, with a deeper look at the ethical question marks that demographic detection raises. Indeed, this is likely the case in some areas of the world. Under the EU AI Act, both biometric identification/categorization and facial recognition databases are considered ‘unacceptable’ risks, ruling out many of the methods we might expect to be otherwise employed.
Between the lines
I am wary of the large potential for misuse of such AI systems in the first place (despite many use cases claiming otherwise benign intentions); in the end, accepting that demographic detection is too much of a socio-legal minefield to be considered trustworthy or even worthwhile at all may be the only watertight solution. However, I am also aware that this is just one of many viewpoints. Perhaps there is an alternative way forward using coordinated industry expertise to fill the ‘governance vacuum,’ for example, by taking inspiration from drug trials and introducing a ‘pre-licensing’ phase where the acceptable (and unacceptable) use cases are agreed upon. A regulatory sandbox may also be worthwhile to trial ideas in a managed space. No matter what, it would be wise to continuously monitor and assess the performance of any solutions that make it ‘into the wild’ to ensure they perform as expected.
Only one thing remains immutable – for developers and end users, the question of sustainability in demographic detection use cases is not going away.