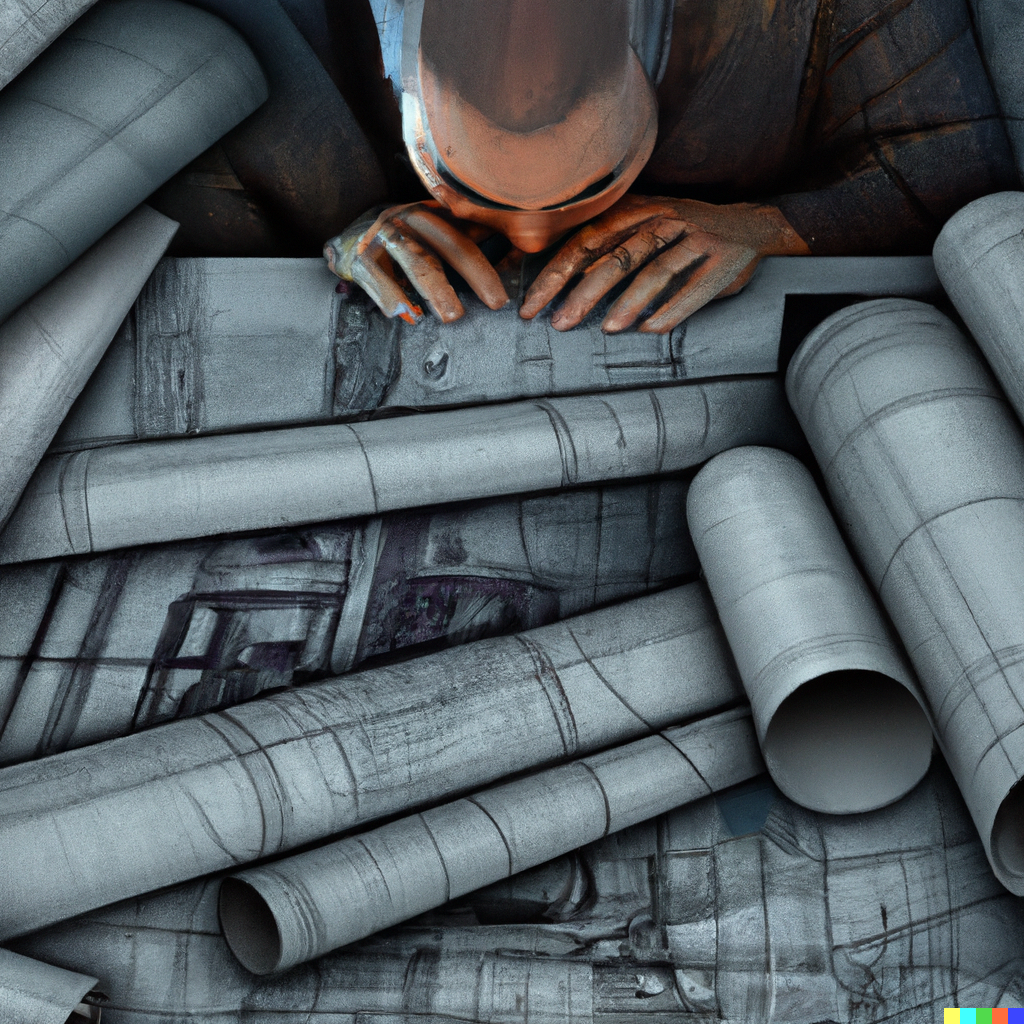
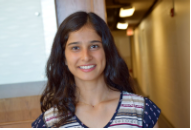
🔬 Research Summary by Mina Narayanan, a research analyst at the Center for Security and Emerging Technology working on AI Assessment, a line of research that studies how to assess AI systems and manage their risk.
[Original paper by Mina Narayanan and Christian Schoeberl]
Overview: Process frameworks for implementing responsible AI have proliferated, making it difficult to make sense of the many existing frameworks. This paper introduces a matrix that organizes responsible AI frameworks based on their content and audience. The matrix points teams within organizations building or using AI towards tools that meet their needs, but it can also help other organizations develop AI governance, policy, and procedures.
Introduction
Organizations have a growing number of frameworks at their disposal to implement responsible AI systems, or systems that minimize unwanted risks and create beneficial outcomes. One type of framework, namely process frameworks, contains actions readers can take toward achieving responsible AI. However, many process frameworks do not name a target audience or describe the audience in general terms. It can also be difficult to discern which needs are satisfied by a process framework.
To address these problems, the paper presents a new matrix that organizes more than 40 openly available frameworks to help organizations characterize them and identify which would best serve their needs. The frameworks were collected through a combination of methods, including searching through AI newsletters and tracing the in- and out-citations of several prominent AI frameworks. Unsurprisingly, many of the frameworks in the matrix focus on trustworthy AI characteristics such as privacy or explainability, likely because of their high signaling power. Organizations can easily modify the matrix by adding more frameworks or altering its dimensions. Ultimately, organizations that use the matrix will be able to more precisely apply frameworks and understand the utility of a framework relative to existing guidance.
Key Insights
Process frameworks for responsible AI provide a blueprint to ensure that organizations are prepared to meet the challenges and reap the benefits of AI systems. They contain actionable procedures for bringing an AI system into existence and/or maintaining its use. Example procedures include minimizing personal data in an AI model’s training stages or engaging with the public for comment on AI systems.
While process frameworks provide a useful shell to plug technical tools and more granular standards into, many frameworks do not specify a target audience or rely on general terms to describe the intended readers. This can make a framework seem applicable to many organizations, but in reality, it is difficult to identify who will implement the framework. Even once an audience is identified, it may still be challenging to discern how a framework measures up to other guidance.
The paper presents a matrix that contains over 40 openly available frameworks for responsible AI to alleviate these limitations. The matrix provides a structured way of thinking about who can use a process framework and the focus of the framework itself. The matrix is geared towards the user of a framework – primarily people within Development and Production teams, as well as Governance teams – residing at organizations building or using AI. To help these users select frameworks that will best serve their needs, the matrix classifies frameworks according to their respective focus areas: an AI system’s components, an AI system’s lifecycle stages, or characteristics related to an AI system.
Frameworks for Development and Production Teams
Frameworks that are suited to Development and Production teams contain processes that people closest to the development and production of AI systems perform. These individuals are usually part of technical teams and include engineers, data scientists, or product managers. Domain experts who raise technical, legal, or social considerations that engineers
may overlook are also well-equipped to implement these processes. Processes that may be found in these frameworks are “stress test an AI model using adversarial attacks” and “document the sources of data that an AI model was trained on.”
Frameworks for Governance Teams
Governance teams typically consist of people who evaluate the impact or ensure the sustainability of an organization that develops or uses AI systems. Frameworks suited to Governance teams contain processes needed to perform oversight, management, or compliance functions for AI systems or the people overseeing these systems. Processes might include “partnering with third parties to perform audits of AI systems” and “assessing the economic impact of AI systems.”
Areas of Focus: Components, Lifecycle, Characteristics
The matrix divides frameworks according to which teams could use them and by areas of focus. Three focus areas that emerged from reviewing the frameworks are Components, Lifecycle, and Characteristics. Components frameworks organize their guidance around an AI system’s components, such as data or models. Lifecycle frameworks focus on the stages of an AI system’s lifecycle, whereas Characteristics frameworks are organized around one or more characteristics, such as explainability or privacy. Frameworks in the matrix are most heavily concentrated in the Characteristics area, likely due to the ease with which organizations can espouse characteristics.
Use Cases
The following scenarios illustrate how the matrix could be used by different stakeholders to select frameworks that meet their needs:
Use Case 1: A data scientist is looking for a framework to help her responsibly document the
datasets used by a machine learning model. The data scientist can focus on the frameworks best suited for Development and Production teams since data documentation is typically completed by those closest to the development and production of the machine learning model. She is interested in frameworks that specifically address data and emphasize the design phase of the AI system lifecycle, so she may narrow her search to frameworks that belong to the Components and Lifecycle areas.
Use Case 2: A Hill staffer needs to draft provisions for a bill on AI governance, especially concerning user information privacy. The bill’s focus suggests that the staffer should locate frameworks that are suited for Governance teams and then examine which of those frameworks fall into the Characteristics area. From this subset, the staffer could pick out language reflecting the bill’s intent and report findings to policymakers.
Between the lines
The matrix demonstrates that one size does not fit all – in other words, one framework cannot meet the needs of every organization building or using AI systems. Therefore, organizations need to know what resources exist for implementing responsible AI and have a way of organizing these resources to select ones that satisfy their needs. The matrix acts as a “table of contents” for frameworks, helping organizations identify gaps existing frameworks do not fill, build their own framework, or map actions for implementing responsible AI to roles. Tools like the matrix that systematically categorize responsible AI frameworks will lay the groundwork for AI governance and support the construction of additional tools, guidance, and standards that advance AI safety.
Check out the matrix in greater detail here: https://cset.georgetown.edu/publication/a-matrix-for-selecting-responsible-ai-frameworks/